Robust Correlation Filter Learning With Continuously Weighted Dynamic Response for UAV Visual Tracking
IEEE Transactions on Geoscience and Remote Sensing(2023)
摘要
Unmanned aerial vehicles (UAVs) visual tracking has always been a challenging task. Existing correlation filter tracking algorithms typically utilize the histograms of oriented gradients (HOGs) and color names (CNs) method to directly incorporate the extracted target features into the model updating process. However, low-resolution (LR) video quality leads to unstable target feature values. To address this limitation, we propose a novel preprocessing technique involving Gaussian denoising. This preprocessing step is designed to enhance the stability of the target’s feature values and make the target’s scale information clearer, thereby improving the tracker’s recognition capability for the target and effectively reducing noise interference. Furthermore, in contrast to other UAV trackers that rely on a singular representation of contextual information, this article aims to enhance the utilization of historical information. Therefore, we introduce a context-based approach that integrates continuously weighted dynamic response maps from both temporal and spatial perspectives. Our tracker has the ability to adapt to rapid environmental changes during the tracking process while simultaneously reducing the potential risks of model overfitting and distortion. Extensive experiments are conducted on authoritative datasets, including DTB70, UAV123@10fps, and UAVDT, comparing our model against other advanced trackers. The experimental results validate the superior tracking performance and robustness of our tracker.
更多查看译文
关键词
robust correlation filter learning,uav,tracking,continuously weighted
AI 理解论文
溯源树
样例
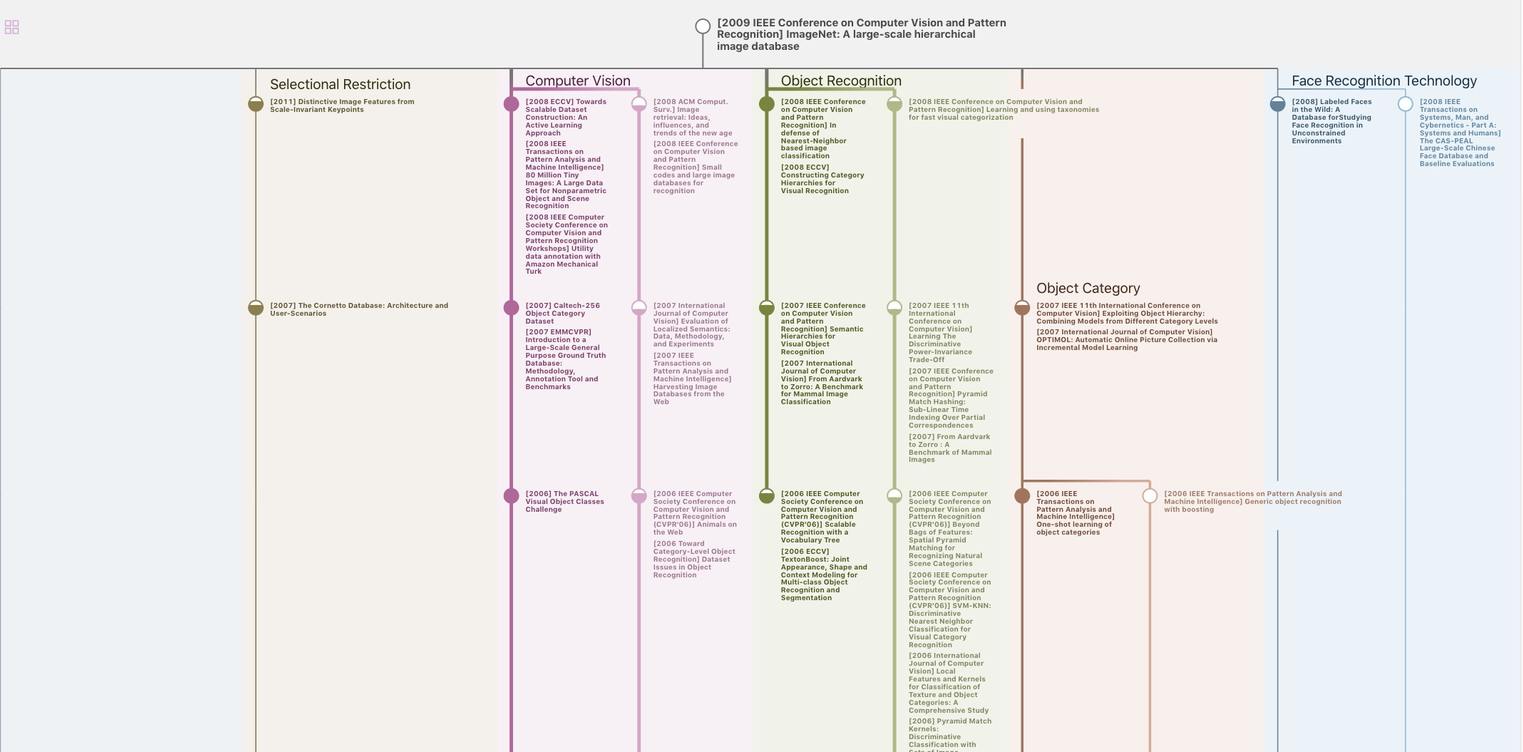
生成溯源树,研究论文发展脉络
Chat Paper
正在生成论文摘要