PointGame: Geometrically and Adaptively Masked Autoencoder on Point Clouds
IEEE TRANSACTIONS ON GEOSCIENCE AND REMOTE SENSING(2023)
摘要
Self-supervised learning is attracting large attention in point cloud understanding. However, exploring discriminative and transferable features still remains challenging due to their nature of irregularity. We propose a geometrically and adaptively masked autoencoder on point clouds for self-supervised learning, termed PointGame. PointGame contains two core components: GATE and EAT. GATE stands for the geometrical and adaptive token embedding module; it not only absorbs the conventional wisdom of geometric descriptors that capture the surface shape effectively, but also exploits adaptive saliency to focus on the salient part of a point cloud. EAT stands for the external attention-based transformer encoder with linear computational complexity, which increases the efficiency of the whole pipeline. Unlike cutting-edge unsupervised learning models, PointGame leverages geometric descriptors to perceive surface shapes and adaptively mines discriminative features from training data. PointGame showcases clear advantages over its competitors on various downstream tasks under both global and local fine-tuning strategies. The code and pretrained models will be publicly available.
更多查看译文
关键词
Point cloud compression,Feature extraction,Three-dimensional displays,Task analysis,Transformers,Representation learning,Shape,Geometric descriptors,geometrical and adaptive token embedding (GATE),masked autoencoder,representation learning,self-supervised learning
AI 理解论文
溯源树
样例
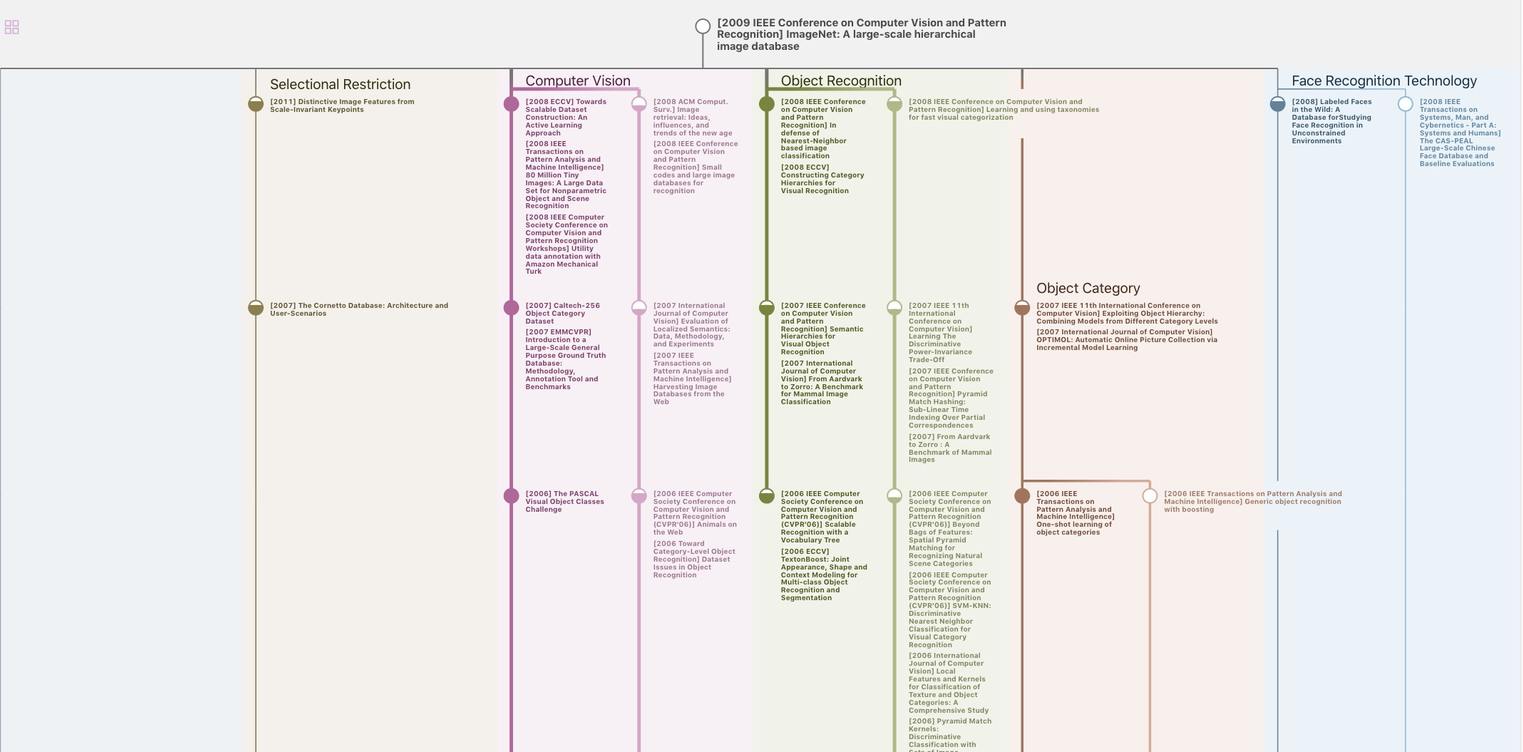
生成溯源树,研究论文发展脉络
Chat Paper
正在生成论文摘要