DePF: A Novel Fusion Approach Based on Decomposition Pooling for Infrared and Visible Images
IEEE TRANSACTIONS ON INSTRUMENTATION AND MEASUREMENT(2023)
摘要
Infrared and visible image fusion is a crucial technique in the field of computer vision, aiming to create synthetic images that simultaneously capture salient features and rich texture details. These fused images play a pivotal role in enhancing various downstream tasks. However, existing fusion methods often encounter challenges such as texture loss and deficiencies in edge information, resulting in less than optimal fusion outcomes. Additionally, straightforward up-sampling techniques struggle to preserve source information from multiscale features. To tackle these issues, a novel fusion network based on the decomposition pooling (de-pooling) manner is proposed, termed as Decomposition Pooling-based Fusion (DePF). DePF features a de-pooling-based encoder designed to extract multiscale image and detail features from source images concurrently. Furthermore, a spatial attention model aggregates these salient features, and the decoder employs a de-pooling reversed operation instead of the typical up-sampling operator to reconstruct the fused features. Unlike conventional max-pooling techniques, the de-pooling layer preserves abundant detail information, facilitating a richer texture and multiscale information retention during the reconstruction phase. Significantly, our approach exhibits remarkable efficiency, requiring merely 23 ms to integrate a pair of infrared and visible images, each with dimensions of 640 x 480. Furthermore, empirical findings corroborate the exceptional fusion efficacy of our methodology in the domains of object detection and noise-related assessments, surpassing the performance of contemporary techniques within numerous image fusion benchmarks.
更多查看译文
关键词
Feature extraction,Image fusion,Task analysis,Decoding,Image reconstruction,Data mining,Generative adversarial networks,Decomposition pooling (de-pooling),deep learning,detail features,image fusion,multiscale features
AI 理解论文
溯源树
样例
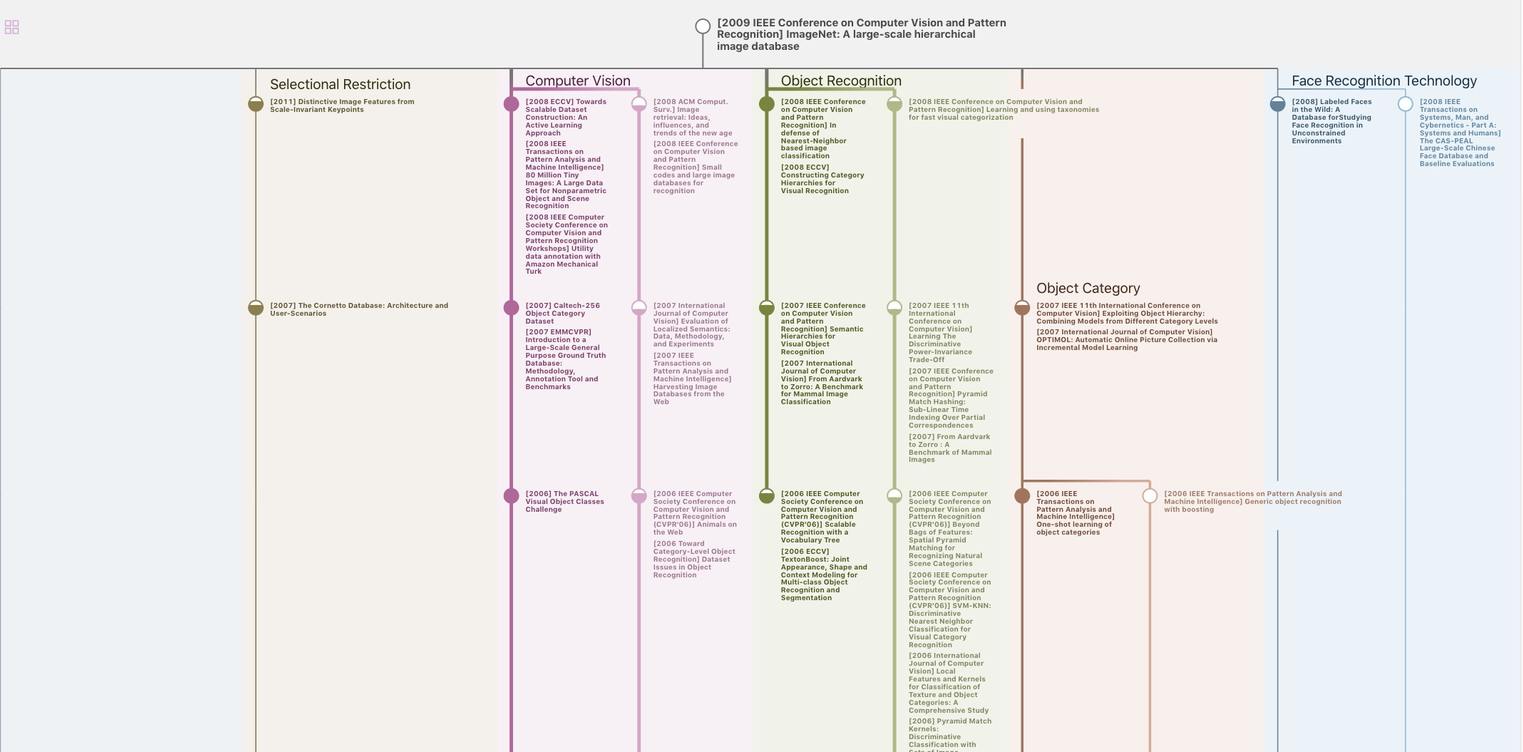
生成溯源树,研究论文发展脉络
Chat Paper
正在生成论文摘要