BrGAN: Blur Resist Generative Adversarial Network With Multiple Joint Dilated Residual Convolutions for Chlorophyll Color Image Restoration
IEEE Transactions on Geoscience and Remote Sensing(2023)
摘要
This article presents a blur resist generative adversarial network (BrGAN) with multiple joint dilated residual convolutions for chlorophyll image restoration of the Geostationary Ocean Color Imager (GOCI). First, a publicly available dataset was built to support this study. Second, a multiple attention perception mechanism and a multiple joint dilated residual convolution module were proposed to cope with the challenge of large missing areas in GOCI chlorophyll images. Third, a patch generative adversarial network (GAN)-based discrimination module was proposed to avoid the restored areas with generating mosaic and shadows. Our experimental results demonstrate that the BrGAN can reach 37.06 in the peak signal-to-noise ratio (PSNR) and 0.0485 in the Learned Perceptual Image Patch Similarity (LPIPS), respectively. The comparative study shows that the BrGAN achieves the highest effectiveness and advancement among other seven state-of-the-art (SOTA) methods.
更多查看译文
关键词
Convolutional neural network (CNN),Geostationary Ocean Color Imager (GOCI) ocean chlorophyll images,image inpainting,image restoration
AI 理解论文
溯源树
样例
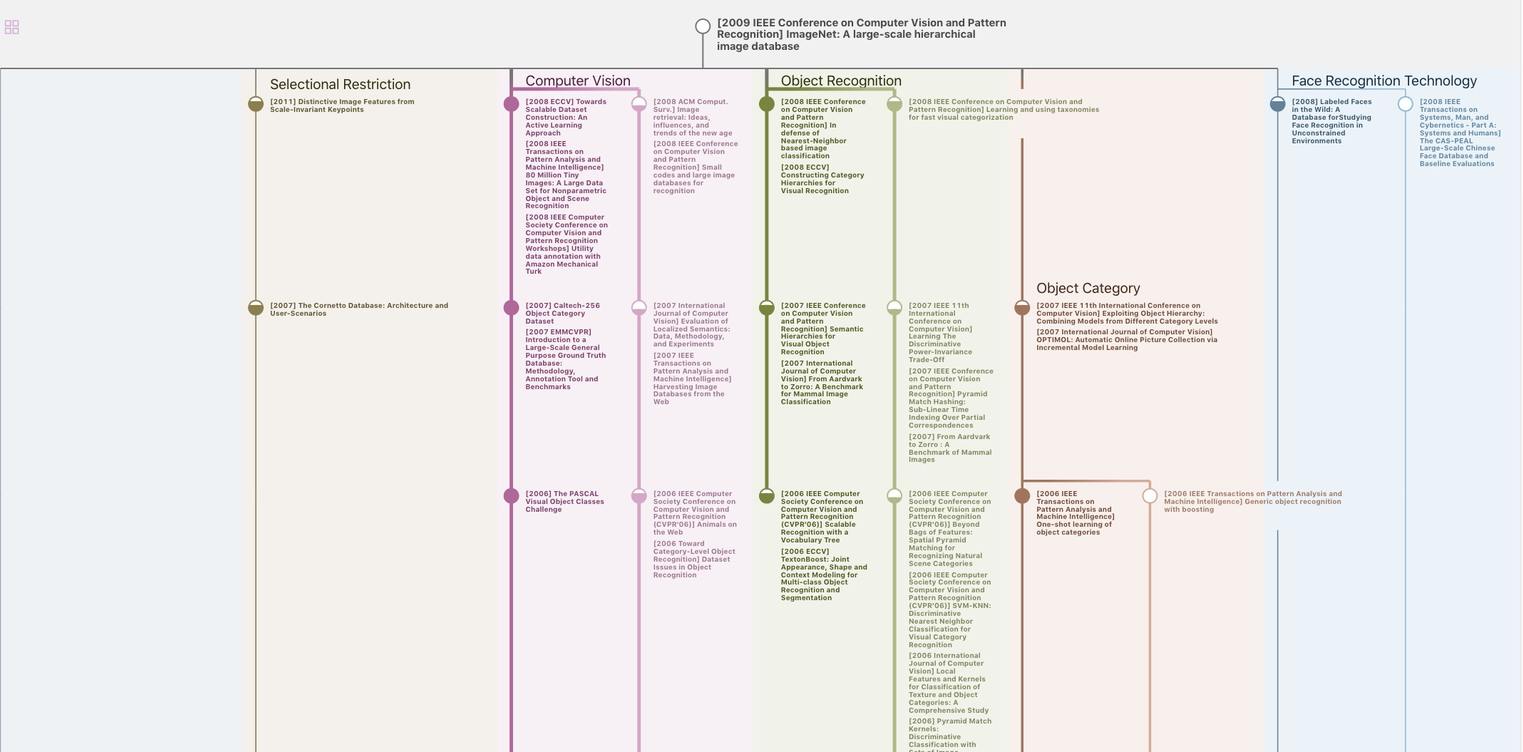
生成溯源树,研究论文发展脉络
Chat Paper
正在生成论文摘要