Deep Reinforcement Learning Enabled Power Allocation for Multi-Connectivity C-V2X Downlink
2023 IEEE 34TH ANNUAL INTERNATIONAL SYMPOSIUM ON PERSONAL, INDOOR AND MOBILE RADIO COMMUNICATIONS, PIMRC(2023)
摘要
Cellular vehicle-to-everything (C-V2X) network is a promising solution to support on road diverse quality of services (QoS) such as ultra reliable low latency communication (URLLC) and enhanced mobile broadband (eMBB). However, satisfying the stringent QoS requirements in high-dynamic C-V2X environment is very challenge. In this paper, we leverage the multi-connectivity technology to enhance the reliability of downlink URLLC in C-V2X. Specifically, with the aid of the cloud radio access network (C-RAN), the network controller duplicates each URLLC packet and transmits its replicas over multiple independent wireless links. To ensure the reliability of URLLC links while maximizing the average rate of eMBB links, we design a coordinated multi-agent deep reinforcement learning algorithm for real-time power allocation of multi-connectivity URLLC links. Each URLLC link is treated as an agent here, and its transmit power is its action. The multiple links serving the same URLLC user are coordinated with a three-layer neural network for information sharing, allowing them to cooperatively choose transmit powers in terms of ensuring reliability while minimizing inter-cell interference and energy consumption. Extensive simulation results validate the effectiveness of the proposed power allocation algorithm for multi-connectivity downlink URLLC.
更多查看译文
关键词
multi-connectivity,packet duplication,URLLC,deep reinforcement learning,C-V2X
AI 理解论文
溯源树
样例
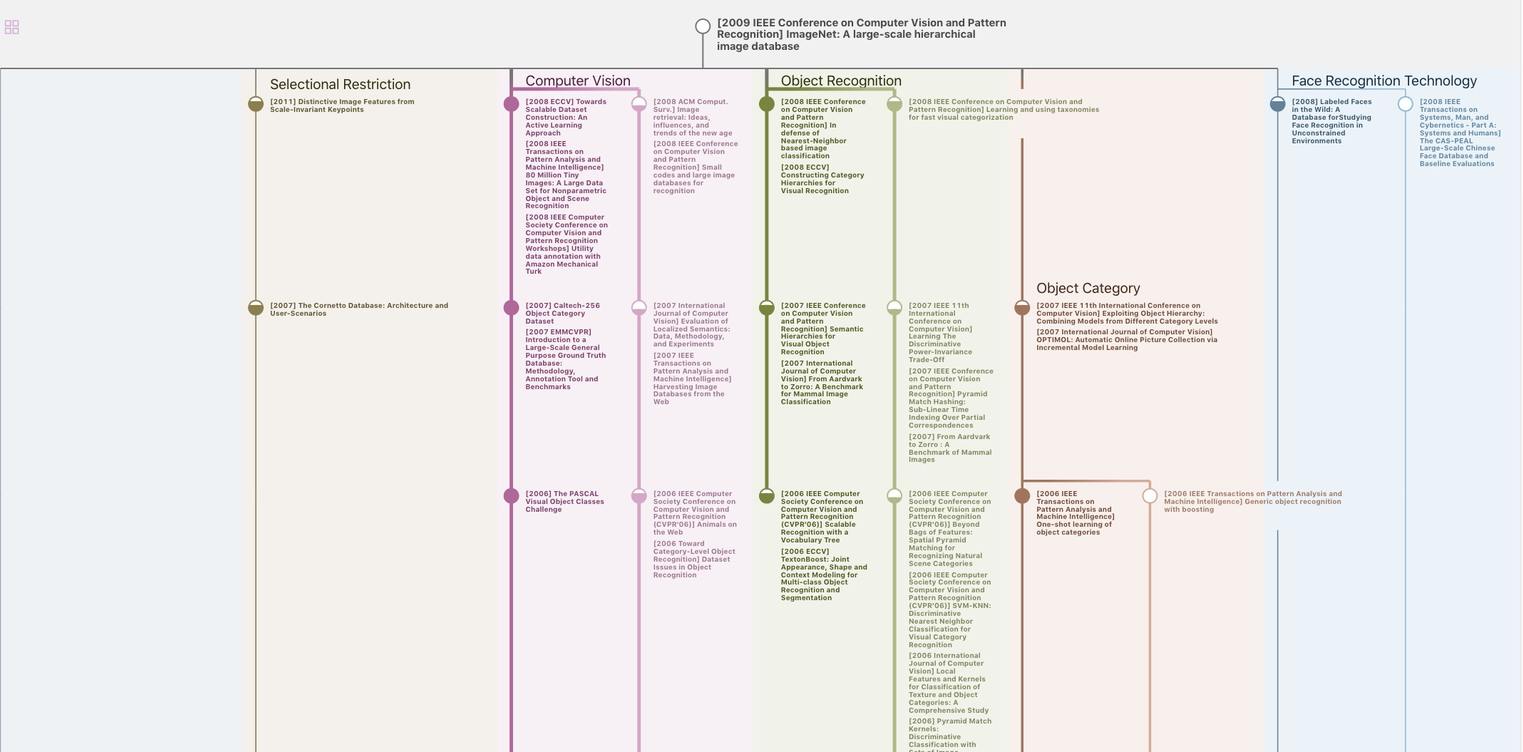
生成溯源树,研究论文发展脉络
Chat Paper
正在生成论文摘要