MANet: An Efficient Multidimensional Attention-Aggregated Network for Remote Sensing Image Change Detection
IEEE TRANSACTIONS ON GEOSCIENCE AND REMOTE SENSING(2023)
摘要
Deep learning has significantly advanced the change detection (CD) in remote sensing (RS) image with its excellent performance. For CD tasks, there are two critical issues. First, with scale variance of different objects in RS images, effectively aggregating multiscale features helps to generate fine-grained change objects. Second, it is critical but challenging to fully exploit the variance information between bi-temporal images to avoid pseudo-variation and region blurring. To alleviate the above issues, this article proposes an efficient multidimensional attention-aggregation network (MANet), which keeps better feature aggregation while maintaining excellent differential attention ability. This article carries three main contributions. First, we propose a multiscale asymmetric convolutional attention (MACA) module. Due to the asymmetric convolution's ability to focus on feature contours effectively, the MACA can not only aggregate multiscale features effectively, but also refine the edge information of features. Second, we propose a dual-dimensional attention (DDA) module for adaptively fusing shallow and deep features, which is used to generate rich feature representations. Third, the difference guidance (DG) module is exploited for enhancing the attention of changed regions to mitigate the influence of uncorrelated changes on the CD result. Experiments on four popular CD datasets show that our network can accomplish higher detection accuracy than the state-of-the-art networks.
更多查看译文
关键词
Change detection (CD),difference guidance (DG) module,dual-dimensional attention (DDA) module,multiscale asymmetric convolutional attention (MACA) module,remote sensing (RS)
AI 理解论文
溯源树
样例
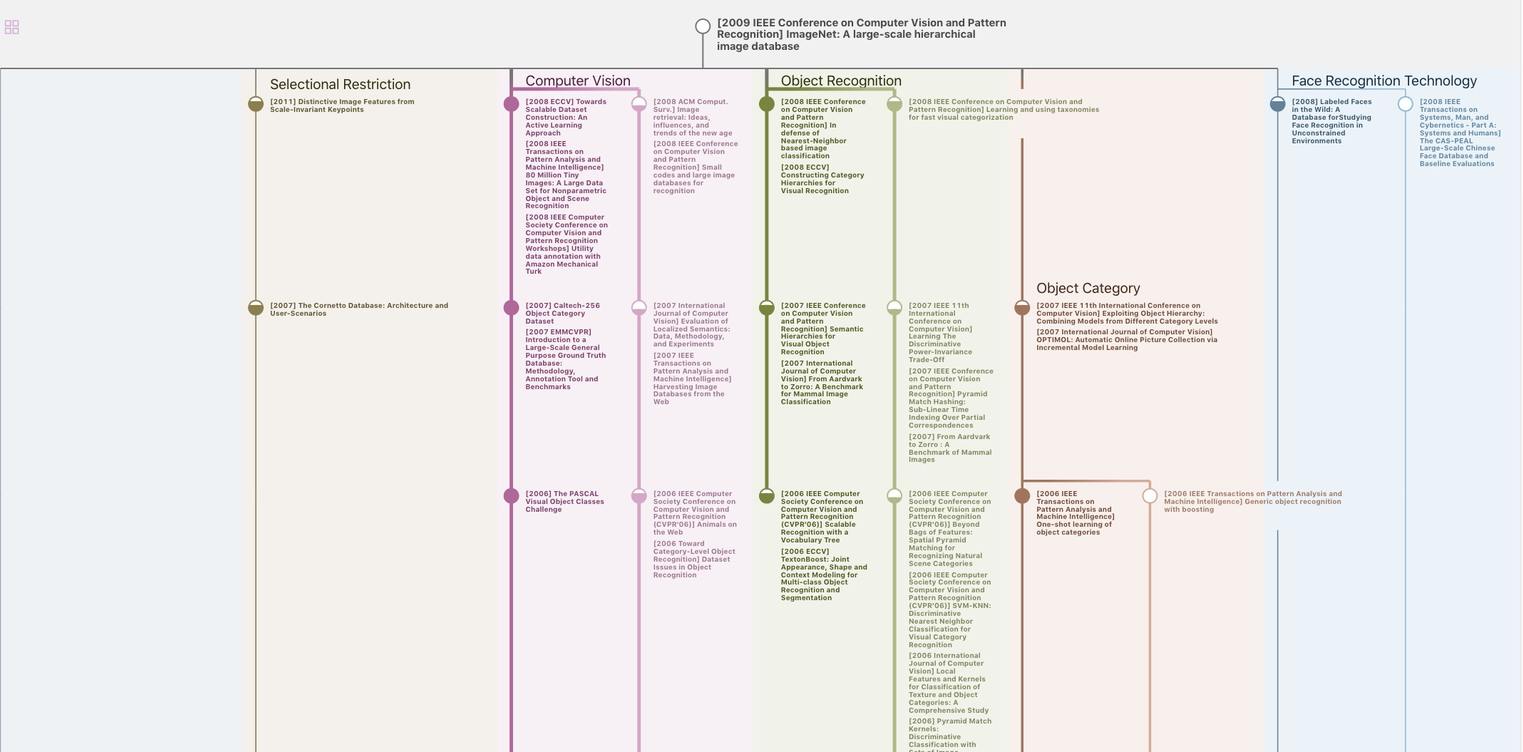
生成溯源树,研究论文发展脉络
Chat Paper
正在生成论文摘要