Privacy-Driven Fine-Grained Data Trading
2023 IEEE 34th Annual International Symposium on Personal, Indoor and Mobile Radio Communications (PIMRC)(2023)
摘要
In this paper, we investigate actual exchange-assisted data trading systems and point out that the increment of data content in a sensitive dataset always results in the increment of its privacy level, i.e., making the dataset more sensitive than before. As a consequence, data trading always follows an incremental privacy-driven paradigm, where (1) buyers with various requirements would purchase subsets of the data with different privacy levels, and (2) when a buyer purchases a subset of the entire dataset with a higher level of privacy, the subsets with all lower levels of privacy are required (in other words, there is a containment relationship between subsets with different levels of privacy). A notable example is attribute-value type datasets. Based on these observations, we propose a new concept of privacy-driven and fine-grained data trading, which enables sellers and buyers to trade in data in an efficient and flexible way. We propose a concrete instantiation, dubbed PDFG, which enables sellers and buyers to conduct fine-grained data trading with minimal costs in terms of computation and communication. We prove that PDFG is indistinguishable against the chosen plaintext attack (CPA) under the real-or-random (RoR) model. We also conduct a comprehensive performance evaluation to demonstrate the practicality and efficiency of PDFG.
更多查看译文
关键词
data trading,privacy evaluation,subsequent-key-locked encryption,entropy-weighting
AI 理解论文
溯源树
样例
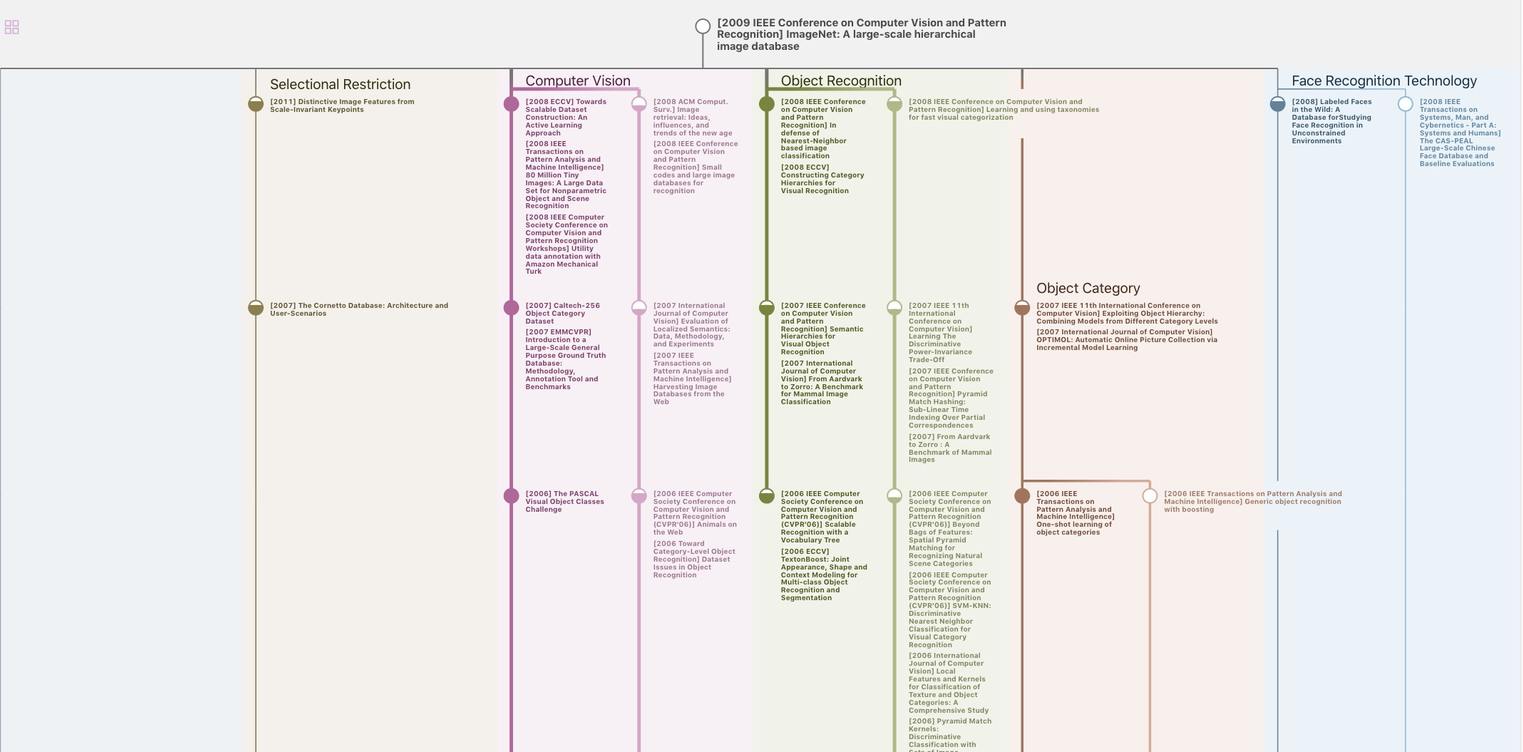
生成溯源树,研究论文发展脉络
Chat Paper
正在生成论文摘要