Anomalous Update Identification Based on Cosine Similarity for Collaborative Wind Power Forecasting
2023 IEEE/IAS INDUSTRIAL AND COMMERCIAL POWER SYSTEM ASIA, I&CPS ASIA(2023)
摘要
Wind power forecasting based on spatial correlation works in a collaborative way to exploit the information of neighboring wind farms to improve the overall forecasting accuracy, but the collaborative forecasting approach needs to centralize the original data of each site, which leads to the problem of data privacy preservation. The Federated Learning framework is applied to collaborative wind power forecasting, allowing the original data of each site to be stored locally for data privacy preservation. A method of identifying sites with anomalous update based on cosine similarity is proposed, based on which anomalous sites are eliminated in the subsequent collaborative forecasting process based on federated learning. A case study from 30 wind farms demonstrates that the proposed approach is effective to identify sites with anomalous update and achieves a forecasting accuracy consistent with the normal situation after eliminating the anomalous sites.
更多查看译文
关键词
wind power forecasting,federated learning,anomalous data identification,privacy-preserving
AI 理解论文
溯源树
样例
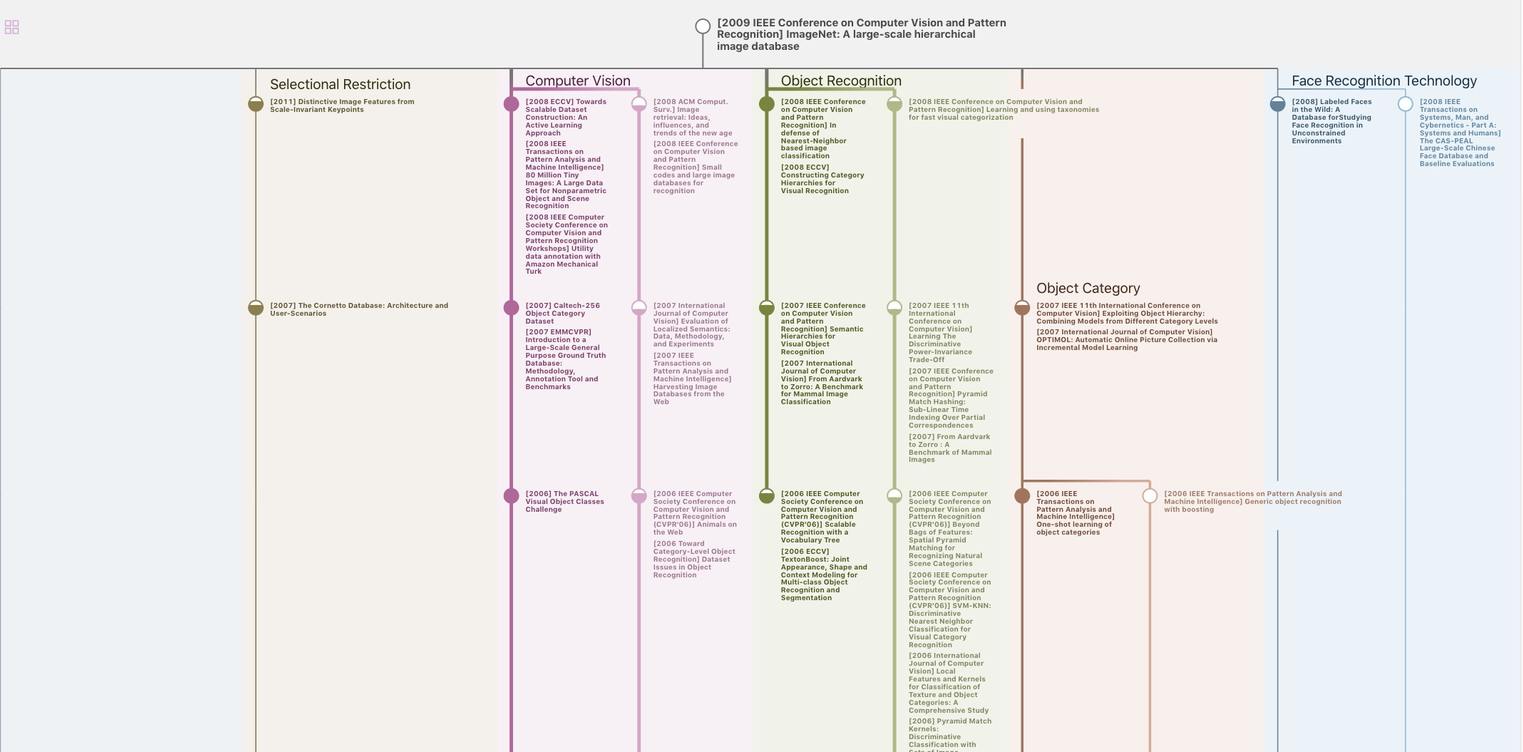
生成溯源树,研究论文发展脉络
Chat Paper
正在生成论文摘要