Global Representation Guided Adaptive Fusion Network for Stable Video Crowd Counting
IEEE Transactions on Multimedia(2023)
摘要
Modern crowd counting methods in natural scenes, even when video datasets are available, are mostly based on images. Because of background interference or occlusion in the scene, these methods can easily lead to mutations and instability in density prediction. There has been minimal research on how to exploit the inherent consistency among adjacent frames to achieve high estimation accuracy of video sequences. In this study, we explore the long-term global temporal consistency in the video sequence and propose a novel Global Representation Guided Adaptive Fusion Network (GRGAF) for video crowd counting. The primary aim is to establish a long-term temporal representation among consecutive frames to guide the density estimation of local frames, which can alleviate the prediction instability caused by background noise and occlusions in crowd scenes. Moreover, in order to further enforce the temporal consistency, we apply the generative adversarial learning scheme and design a global-local joint loss, which can make the estimated density maps more temporally coherent. Extensive experiments on four challenging video-based crowd counting datasets (FDST, DroneCrowd, MALL and UCSD) demonstrate that our method makes effective use of spatio-temporal information of video and outperforms the other state-of-the-art approach.
更多查看译文
关键词
Adaptive fusion, crowd counting, global temporal representation, spatio-temporal consistency, video understanding
AI 理解论文
溯源树
样例
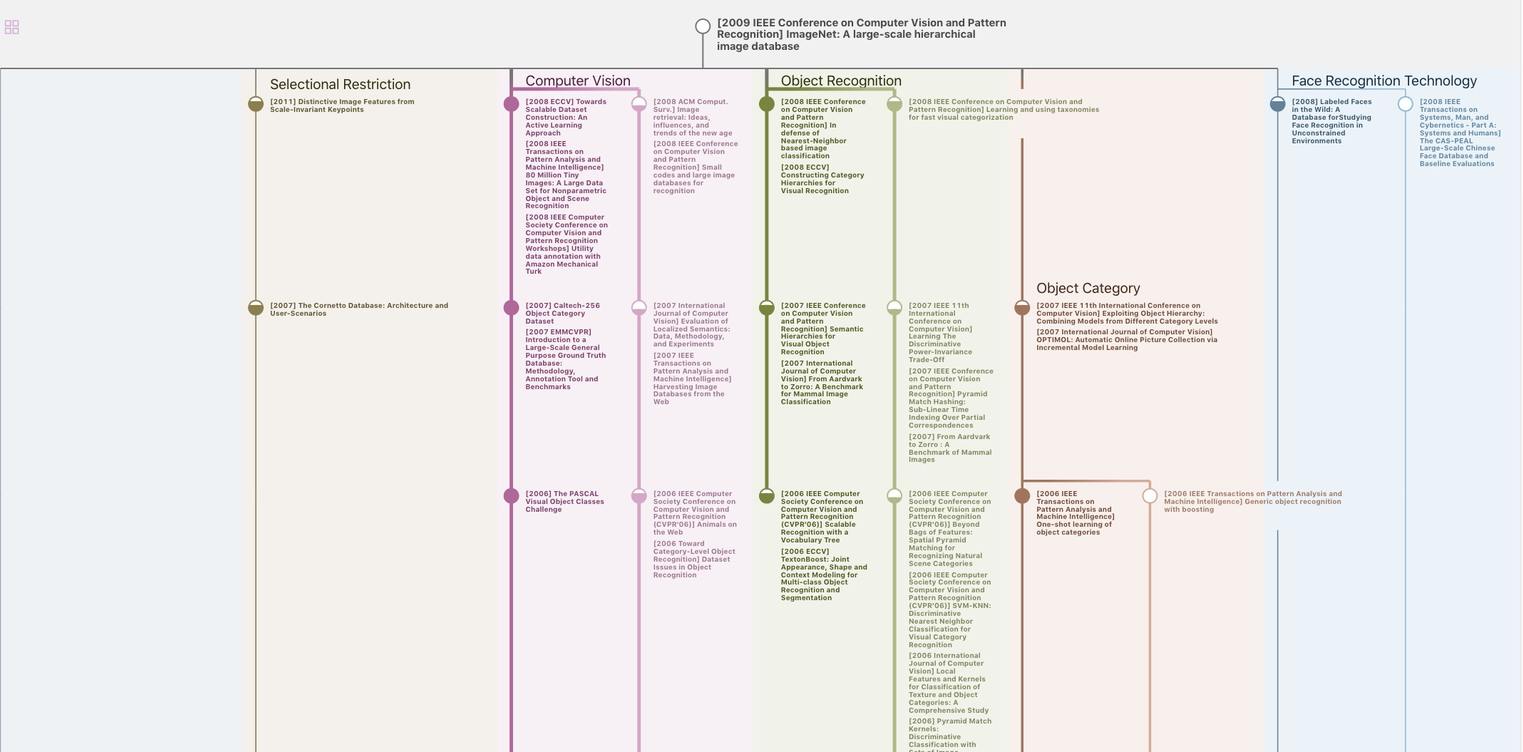
生成溯源树,研究论文发展脉络
Chat Paper
正在生成论文摘要