Finite-Sample Bounds for Two-Distribution Hypothesis Tests
2023 IEEE 10th International Conference on Data Science and Advanced Analytics (DSAA)(2023)
摘要
With the rapid growth of large language models, big data, and malicious online attacks, it has become increasingly important to have tools for anomaly detection that can distinguish machine from human, fair from unfair, and dangerous from safe. Prior work has shown that two-distribution (specified complexity) hypothesis tests are useful tools for such tasks, aiding in detecting bias in datasets and providing artificial agents with the ability to recognize artifacts that are likely to have been designed by humans and pose a threat. However, existing work on two-distribution hypothesis tests requires exact values for the specification function, which can often be costly or impossible to compute. In this work, we prove novel finite-sample bounds that allow for two-distribution hypothesis tests with only estimates of required quantities, such as specification function values. Significantly, the resulting bounds do not require knowledge of the true distribution, distinguishing them from traditional p-values. We apply our bounds to detect student cheating on multiple-choice tests, as an example where the exact specification function is unknown. We additionally apply our results to detect representational bias in machine-learning datasets and provide artificial agents with intention perception, showing that our results are consistent with prior work despite only requiring a finite sample of the space. Finally, we discuss additional applications and provide guidance for those applying these bounds to their own work.
更多查看译文
关键词
statistics,hypothesis testing,specified complexity,anomaly detection,machine learning,bias,artificial agents
AI 理解论文
溯源树
样例
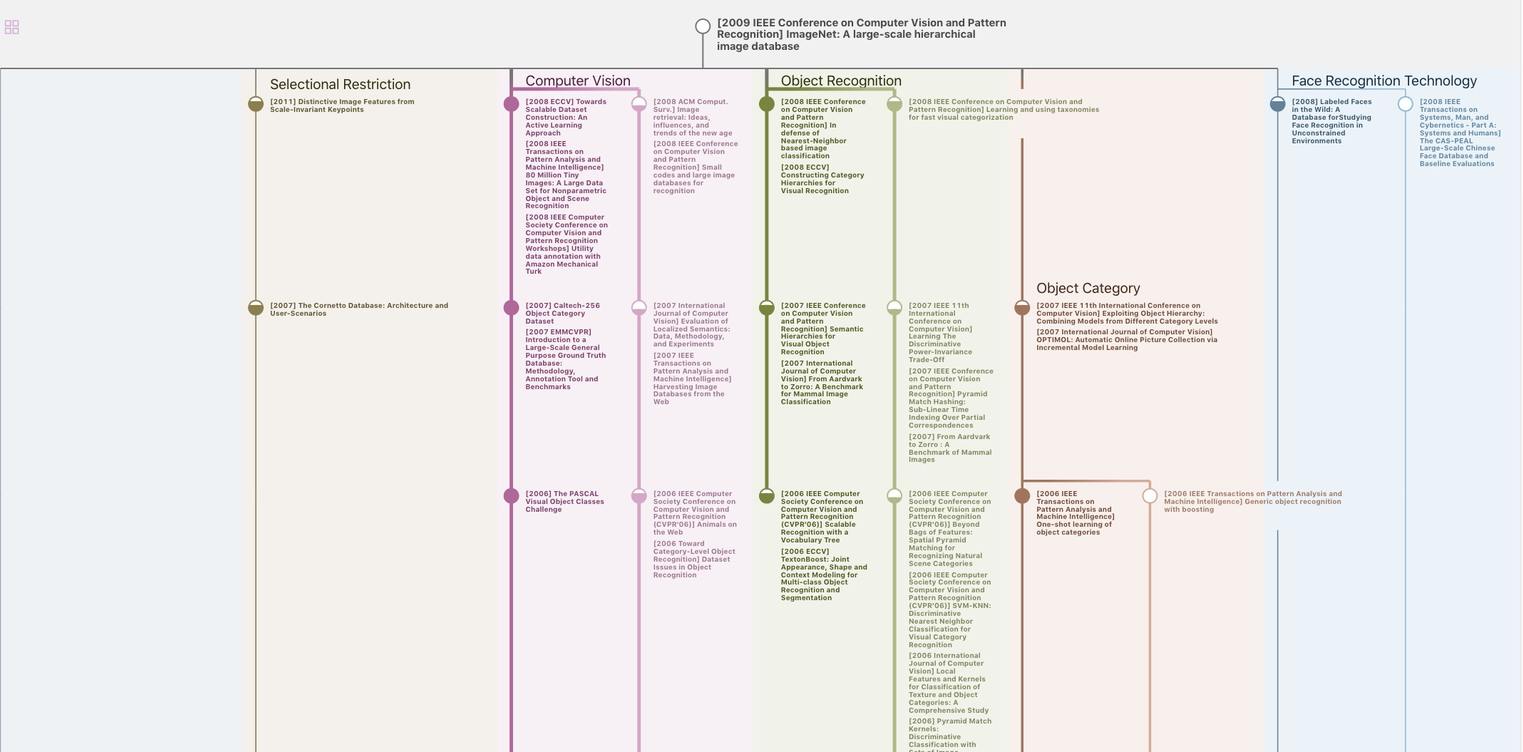
生成溯源树,研究论文发展脉络
Chat Paper
正在生成论文摘要