Deep Reinforcement Learning for Downlink Scheduling in 5G and Beyond Networks: A Review
2023 IEEE 34TH ANNUAL INTERNATIONAL SYMPOSIUM ON PERSONAL, INDOOR AND MOBILE RADIO COMMUNICATIONS, PIMRC(2023)
摘要
The coexistence of a wide variety of different applications with diverse Quality of Service (QoS) and Quality of Experience (QoE) requirements calls for more sophisticated radio resource scheduling in 5G and beyond (5GB) networks compared to previous generations. To address this challenge, a growing body of research has explored deep reinforcement learning (DRL) to solve the radio resource scheduling problem. In this paper, we review representative literature on the topic of downlink scheduling for 5GB networks using DRL, with emphasis on fine-grained approaches that directly allocate resource blocks (RBs) to user equipments (UEs). We conclude by discussing four ways to improve upon this early-stage research and identify some open problems that must be solved to make DRL a viable solution to the downlink scheduling problem in 5GB networks.
更多查看译文
AI 理解论文
溯源树
样例
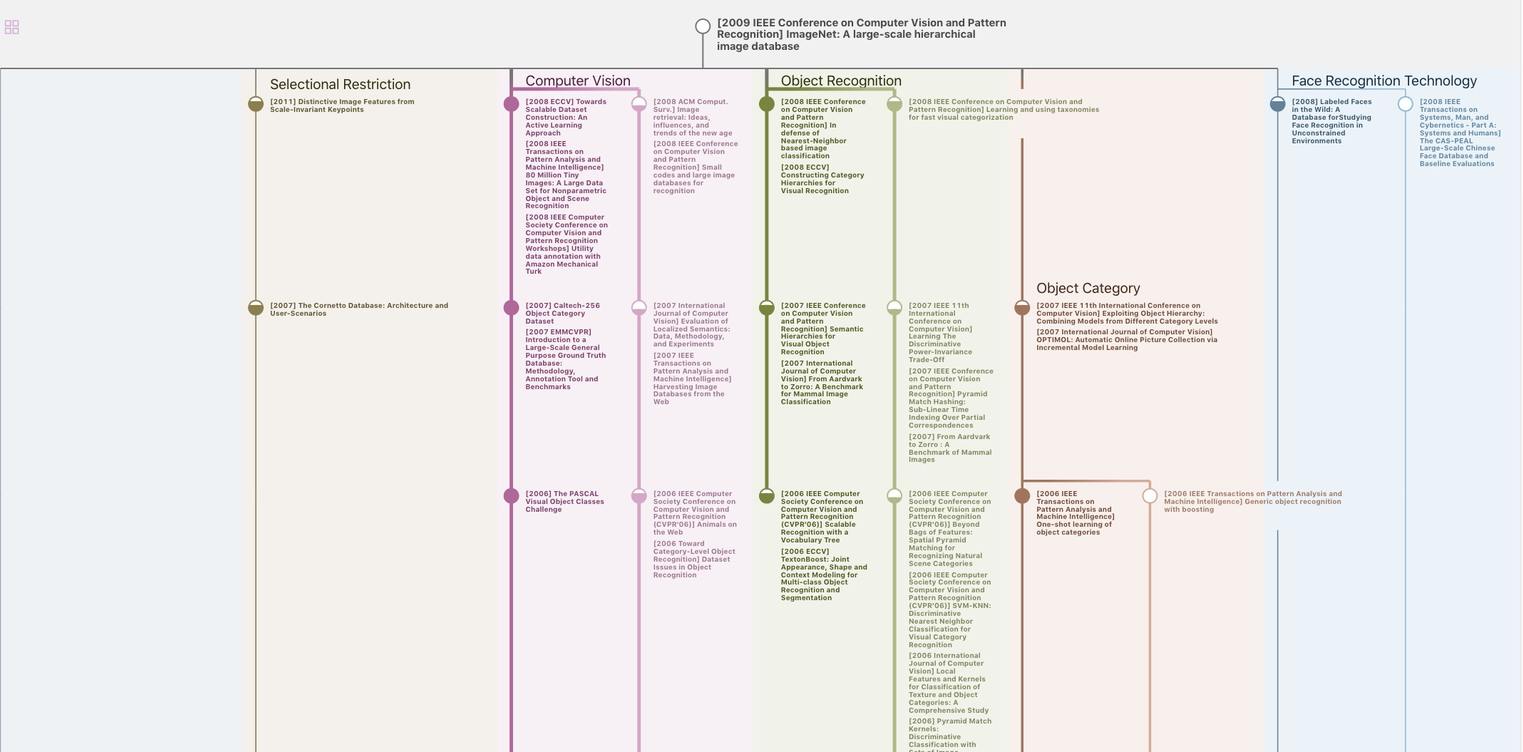
生成溯源树,研究论文发展脉络
Chat Paper
正在生成论文摘要