EEG and EMG fusion-based hand 3D Trajectory Estimation using deep learning model: A preliminary study
2023 14th International Conference on Computing Communication and Networking Technologies (ICCCNT)(2023)
摘要
Hand trajectory estimation plays an important part in various fields, such as human-computer interaction (HCI), rehabilitation robotics, and prosthetics. This study investigates the use of EEG and EMG data fusion in hand trajectory estimation, employing a dataset of five subjects and a deep learning algorithm based on Convolutional Neural Networks (CNN) and Long Short-Term Memory (LSTM). The results demonstrate a significant enhancement in hand trajectory estimation accuracy, with an average accuracy of 0.608 ± 0.031 across the database of five subjects for entire frequency range of EEG signal with EMG signal. The fusion of EEG and EMG data allows for improved spatial resolution, capturing the intention or planning of hand movements from EEG signals and precise muscle activations from EMG signals. The CNN-LSTM deep learning algorithm effectively integrates and processes these fused signals, leading to more accurate and reliable hand trajectory estimations. Furthermore, the correlation coefficient of the estimated hand trajectories with the ground truth trajectories exhibits a strong correlation of 0.6354 ± 0.030 on average across the dataset for the entire frequency range of EEG signal with EMG signal. The fusion of EEG and EMG data enables a more comprehensive understanding of the neural processes and muscle activations associated with hand movements, contributing to the accuracy and reliability of the hand trajectory estimation. These findings highlight the significance of EEG and EMG data fusion in hand trajectory estimation and its potential to improve the performance of applications such as HCI, rehabilitation robotics, and prosthetics.
更多查看译文
关键词
Deep learning,EEG,EMG,Estimation,Data Fusion
AI 理解论文
溯源树
样例
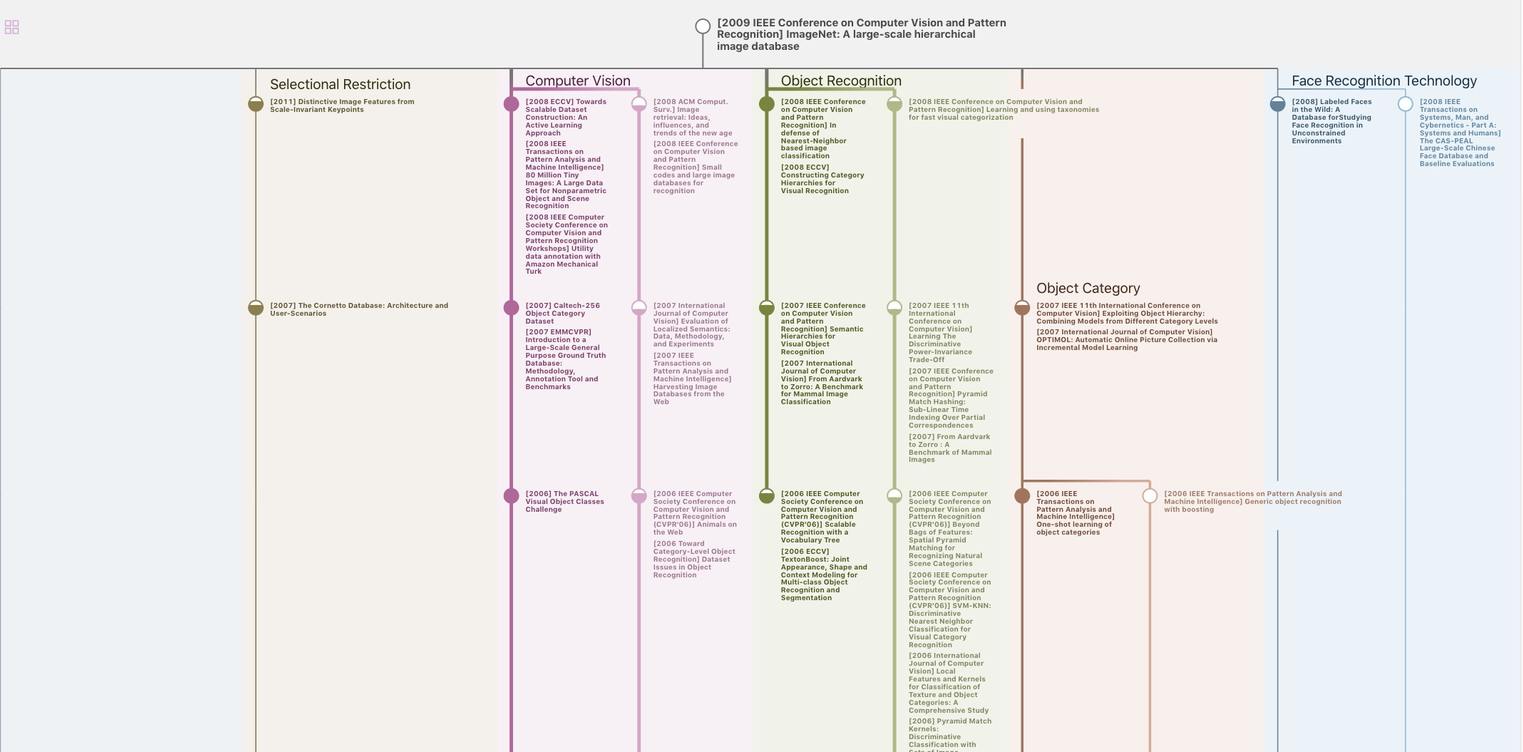
生成溯源树,研究论文发展脉络
Chat Paper
正在生成论文摘要