Learning-Based Inverse Kinematics Identification of the Tendon-Driven Robotic Manipulator for Minimally Invasive Surgery
IECON 2023- 49th Annual Conference of the IEEE Industrial Electronics Society(2023)
摘要
It is well-known that the tendon-driven robotic manipulator plays an important role in robotic-assisted minimally invasive surgery (MIS). However, due to the intrinsic nonlinearities, uncertainties, slack and hysteresis introduced by the tendon-driven actuation, the tendon-driven robotic manipulator is difficult to model and control when compared with the traditional actuation styles. To serve the modeling purpose, in this paper, the deep-learning-based intelligent modeling of inverse kinematics in the snake-like tendon-driven surgical instrument is presented. In the proposed approach the Deep Recurrent Neural Network (DRNN) with Long Short-Term Memory (LSTM) architecture is adopted to memorize and identify the nonlinear inverse kinematics of the tendon-driven surgical instrument through the history of the motor and tip positions. To collect highly reliable data to train the DRNN, the experiment to generate training data is carefully designed with the consideration of the stainless tendon characters and motor limitations. During the designed controller movements, the kinematics data is obtained by recording the motor positions and the tip positions. Besides, it is noticed that there are correlations of the sequential data samples, which could significantly reduce the modeling accuracy. To remove the correlations and improve the modeling performance, the correlations of the sequential data samples are removed by modifying the training processes. Modeling results and detailed discussions verified the effectiveness of the proposed approach.
更多查看译文
关键词
Robot learning,surgical robotics,deep recurrent neural networks,tendon-driven robotic manipulator,minimally invasive surgery
AI 理解论文
溯源树
样例
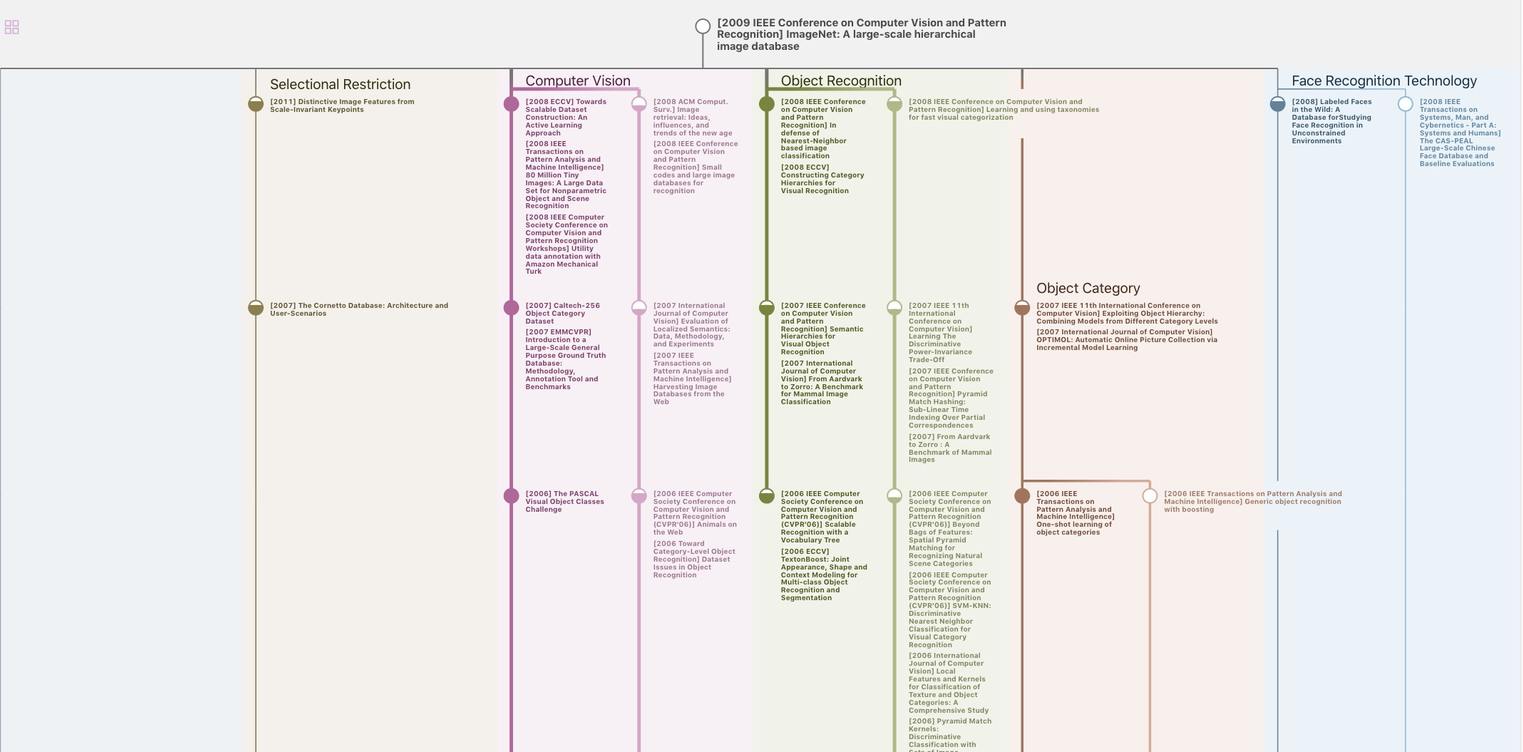
生成溯源树,研究论文发展脉络
Chat Paper
正在生成论文摘要