Improving the Performance of Epileptic Seizure Prediction using Supervised Clustering of Deep Features
2023 14th International Conference on Computing Communication and Networking Technologies (ICCCNT)(2023)
摘要
Epileptic seizures impact over 65 million people worldwide. Predicting epilepsy will greatly improve patient confidence and overall quality of life by enabling timely intervention and reducing the risk of fatal injuries. While current state-of-the-art systems use electroencephalography (EEG) as their primary input, EEG is susceptible to artefacts and may not be suitable for ambulatory home care devices. In this paper, we explore the development of a seizure prediction system that utilizes electrocardiography (ECG) as its input, offering the potential for integration into ambulatory devices. In situations where a seizure cannot be avoided, having a family member or friend record a video during the seizure can provide valuable insights for refining the treatment plan. This is very important from a neurologist’s perspective to characterise the seizure.In this paper, we develop a convolutional neural network-based seizure detection system, and subsequently, we extend it for seizure prediction. The baseline detection system gave an area under the receiver operating characteristics curve (AUROC) score of 0.63 and an area under the detection error trade-off curve (AUDET) score of 0.3220. We used supervised clustering of the deep features to enhance the performance of the systems. We obtained an AUROC score of 0.7238 and an AUDET score of 0.2648 in the frequency domain based-detection system. Subsequently, we developed a baseline system for epilepsy prediction in the time domain, which showed a performance of 0.7469 in AUROC and 0.2461 in AUDET. The final frequency domain-based prediction system yielded a performance of 0.8327 in AUROC and 0.1500 in AUDET, which is an improvement of 0.0858 in AUROC and a 0.0961 reduction in AUDET.
更多查看译文
关键词
Epilepsy,Seizure Prediction,ECG,CNN,Center Loss,Crossentropy Loss,Detection Error Tradeoff
AI 理解论文
溯源树
样例
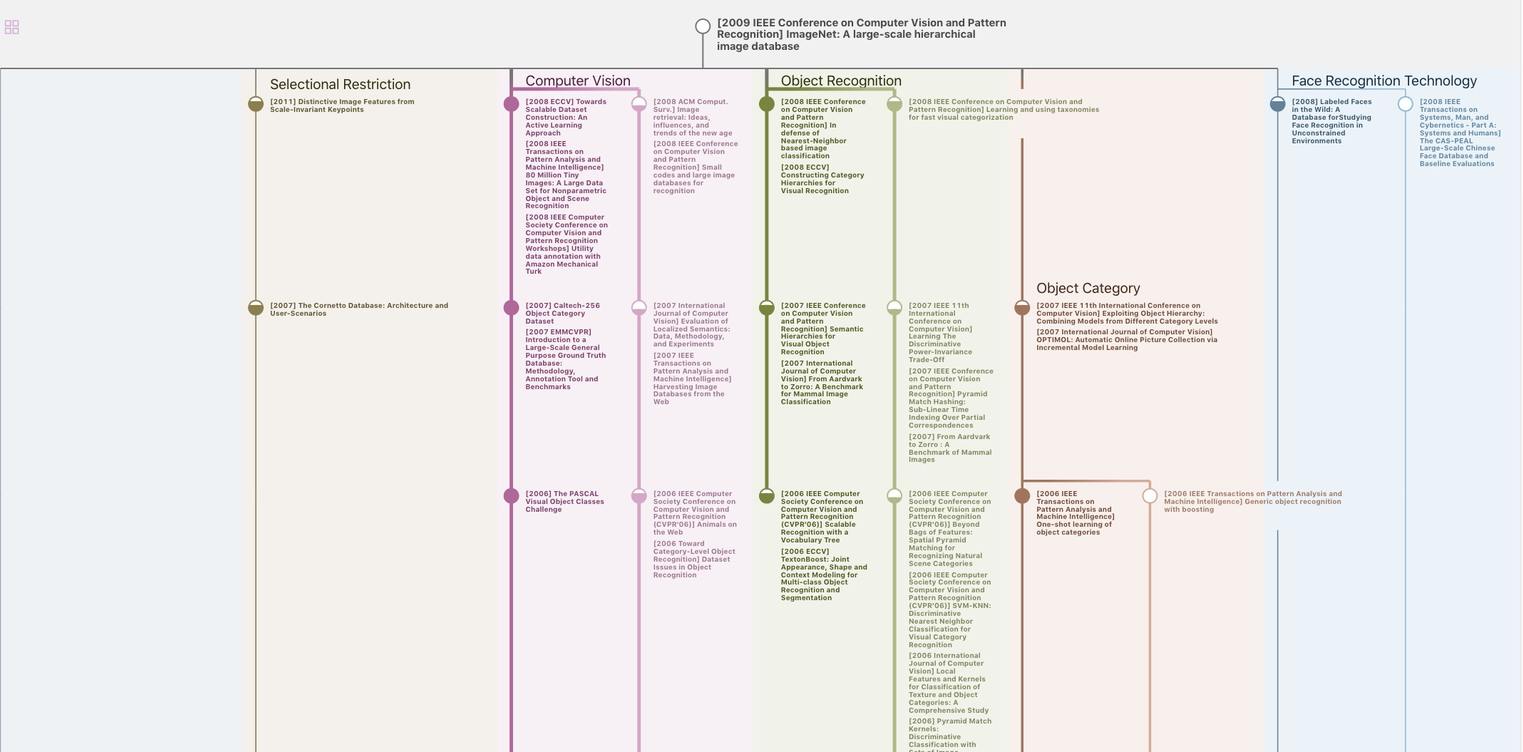
生成溯源树,研究论文发展脉络
Chat Paper
正在生成论文摘要