Learning Meta-Stable State Transition Representation of Brain Function for ADHD Identification
IEEE TRANSACTIONS ON INSTRUMENTATION AND MEASUREMENT(2023)
摘要
Attention deficit hyperactivity disorder (ADHD) is a common neurodevelopmental disorder in children with complex onset symptoms, and brain function analysis is a helpful way to assist in diagnosis. Functional magnetic resonance imaging (fMRI) studies have revealed that the brain function activity pattern in the resting state is also dynamically changing, that is, there is a meta-stable state transition within the period of acquisition time, which is related to neurological diseases. However, existing studies neglect to mine such state transition pattern information for better disease identification. Here, we propose an end-to-end method that learns meta-stable state transition representation for ADHD identification. First, the fMRI data acquired during the entire period are divided into overlapping segments based on the sliding window operation, and each segment is used to capture the meta-stable state of brain function. Then, for each meta-stable state, a 2-D convolutional neural network (CNN) is adopted to extract functional connectivity (FC) information from FC network (FCN) constructed by fMRI volumes, while a 3-D CNN is used to extract spatial information from fMRI volumes and a bidirectional long short-term memory (Bi-LSTM) is used to extract temporal information from the volume sequence, and the FC feature and spatiotemporal feature are fused to represent the meta-stable state. Finally, a Bi-LSTM with attention mechanism is conducted to learn the state transition pattern from the representations of the meta-stable states, and the state transition feature is used for classification. The results on the ADHD-200 dataset indicate that: 1) the meta-stable transition pattern is indeed useful and can improve identification performance; 2) fusing the static FC feature and dynamic volume sequence feature of brain function can better characterize meta-stable states; and 3) the combination of window size = 50 and step size = 40 is an ideal choice in the sliding window operation, which can obtain the best performance.
更多查看译文
关键词
Functional connectivity network (FCN),functional magnetic resonance imaging (fMRI),sliding window,state transition,volume sequence
AI 理解论文
溯源树
样例
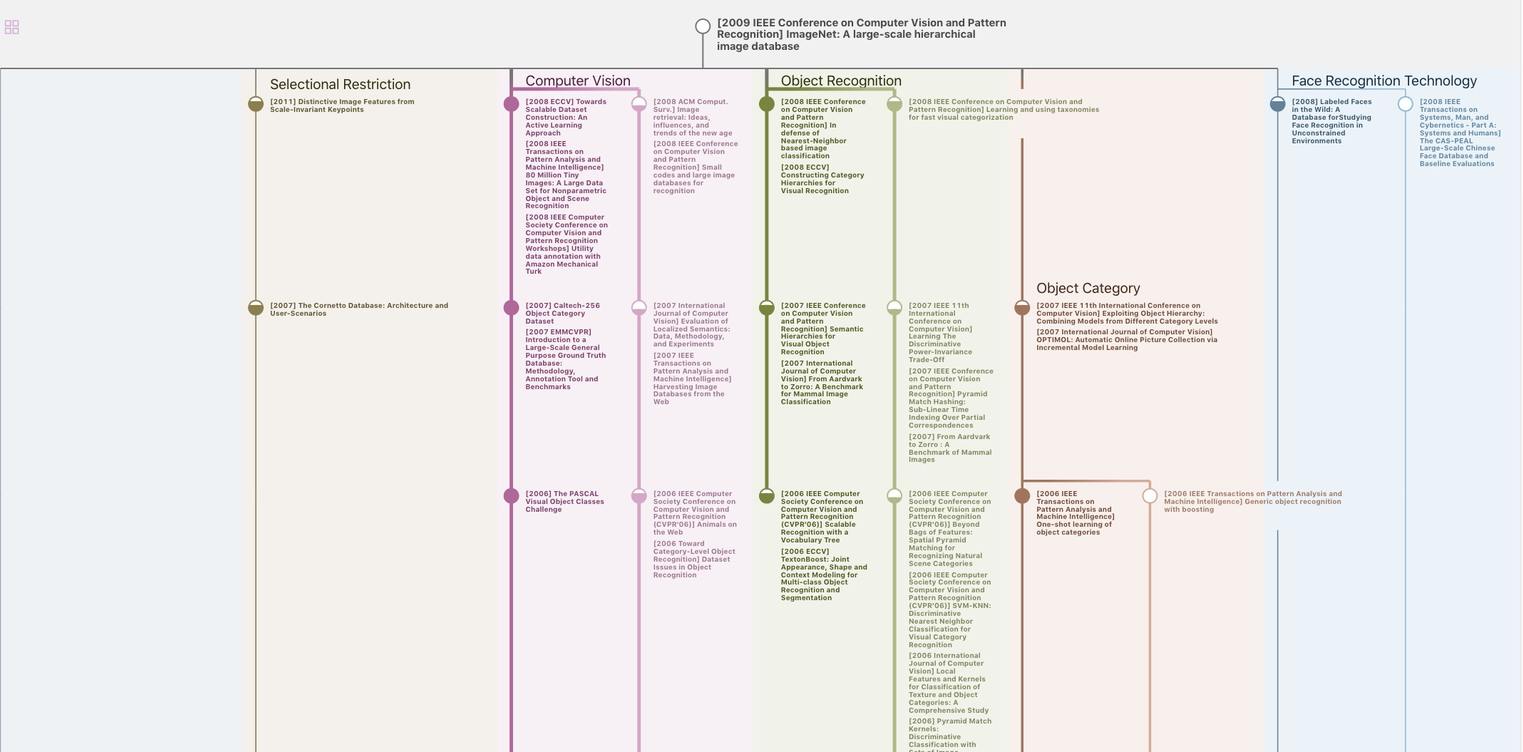
生成溯源树,研究论文发展脉络
Chat Paper
正在生成论文摘要