DRJLRA: A Deep Reinforcement Learning-Based Joint Load and Resource Allocation in Heterogeneous Coded Distributed Computing
2023 IEEE 34TH ANNUAL INTERNATIONAL SYMPOSIUM ON PERSONAL, INDOOR AND MOBILE RADIO COMMUNICATIONS, PIMRC(2023)
摘要
In this paper, we introduce the DRJLRA algorithm, a load and resource allocation scheme based on deep reinforcement learning (DRL) for a generic multi-master, multiworker coded distributed computing (CDC) system. Our aim is to minimize the combined delay of communication and computation for a set of matrix-vector multiplication tasks. The proposed DRL-based approach has several unique features that set it apart from existing literature. Firstly, it is applicable to general CDC systems with multiple masters and workers. Additionally, it considers multi-task CDC systems with stochastic task arrivals, takes into account the heterogeneity of workers with random computation and communication delays, and utilizes the state-of-the-art soft actor-critic (SAC) DRL algorithm, making it versatile and efficient in handling complex and dynamic CDC environments. Our results demonstrate that DRJLRA outperforms benchmark schemes significantly. It is thus well-suited for real-world CDC systems with diverse and dynamic workloads.
更多查看译文
关键词
Coded distributed computing,joint load and resource allocation,deep reinforcement learning,soft actor-critic
AI 理解论文
溯源树
样例
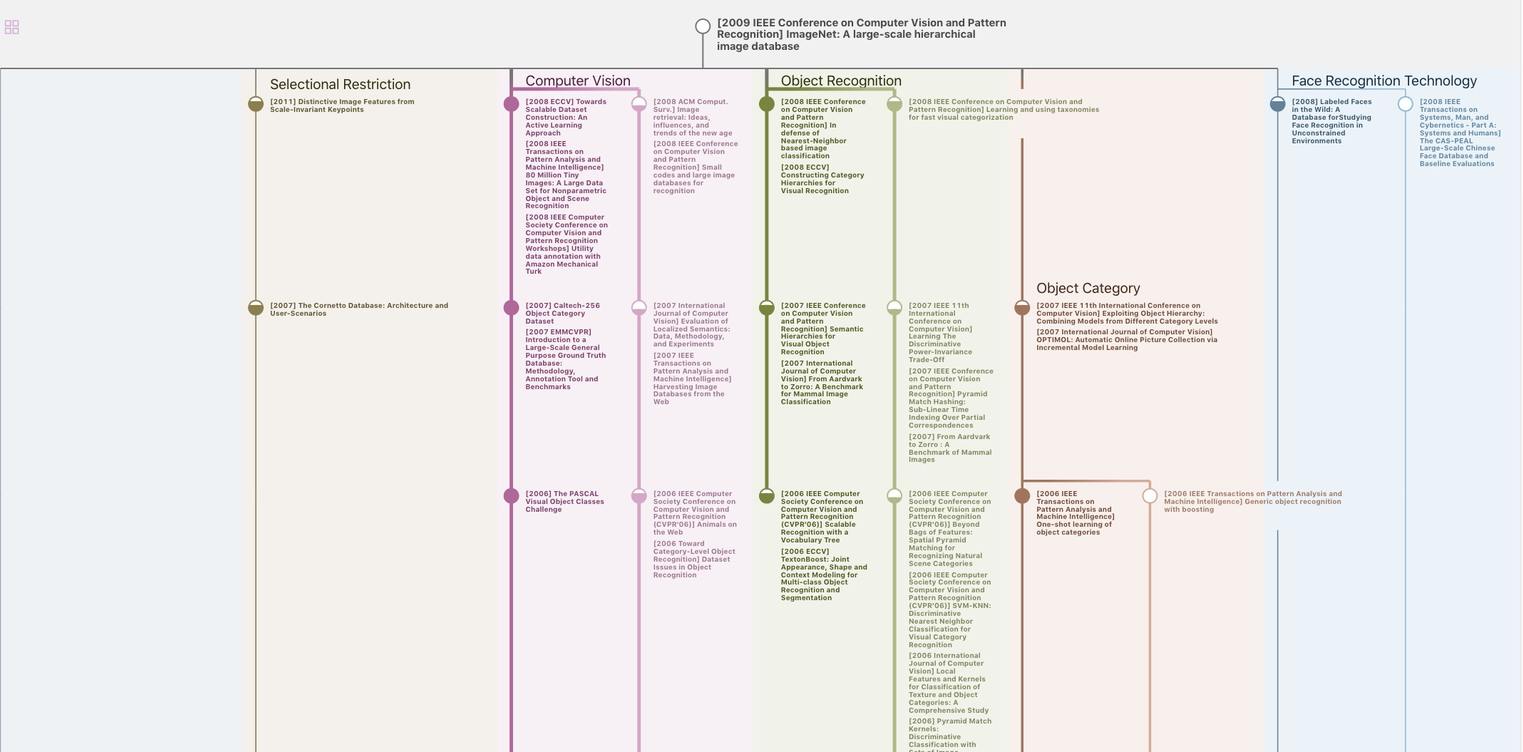
生成溯源树,研究论文发展脉络
Chat Paper
正在生成论文摘要