Sensor Fault Detection for UAVs Based on MIC-LSTM With Attention Mechanism
2023 CAA Symposium on Fault Detection, Supervision and Safety for Technical Processes (SAFEPROCESS)(2023)
摘要
The unmanned aerial vehicle (UAV) sensors are indispensable parts of UAVs, and detecting their faults is of great significance for the safe flight of the entire UAV. The existing fault detection methods have limitations in selecting input variables related to flight data, and do not fully consider the contribution of relevant data in the methods. In order to solve these problems, this paper proposes a sensor fault detection method (MICA-LSTM) based on maximum information coefficient (MIC) and long short-term memory network (LSTM) with attention mechanism. Firstly, MIC is used to select input variables related to the flight data to be detected, reducing interference from irrelevant data. Subsequently, an LSTM model with attention mechanism is used to train the selected input time series data and construct a UAV fault detection model. This method can extract relevant features from large flight data and assign different weights to these features based on different time series, thus achieving accurate fault detection. The proposed method is compared with methods lacking MIC and attention mechanism through experimental validation using simulated data from the University of Minnesota UAV model. The results indicate that the proposed method exhibits better performance and accuracy in UAV sensor fault detection.
更多查看译文
关键词
unmanned aerial vehicle (UAV),fault detection,maximum information coefficient (MIC),long short-term memory network (LSTM),attention mechanism
AI 理解论文
溯源树
样例
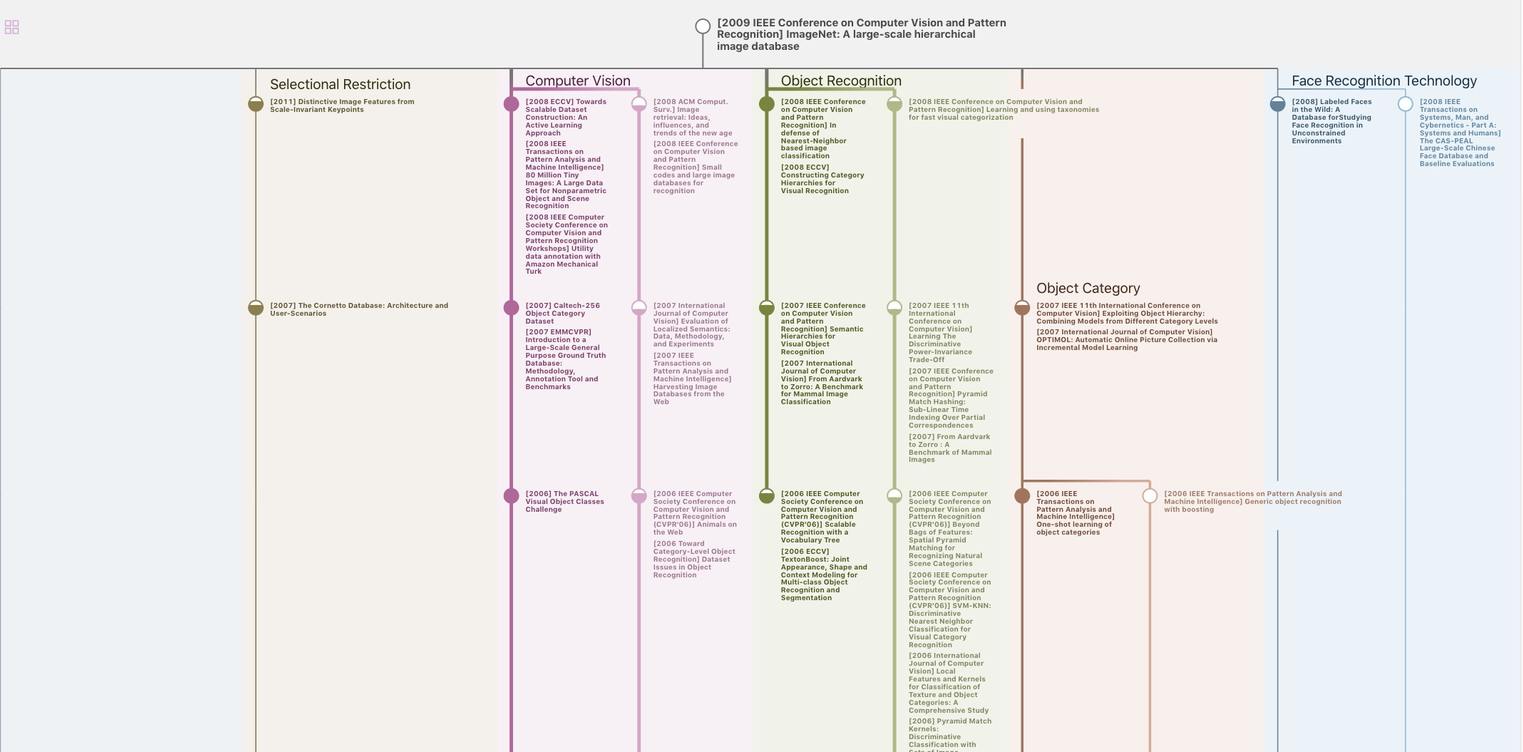
生成溯源树,研究论文发展脉络
Chat Paper
正在生成论文摘要