Unsupervised Deep Learning for Ground Roll and Scattered Noise Attenuation
IEEE Transactions on Geoscience and Remote Sensing(2023)
摘要
The attenuation of coherent noise in land seismic data, specifically ground roll and near-surface scattered energy, remains a longstanding challenge. Although recent advances in deep learning have improved signal separation from coherent noise, supervised methods are limited by the necessity for realistic training samples. To circumvent this issue, we propose an unsupervised deep learning approach to attenuate ground roll and scattered energy, eliminating the requirement for training labels. Our method leverages the inherent low-frequency bias of a generator network, which is naturally prone to learn self-similar features during training. This empowers the network to extract the desired component exhibiting self-similarity in the time-space domain, while disregarding unwanted components. Notably, horizontal components in seismic data exhibit pronounced self-similarity. To enhance the self-similarity of ground roll, we apply a linear moveout (LMO) correction to horizontally align it and utilize the generator network for separation. Additionally, for scattered energy attenuation, we employ the generator network to extract flattened reflections after normal moveout (NMO) correction. Our strategy distinctively merges model-driven procedures, specifically NMO and LMO, anchored in the geological velocity model. The synergy between data-driven deep learning and model-driven processes underscores the success of our approach. We demonstrate the validity of our proposed method using both synthetic and field shot data. The field data examples highlight the superior attenuation capabilities of our method, surpassing conventional denoising techniques by effectively reducing both random and coherent noise.
更多查看译文
关键词
unsupervised deep learning,noise attenuation,deep learning,ground roll
AI 理解论文
溯源树
样例
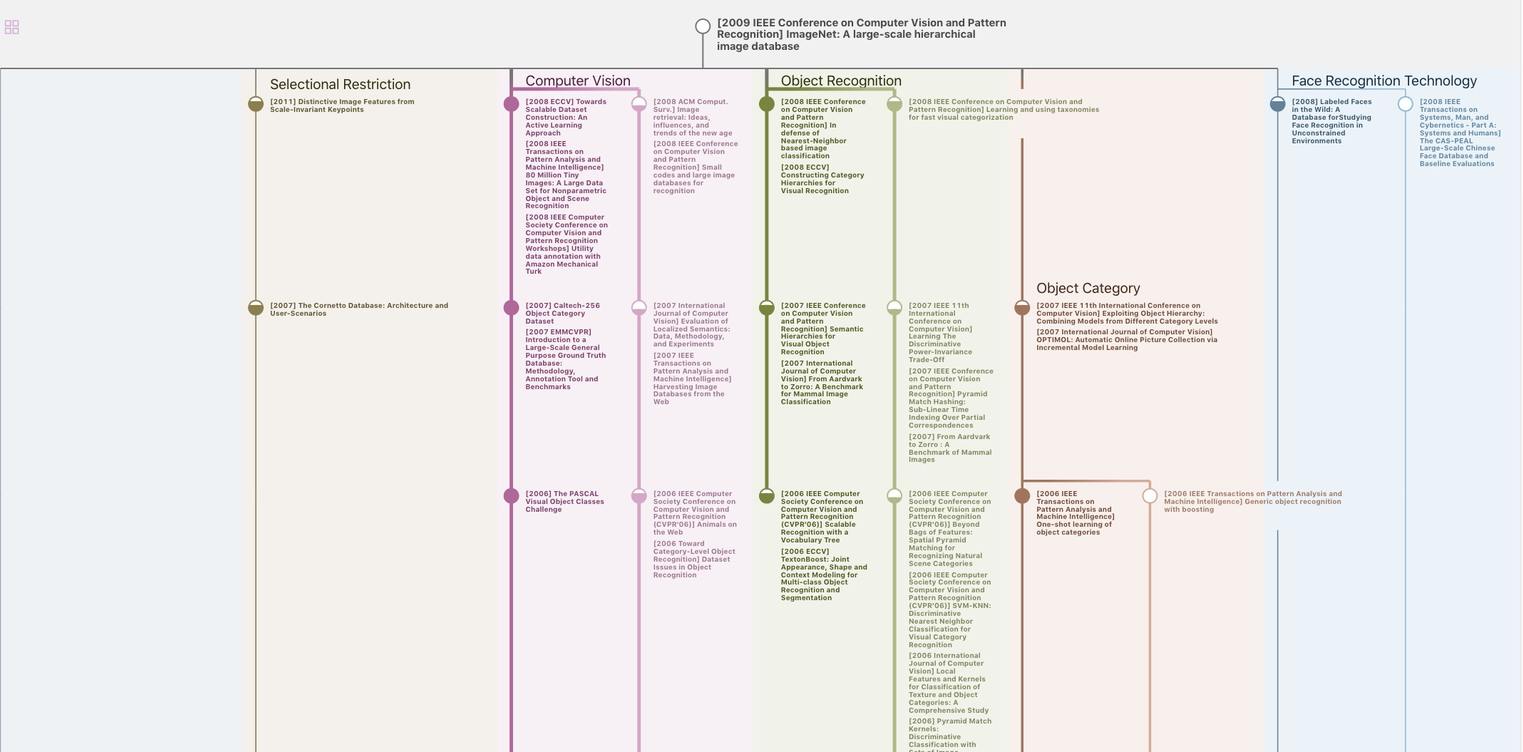
生成溯源树,研究论文发展脉络
Chat Paper
正在生成论文摘要