Online Traffic Prediction in Multi-RAT Heterogeneous Network: A User-Cybertwin Asynchronous Learning Approach
2023 IEEE 34TH ANNUAL INTERNATIONAL SYMPOSIUM ON PERSONAL, INDOOR AND MOBILE RADIO COMMUNICATIONS, PIMRC(2023)
Abstract
In this paper, we propose a novel traffic prediction scheme for multiple radio access technology (multi-RAT) heterogeneous network. The scheme is named user-Cybertwin asynchronous learning (UCAL), which aims to extract meaningful patterns from noisy network traffic measurements and mitigate the impact of highly nonstationary measurements for ensuring the prediction accuracy. Specifically, we design a pattern extraction method that minimizes the Frobnius norm between the collected measurements and the expected k-rank approximation of the measurements in order to extract useful information. Then, by transforming the conventional long short term memory (LSTM) model into a nonlinear state space and incorporating Gaussian noise, we develop an online LSTM algorithm to adapt fast to changing environments. As a result, the parameter updating of the new online LSTM model can keep up with data changes while capturing complicated and nonlinear relationships among measurements. We consider both the surrounding environment conditions on the mobile user side and end-to-end link conditions on the Cybertwin side, and iteratively update the model parameters in both Cybertwin and MU. Simulation results demonstrate that the proposed UCAL scheme can achieve high traffic prediction accuracy in comparison to existing schemes. It can also significantly improve the efficiency in maintaining the prediction accuracy even when the dimension of traffic measurements increases.
MoreTranslated text
AI Read Science
Must-Reading Tree
Example
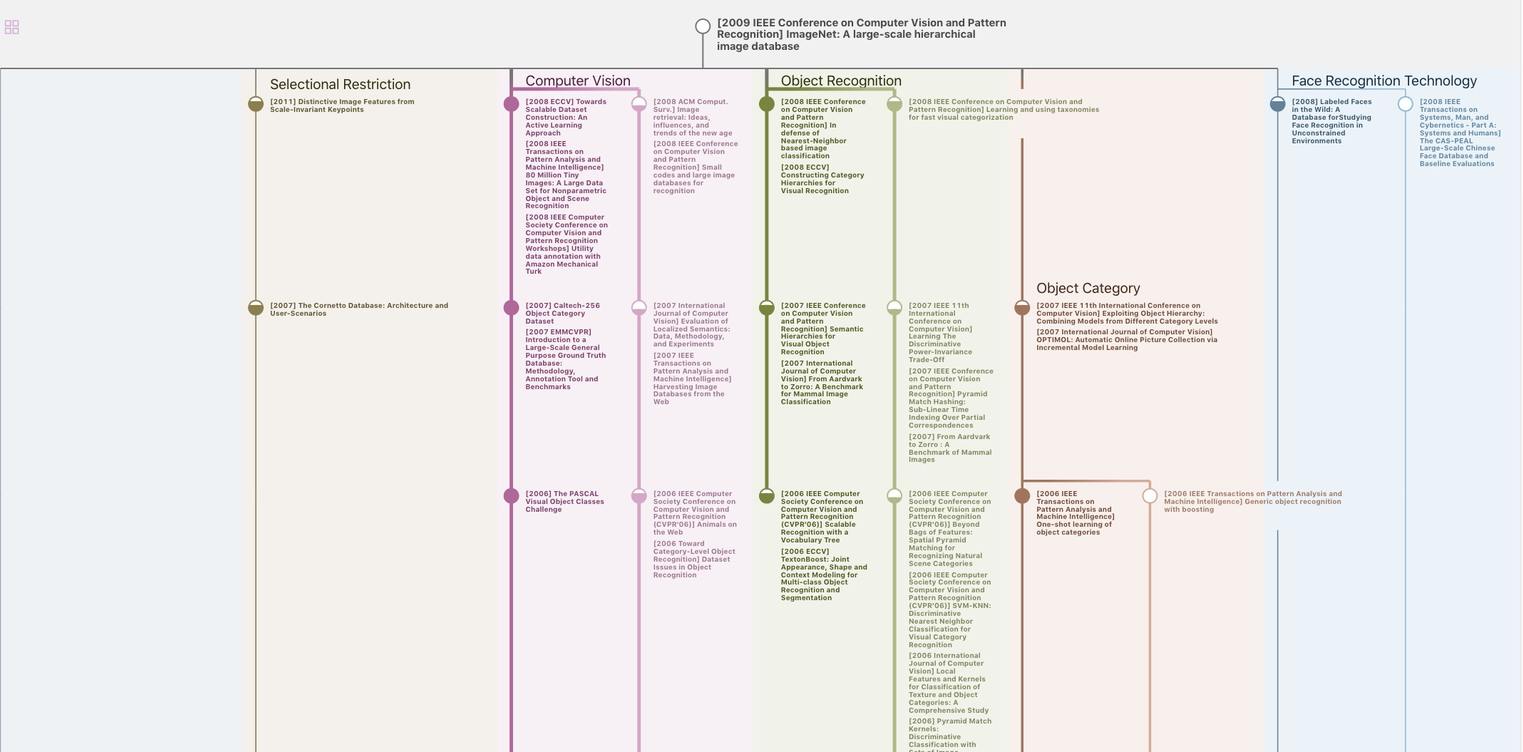
Generate MRT to find the research sequence of this paper
Chat Paper
Summary is being generated by the instructions you defined