Learning Adaptive Cruise Control for Autonomous Vehicles Using End-to-End Deep Reinforcement Learning
IECON 2023- 49th Annual Conference of the IEEE Industrial Electronics Society(2023)
摘要
The most challenging task for autonomous vehicles (AVs) is to share the road with human-driven vehicles (HDVs), since the driving behaviors of HDVs are unknown to the AVs. And AVs are supposed to make optimal decisions in real time based on onboard sensors only. To achieve this goal, we model the problem as a Partially Observable Markov Decision Process (POMDP) and propose an end-to-end decision-making framework for AVs based on a deep reinforcement learning (DRL) algorithm in combination with classical control methods to allow vehicles to achieve an adaptive cruise control. To reduce the gap in the Sim2real problem, a high-fidelity simulator is developed using ROS-Gazebo, which allows for a realistic multi-vehicle simulation with various sensors. The raw data obtained from these sensors is the input of a LSTM neural network, which could be mapped directly to the low-level commands. Then, an adaptive driving policy will be learned automatically from the virtual environment through self-play training mode. Finally, the trained model is tested in both virtual platform and corresponding real-world scenario, which validates the effectiveness and feasibility of the proposed decision-making framework.
更多查看译文
关键词
Deep reinforcement learning,sim-to-real,self-driving car,ROS-Gazebo
AI 理解论文
溯源树
样例
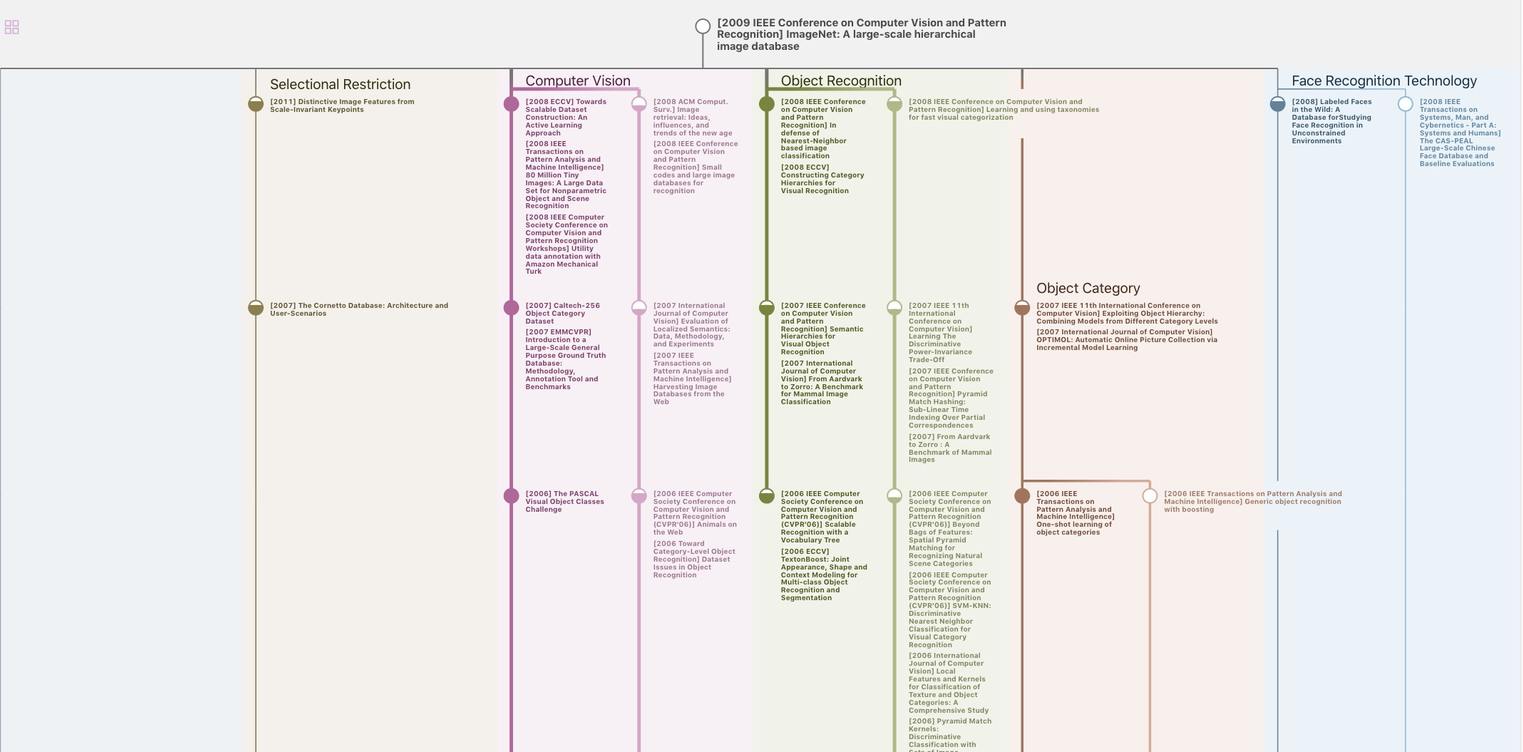
生成溯源树,研究论文发展脉络
Chat Paper
正在生成论文摘要