MDFS-Net: Multidomain Few Shot Classification for Hyperspectral Images With Support Set Reconstruction
IEEE TRANSACTIONS ON GEOSCIENCE AND REMOTE SENSING(2023)
摘要
Deep neural networks are highly specialized for a given task and visual domain, which can limit their practical use. To address this issue, recent studies have proposed to learn universal feature extractors that can be used across multiple domains simultaneously, inspired by the success of transfer learning (TL). However, these universal features are still inferior to specialized networks. In the context of hyperspectral image (HSI) classification, the lack of labeled training samples due to high cost and the restriction to single-domain learning further complicates the problem. To overcome these challenges, we propose a solution that combines the problems of multidomain learning (MDL) and few-shot learning (FSL) for HSI classification. Our goal is to train a highly shareable network with all domains in the low-shot training regime. We call our network the multidomain few-shot network (MDFS-Net), which shares the majority of model parameters (specifically, convolution and dense layer parameters) across domains while keeping domain-specific batch-normalization layers separate to capture domain characteristics. To address the overfitting issue in few-shot models, we supplement the main classification task with an auxiliary self-supervised task. We test our proposed method on five benchmark HSI datasets and find that MDFS-Net consistently outperforms relevant baselines convincingly. Our approach offers a promising solution for HSI classification in remote sensing (RS) by enabling the design of a unified classification system that can work with multiple HSI sites (domains) and fewer labeled samples.
更多查看译文
关键词
3-D CNNs,few-shot learning (FSL),hyperspectral image (HSI) classification,multidomain learning (MDL),remote sensing (RS)
AI 理解论文
溯源树
样例
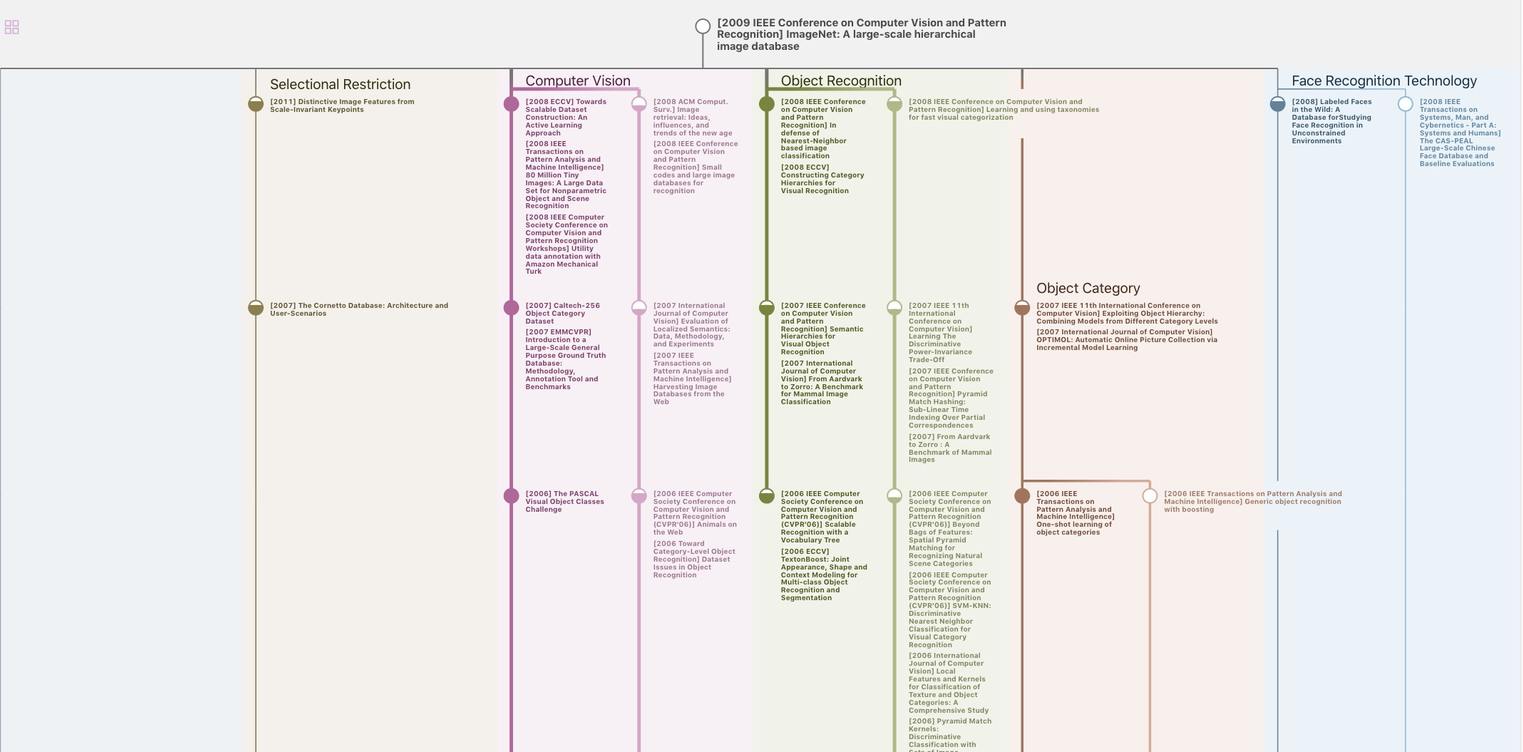
生成溯源树,研究论文发展脉络
Chat Paper
正在生成论文摘要