Entity recognition based on heterogeneous graph reasoning of visual region and text candidate
Machine Learning(2024)
摘要
Entity recognition plays a crucial role in various domains, such as natural language processing, information retrieval, and question-answering systems. While significant progress has been made in recognizing entities from plain text, the exploration of entity recognition from multimodal data remains limited due to disparities in semantic representation. In light of this challenge, given the supportive nature of visual and text data, we propose a novel entity recognition model called Heterogeneous Graph Reasoning(HGR), leveraging the synergistic nature of visual and textual data. HGR utilizes image objects to facilitate text entity extraction by mining the potential pair projection between text entity and image object. This is achieved through the utilization of the Vision Refine and Graph Cross Inference modules. In the Vision Refine module, semantically relevant objects hidden in the image are selected to aid in the text entity extraction. In the Graph Cross Inference module, cross-association inference between visual regions and textual entities is constructed through graph construction, heterogeneous graph fusion, visual region refinement and cross inference. To validate the effectiveness of our model, extensive experiments on four multimodal datasets are conducted. Among these datasets, two originate from Chinese unmanned surface vehicles and journalism(USV and NEWS), while the remaining two are public English multimodal datasets(Twitter-2015 and Twitter-2017). The experimental results demonstrate the superiority of our model, with F1-sore improvements of 1.55%, 0.12%, 0.22%, and 0.99% on the four datasets, respectively, when compared to the second-best state-of-the-art model.
更多查看译文
关键词
Entity recognition,Heterogeneous graph reasoning,Graph cross inference
AI 理解论文
溯源树
样例
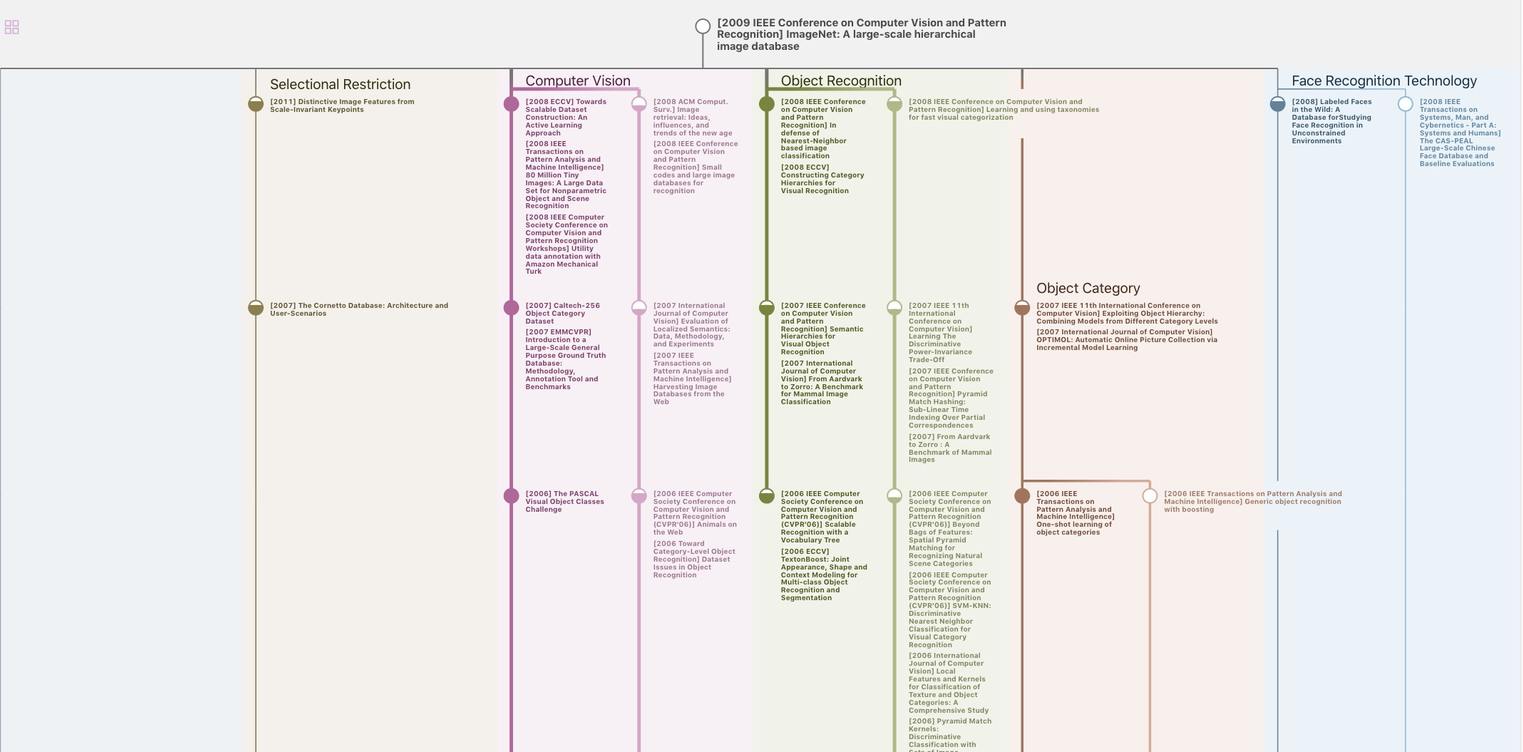
生成溯源树,研究论文发展脉络
Chat Paper
正在生成论文摘要