A Semisupervised Just-In-Time Learning Framework Based on Local Label Propagation and Its Application to Industrial Virtual Sensing
IEEE Sensors Journal(2023)
摘要
Just-In-Time Learning (JITL) has proven to be an effective tool for developing virtual sensors for complex industrial processes. However, most of the existing JITL-based virtual sensing methods are supervised, whereas labeled samples within a local area are usually particularly scarce. As a result, the performance of these JITL-based virtual sensors are unable to reach the fullest potentials. To this end, a semisupervised JITL (SsJITL) framework is proposed based on local label propagation (LLP), which is referred to as “LLP-SsJITL. ” In the LLP-SsJITL, a robust semisupervised relevant neighborhood dataset of the query sample is first formed by accounting for both spatially and temporally relevant information of the query sample. Then, with the aid of the technique of label propagation on the constructed neighborhood of the query sample, a semisupervised objective function is developed, which transforms the estimation task into an analytical model-free optimization problem. By doing so, abundant unlabeled data are fully exploited such that the shortcomings of the supervised JITL resulting from the scarcity of labeled samples are remedied. Two real-life industrial processes are used to evaluate the performance of the LLP-SsJITL framework, showing significantly improved performance by using the LLP-SsJITL framework.
更多查看译文
关键词
Just-in-time learning (JITL),label propagation,model-free optimization,semisupervised learning,virtual sensor
AI 理解论文
溯源树
样例
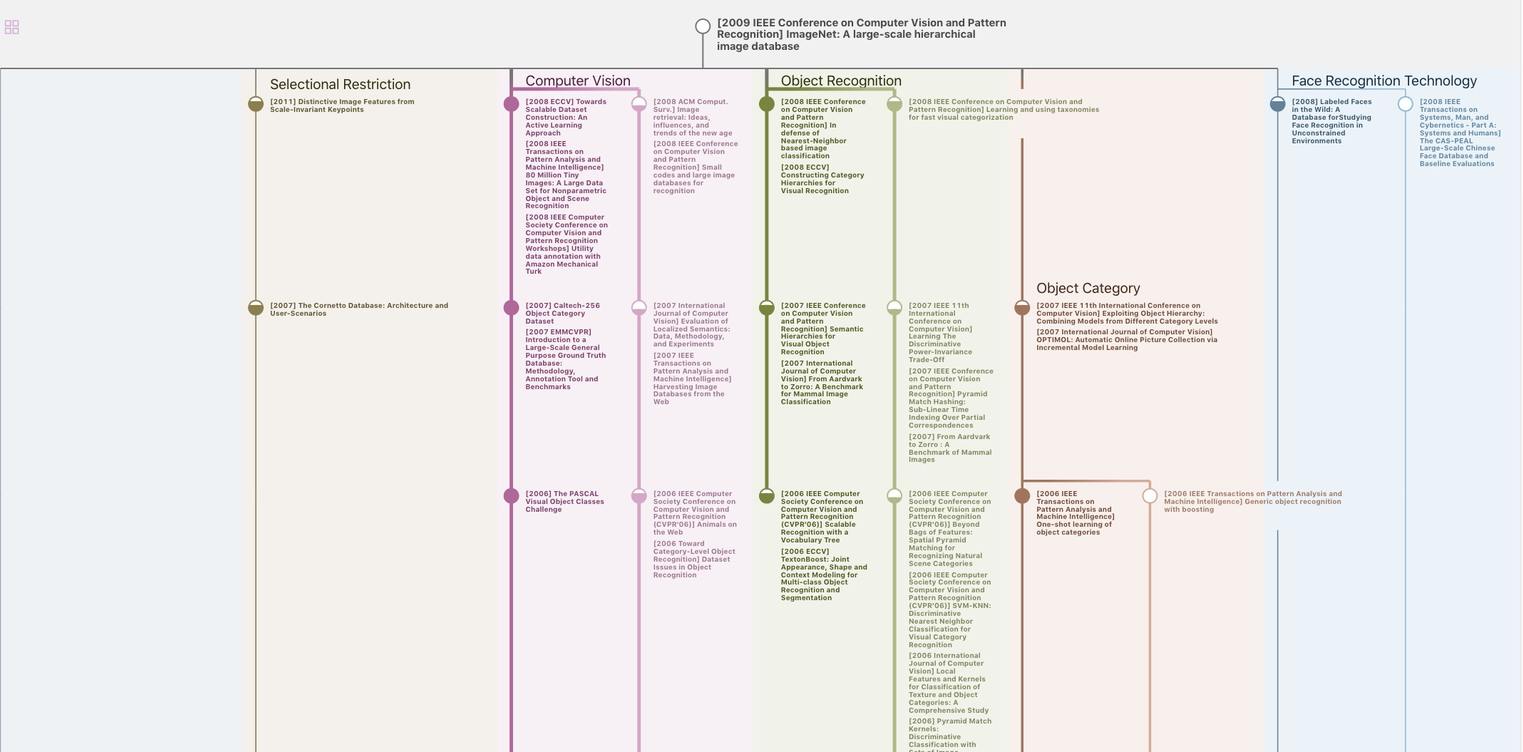
生成溯源树,研究论文发展脉络
Chat Paper
正在生成论文摘要