State of Health Estimation for Lithium-ion Batteries by Using Partial Battery Data with a Hybrid Neural Network Model
2023 IEEE/IAS INDUSTRIAL AND COMMERCIAL POWER SYSTEM ASIA, I&CPS ASIA(2023)
摘要
The growing utilization of lithium-ion batteries in new energy vehicles is emerging as a promising solution to address the global energy crisis and issues related to environmental pollution. Ensuring battery safety and reliability relies heavily on the precise assessment of the SOH, which is of utmost importance. However, the historical state information of batteries used in most data-driven methods may be incomplete or missing, especially in scenarios with small data sizes. In this research, we introduce a sliding window method to reconstruct battery charge and discharge data. This approach involves extracting voltage and current data segments during the battery's charging and discharging stages. These segments are used to construct a VI matrix. We propose a CNN-LSTM deep neural network model for estimating the SOH of lithium-ion batteries. Our model uses two CNN neural network layers to extract frequency domain information from the battery segment data and an LSTM neural network layer to extract the time-domain features of the data. The superiority and generalization capability of the proposed model was validated on the lithium-ion batteries dataset from CALCE by comparing the estimation results with those of single CNN and LSTM models.
更多查看译文
关键词
State of Health,CNN,LSTM,Sliding window,Lithium-ion batteries
AI 理解论文
溯源树
样例
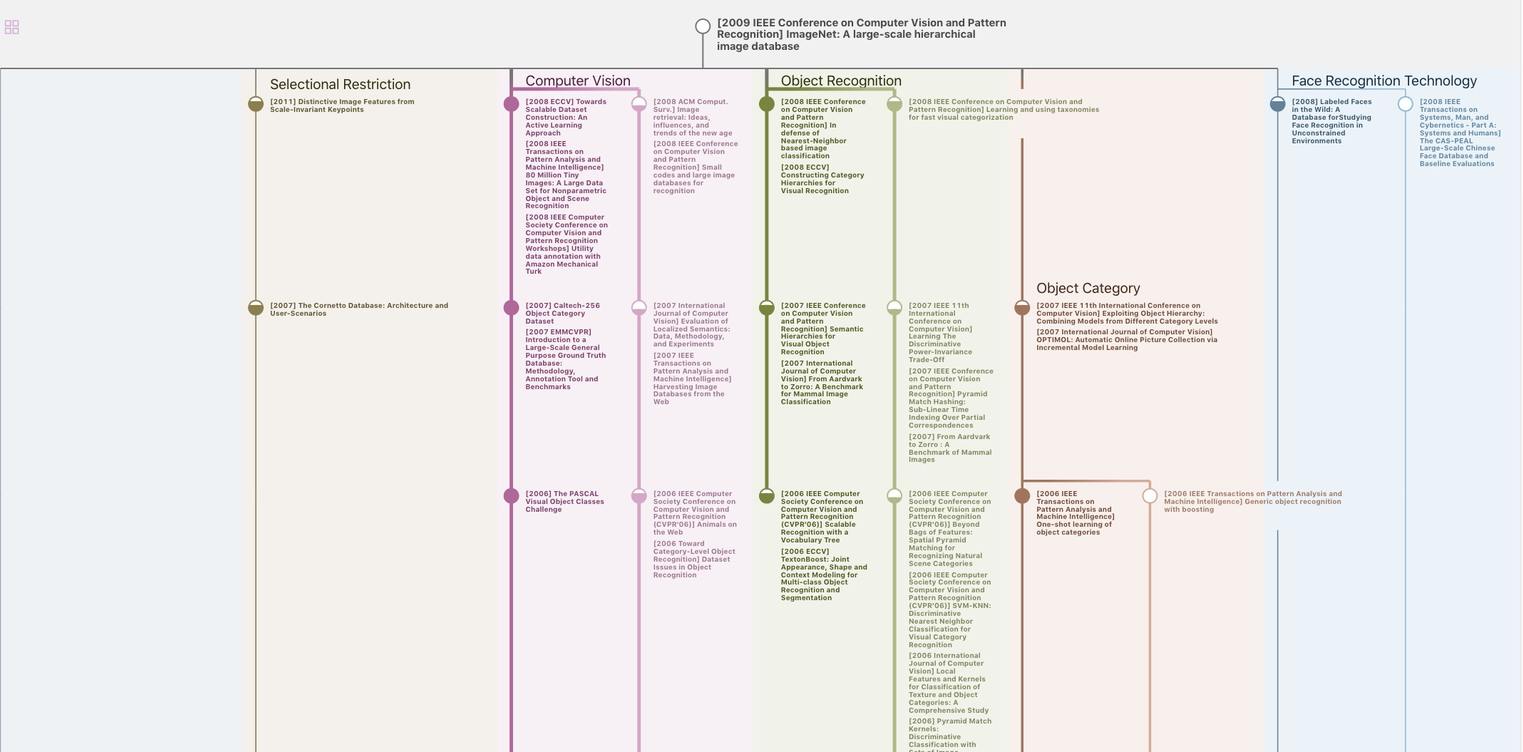
生成溯源树,研究论文发展脉络
Chat Paper
正在生成论文摘要