Sequence-to-Sequence-Based Beta-VAE Combined With IECA Attention Mechanism for Energy Disaggregation Algorithm
IEEE TRANSACTIONS ON INSTRUMENTATION AND MEASUREMENT(2023)
摘要
Nonintrusive load monitoring (NILM) is a technology that measures and identifies individual appliances' energy consumption by analyzing the total power consumption collected by building sensors. Using the energy disaggregation method, the total power consumption can be disaggregated down to the device level. With the emergence of deep learning, energy disaggregation methods have improved in recent years. However, NILM still faces significant challenges in its generalization capabilities for different households and in disaggregating complex multistate devices. To address these issues, on the basis of sequence-to-sequence, this article proposes a beta-variational autoencoder (Beta-VAE) combined with improved efficient channel attention (IECA) mechanism for energy disaggregation algorithm. In this model, Beta-VAE leverages its strong feature learning and disentanglement capabilities to improve the accuracy of multistate device disaggregation by learning the feature representation of the latent space. Meanwhile, a new improved channel attention IECA is also introduced, which is combined with skip connection technology and is applied between the encoder and decoder to capture multiscale features and improve the reconstruction of power signals. Furthermore, we apply the Gaussian error linear unit (GELU) activation function and instance normalization to the feature extraction network to improve the convergence speed and generalization capability of the model. Finally, we tested and compared our method with several state-of-the-art NILM algorithms on the U.K.-DALE and REFIT datasets and obtain significant improvement. The results showed that the mean absolute error (MAE) of all appliances decreased by an average of 46% and the F1 Score increased by 20%.
更多查看译文
关键词
Beta-variational autoencoder (Beta-VAE),energy disaggregation,Gaussian error linear unit (GELU),improved efficient channel attention (IECA) attention mechanism,instance normalization,nonintrusive load monitoring (NILM)
AI 理解论文
溯源树
样例
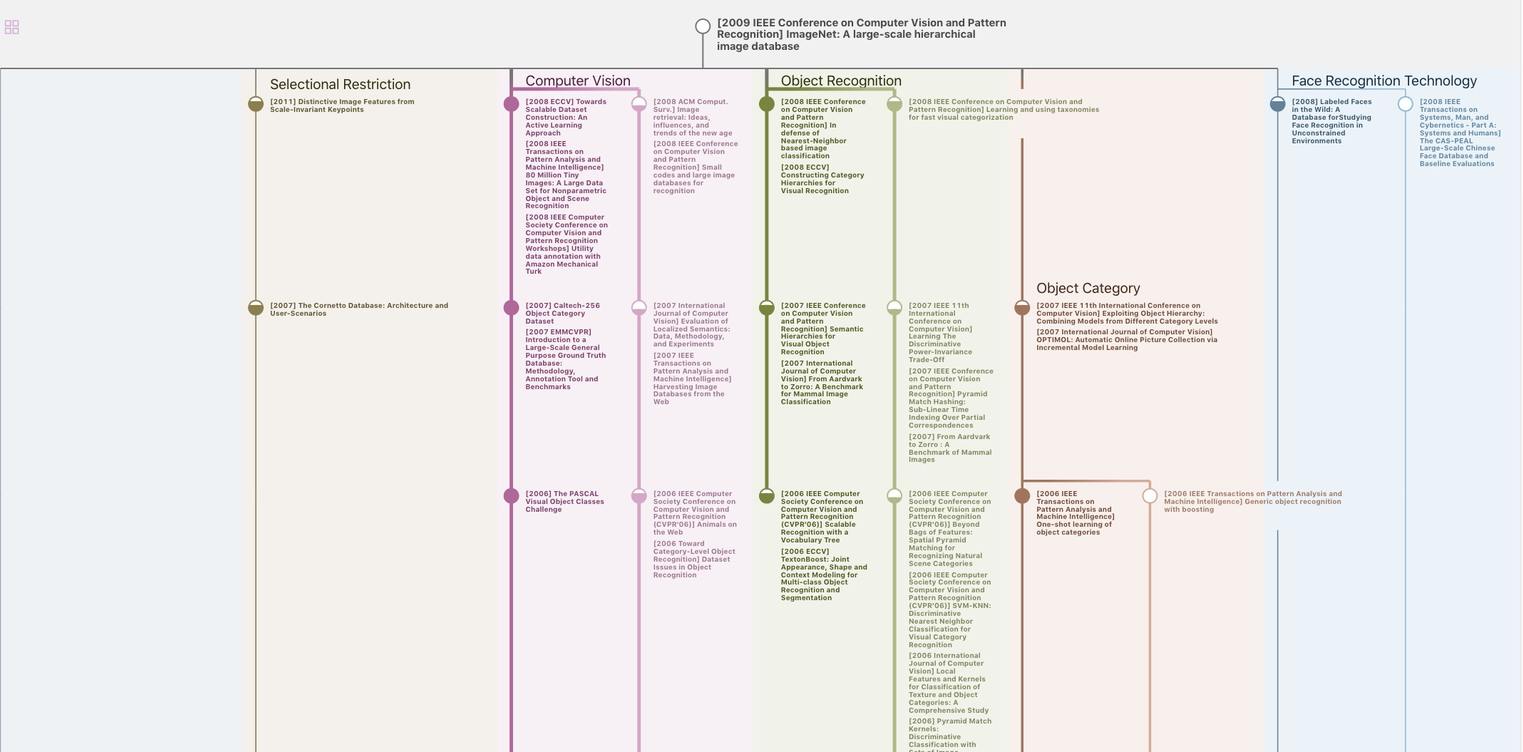
生成溯源树,研究论文发展脉络
Chat Paper
正在生成论文摘要