Learned Image Transmission over MIMO Fading Channels.
2023 IEEE 34TH ANNUAL INTERNATIONAL SYMPOSIUM ON PERSONAL, INDOOR AND MOBILE RADIO COMMUNICATIONS, PIMRC(2023)
摘要
Learned image transmission (LIT) has shown promising progress in recent years to boost the end-to-end transmission performance in semantic communications. To further enhance the system efficiency, in this paper, we propose a novel LIT framework built on multiple-input multiple-output (MIMO) fading channels. In particular, the proposed framework supports concurrent transmission of multiple streams, which can maximize the multiplexing gain in end-to-end semantic communication systems. By jointly considering the entropy distribution of the image semantic features and the wireless MIMO channel states, we design a spatial multiplexing mechanism that can adaptively realize coding rate allocation and stream mapping. As a result, source content and channel environment will be seamlessly coupled, which maximizes the coding gain. Moreover, the proposed LIT model is versatile: a single model can support various transmission rates. The whole model is optimized under the constraint of transmission rate-distortion (RD) tradeoff. Experimental results verify that our scheme substantially increases the throughput of semantic communication systems, and outperforms traditional MIMO communication systems under realistic fading channels.
更多查看译文
关键词
channel environment,end-to-end semantic communication systems,end-to-end transmission performance,image semantic features,learned image transmission,LIT framework,LIT model,MIMO communication systems,MIMO fading channels,multiple-input multiple-output fading channels,realistic fading channels,semantic communication systems,spatial multiplexing mechanism,transmission rate-distortion tradeoff,wireless MIMO channel states
AI 理解论文
溯源树
样例
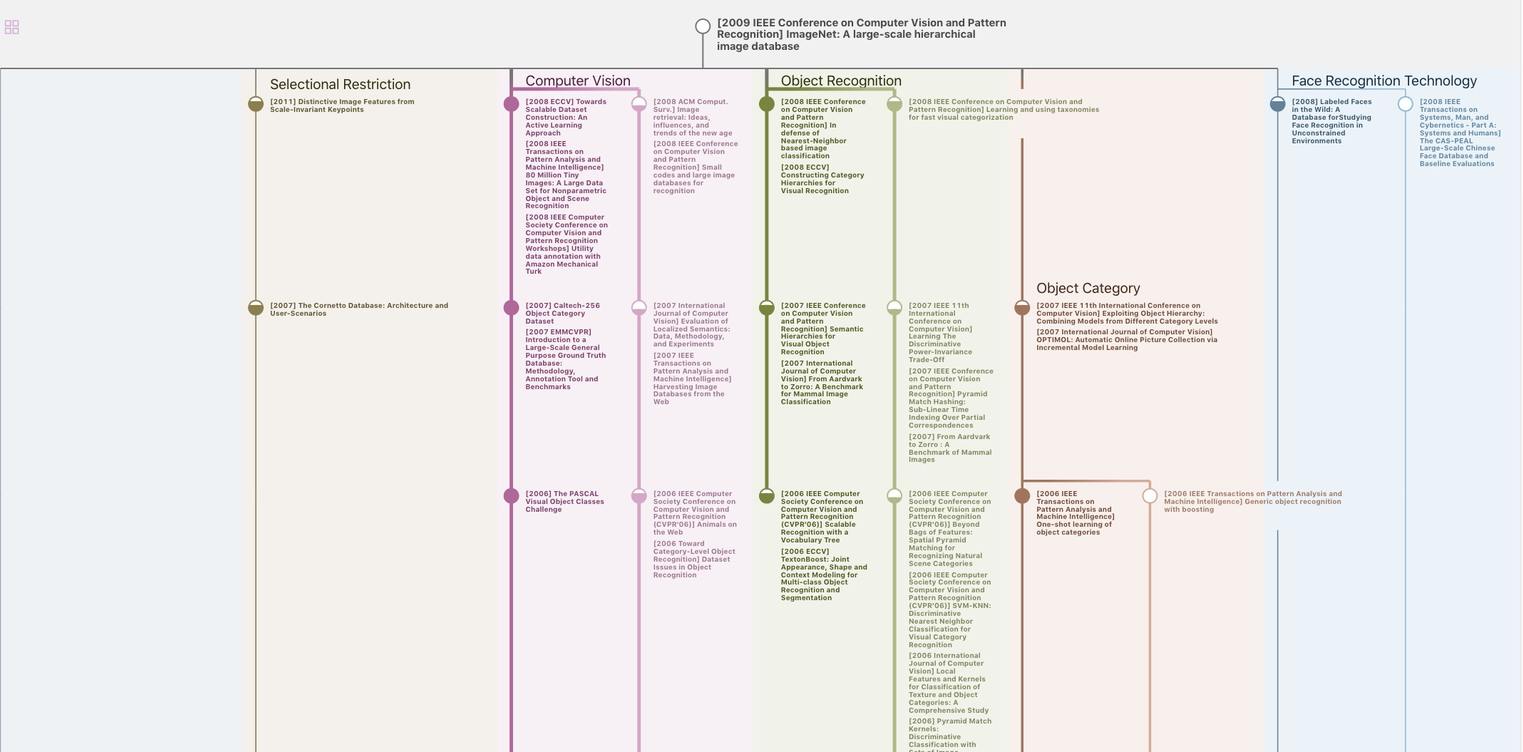
生成溯源树,研究论文发展脉络
Chat Paper
正在生成论文摘要