Obfuscated Malware detection using Machine Learning models
2023 14th International Conference on Computing Communication and Networking Technologies (ICCCNT)(2023)
摘要
The number of malware attacks has been growing at an alarming rate especially in the recent years. Cyber criminals equip themselves with the latest technologies and discover new methods of attack every day. Therefore, researchers have paid a lot of attention to malware detection in order to lower malware threats. Static analysis or behaviour analysis are used in majority of the investigative experiments. New studies indicate that modern malware files employ diverse techniques to evade detection and analysis, rendering both types of analyses susceptible. This renders the extracted features potentially useless and only serves as a diversion for malware analysts. However, volatile memory holds significant potential for extracting valuable data and insights regarding the characteristics and behaviors of malware. Furthermore, memory analysis possesses the capability to detect atypical forms of malware, such as malware residing in computer memory (in-memory) and malware that operates without leaving a trace on the disk (fileless). The study aims to detect malware attacks with high accuracy by employing feature engineering followed by usage of machine learning methods. In this present paper we examine memory data from the CIC-Malmem-2022 dataset containing threat analysis data, the algorithms used for malware detection along with their performance metrics and compare the various Machine Learning models and their use.
更多查看译文
关键词
Malware attacks,cybersecurity,memory analysis,machine learning,feature engineering,performance metrics
AI 理解论文
溯源树
样例
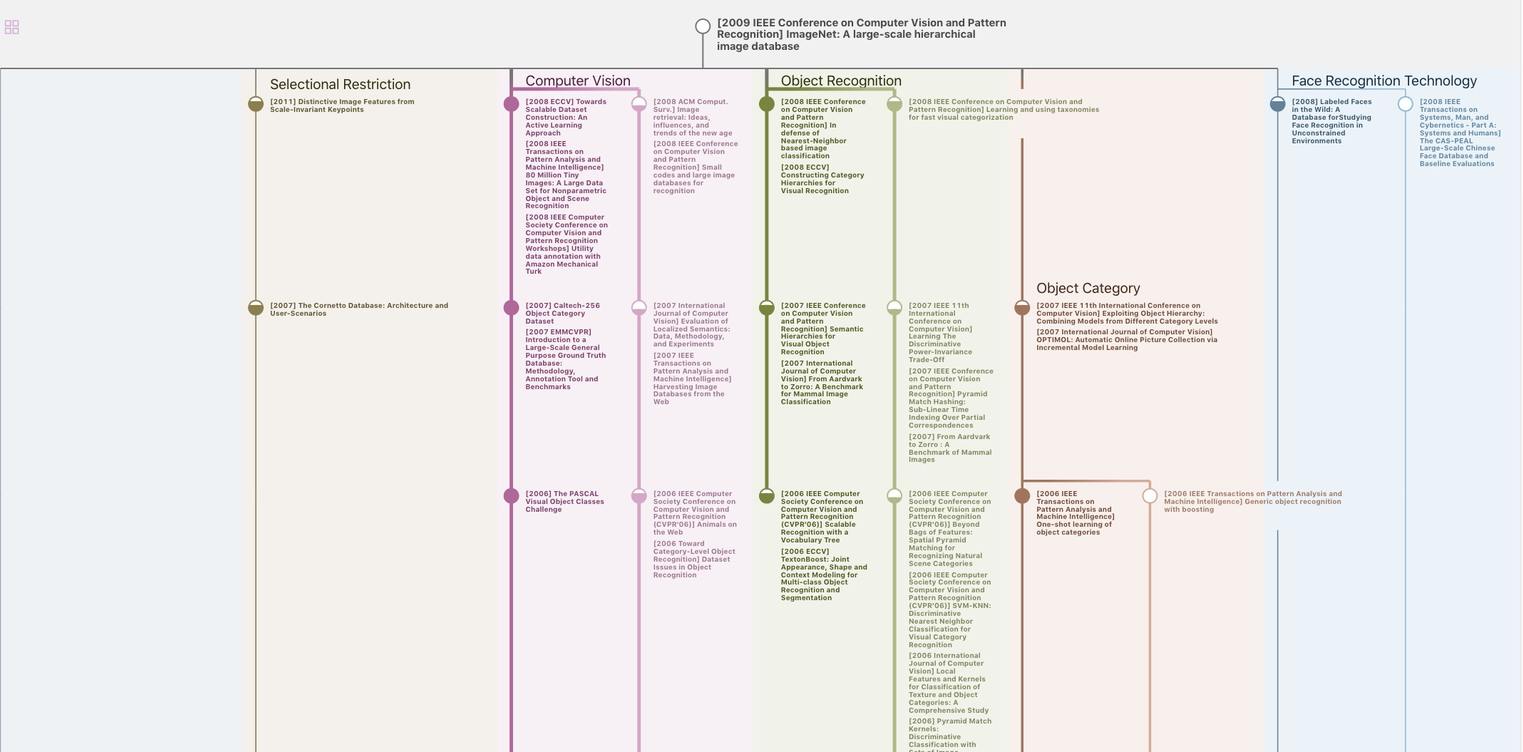
生成溯源树,研究论文发展脉络
Chat Paper
正在生成论文摘要