GeoFormer: A Geometric Representation Transformer for Change Detection
IEEE TRANSACTIONS ON GEOSCIENCE AND REMOTE SENSING(2023)
摘要
Deep representation learning has improved automatic remote sensing change detection (RSCD) in recent years. Existing methods emphasize primarily convolutional neural networks (CNNs) or transformer-based networks. However, most of them neither effectively combine CNNs and transformers nor use prior geometric information to refine regions. In this article, a novel geometric representation transformer (GeoFormer) is proposed for high-resolution RSCD. GeoFormer utilizes convolutional information to guide the transformer by employing geometric prior knowledge. Specifically, the proposed GeoFormer consists of three carefully designed components: the geometric-based Swin transformer (Geo-Swin Transformer) encoder, the Laplace attention fusion (LAFusion) module, and the UNet++CD decoder. First, the Geo-Swin Transformer is a novel-designed nonlocal Siamese encoder that combines geometric convolution with a transformer to provide local geometric representation information for remote contextual features. Then, an LAFusion module is proposed to achieve robust bi-temporal feature fusion, which is founded on attention mechanism and edge information. Finally, UNet++CD decodes fine-grained information from the fused features by a dense multiscale upsampling process. Experimental results demonstrate that the proposed GeoFormer performs better than benchmark methods on four change detection datasets (LEVIR-CD, WHU-CD, DSIFN-CD, and CDD) and is able to detect the edges of change regions more precisely.
更多查看译文
关键词
Attention mechanism,change detection,edge information,transformer
AI 理解论文
溯源树
样例
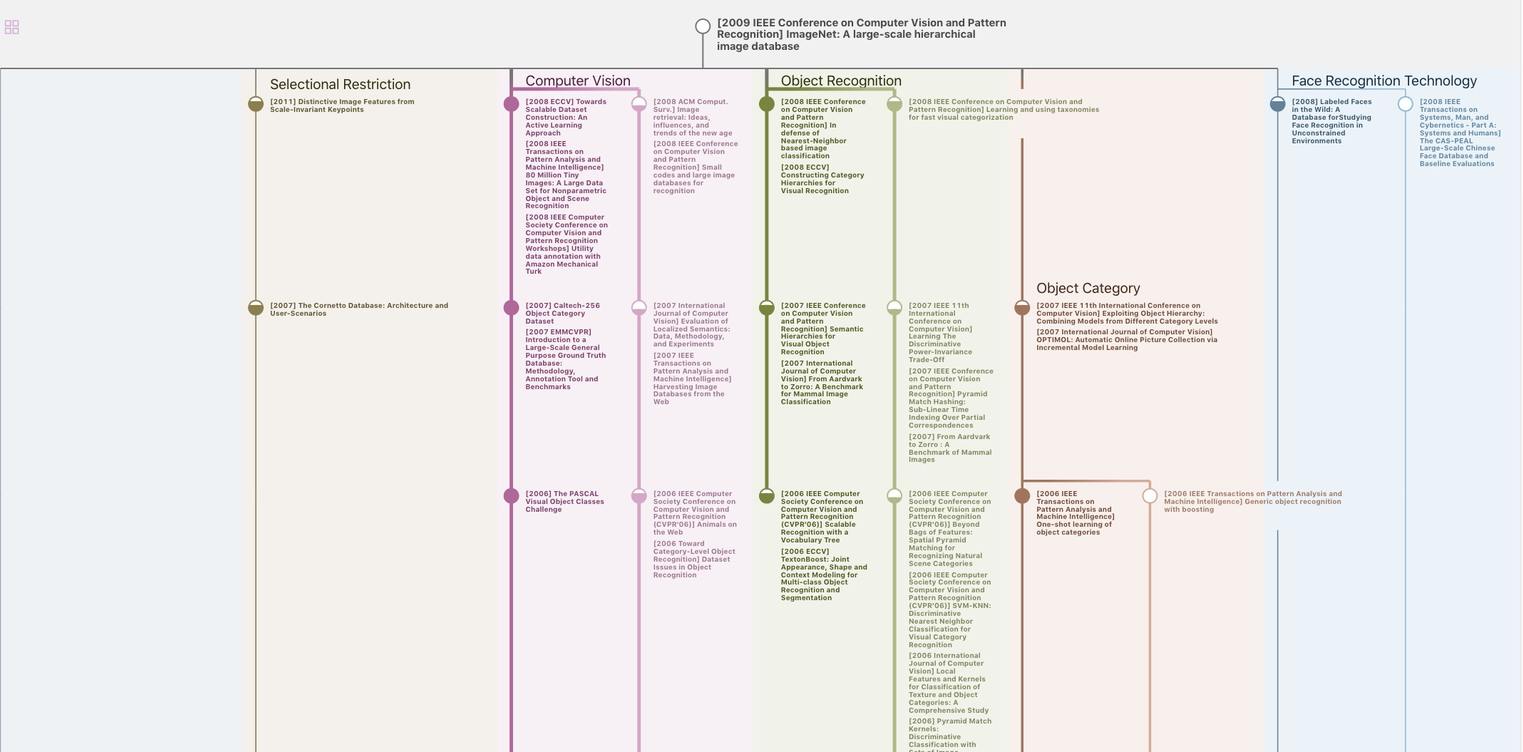
生成溯源树,研究论文发展脉络
Chat Paper
正在生成论文摘要