Layered Soil Remote Sensing With Multichannel Passive Microwave Observations Using a Physics-Embedded Artificial Intelligence Framework: A Theoretical Study
IEEE TRANSACTIONS ON GEOSCIENCE AND REMOTE SENSING(2023)
摘要
The vertical distribution of soil properties is crucial in accurately representing various hydrological and ecological processes such as freeze-thaw cycles and diurnal variations. In this article, considering the complexity of the multiparameter features of layered soil, we evaluate the potential to retrieve the vertical distribution of the moisture and temperature of soil using multichannel passive microwave observations. To enhance the inversion efficiency and accuracy, a novel physics-embedded artificial neural network (P-ANN) inversion algorithm combining multiangle (30 degrees-50 degrees), multifrequency (L-, C-, and X-bands), and multipolarization (horizontal and vertical polarization) passive observations is proposed. In this approach, the multichannel physical brightness temperature simulations corresponding to the predicted soil state parameters are integrated into the loss function of a standard fully connected feedforward neural network (NN), enabling efficient convergence with limited sampling data in the training process. Testing results exhibit that the inversion performance of P-ANN is superior to that of conventional NN approaches, which only adopts errors in soil states in the loss function to train the network. The test also shows that the proposed P-ANN approach outperforms traditional optimization algorithms in dealing with layered soil retrieval. In order to further improve the retrieval accuracy, an advanced local optimization scheme is also proposed, where the output from P-ANN is further treated as the initial value to a local optimization algorithm, achieving even closer results to the ground truths without excessive computational costs. In addition, to estimate the reliability of the model predictions, this article also establishes, in the testing process, a statistical relationship between the soil inversion error and the error of the corresponding brightness temperatures. When the trained NN is in operation, the error of brightness temperature is calculated through the physical model, and the reliability of retrieval soil results is then acquired by putting the calculated brightness temperature errors into the preestablished statistical relationship. The proposed concepts and approaches have demonstrated the feasibility of using P-ANN model with multidimensional observations to invert the multivariable layered soil structures. The proposed approach holds great potential for various remote sensing applications as well as solving a wide range of inverse problem challenges.
更多查看译文
关键词
Soil,Artificial neural networks,Neural networks,Optimization,Brightness temperature,Reliability,Training,Layered soil remote sensing,optimization algorithm,physics-driven artificial neural network (ANN),reliability
AI 理解论文
溯源树
样例
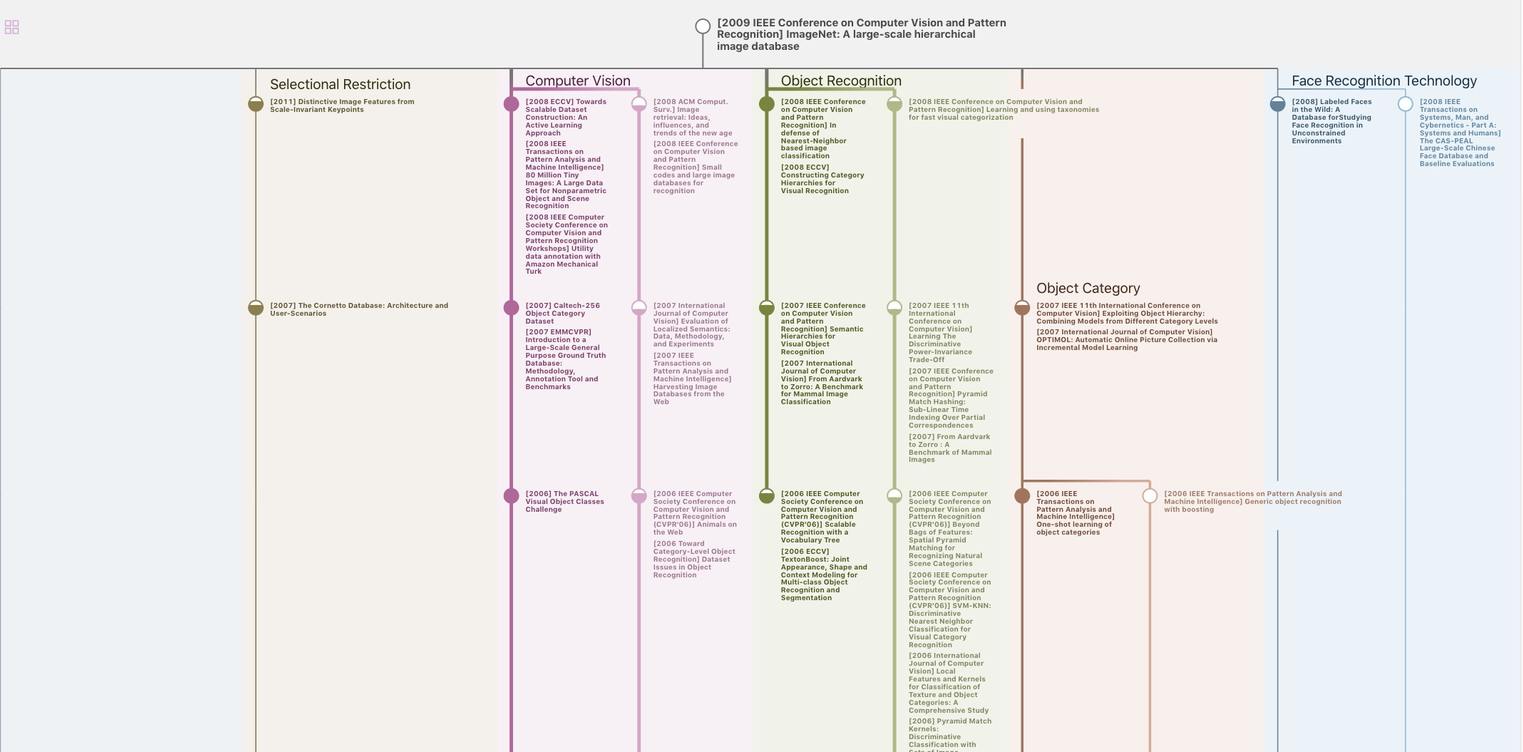
生成溯源树,研究论文发展脉络
Chat Paper
正在生成论文摘要