Few-Shot Remote Sensing Scene Classification With Spatial Affinity Attention and Class Surrogate-Based Supervised Contrastive Learning
IEEE Transactions on Geoscience and Remote Sensing(2023)
摘要
Learning powerful and discriminative representation is critical for boosting the performance of few-shot remote sensing scene classification (FSRSSC). The remote sensing images have unique characteristics, such as, complex background and co-occurrence of multiple objects, making FSRSSC challenging. To address this problem, in this article, a novel FSRSSC method is proposed. First, a spatial affinity attention (SAA) mechanism is designed to encourage the network model to focus on critical regions. The SAA infers attention maps from both channel and spatial dimensions, and encodes the mean values and affinities of feature nodes in each channel along the vertical and horizontal directions. Second, a class surrogate-based supervised contrastive learning (CSSCL) strategy is proposed to promote intra-class compactness and inter-class dispersion. Different from supervised contrastive learning (SCL), the CSSCL learns a surrogate for each class in the contrastive space and select the corresponding class surrogate rather than the samples from the same class for the anchor to form positive pairs. It can alleviate the impact of too hard or too simple positive sample pairs on the model generalization that exists when SCL is introduced into FSRSSC. The model is trained under the joint supervision of cross-entropy (CE) loss and CSSCL loss on the merged base class data instead of using a meta-learning strategy to learn a robust base feature extractor. Extensive experimentations on three public remote sensing benchmark datasets show that our proposed method can achieve a competitive or state-of-the-art (SOTA) classification performance.
更多查看译文
关键词
Class surrogate-based supervised contrastive learning (CSSCL),few-shot leaning,remote sensing image scene classification,spatial affinity attention (SAA)
AI 理解论文
溯源树
样例
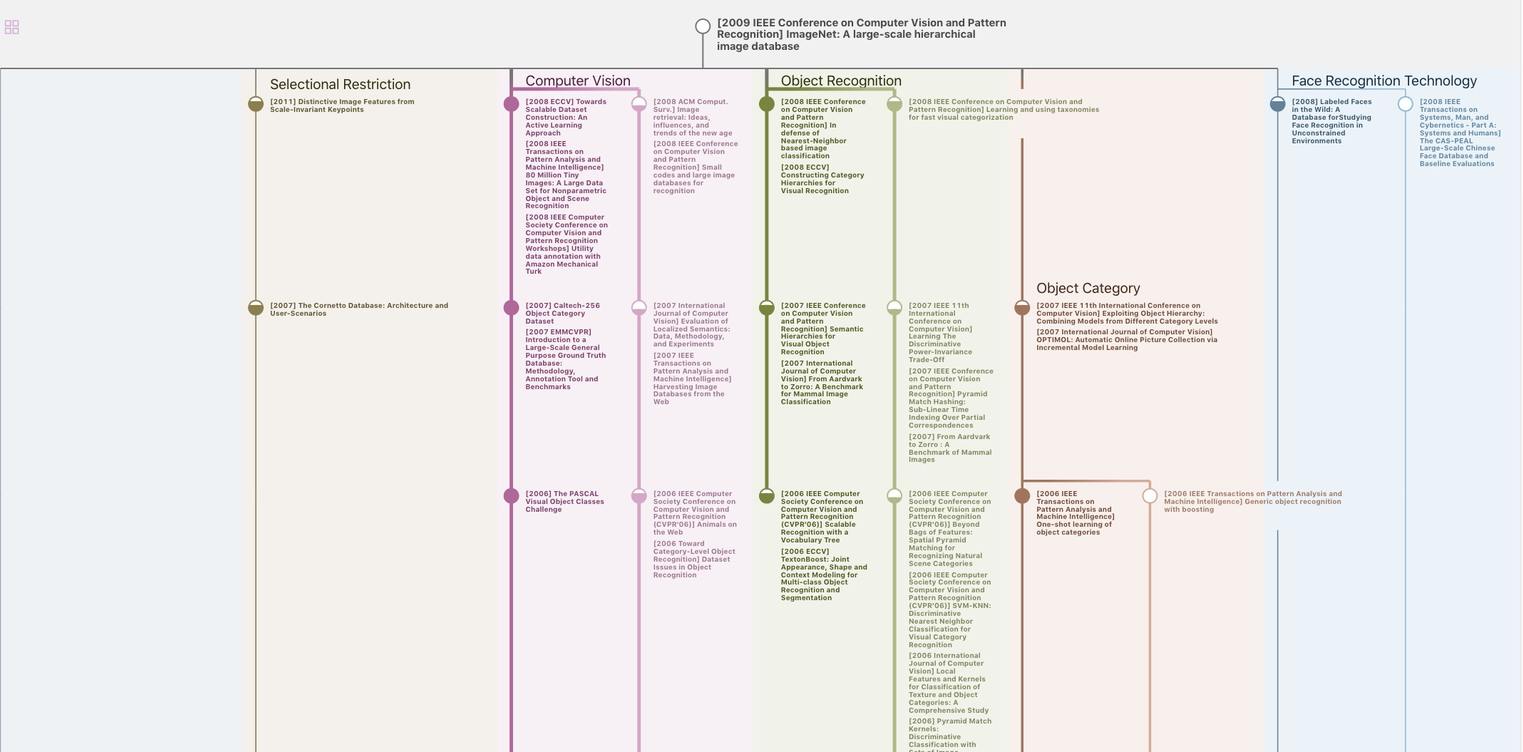
生成溯源树,研究论文发展脉络
Chat Paper
正在生成论文摘要