Machine Learning-Driven Threshold Optimization for Wavelet Packet Denoising in EEG-Based Mental State Classification
2023 14th International Conference on Computing Communication and Networking Technologies (ICCCNT)(2023)
摘要
The accurate classification of mental states from electroencephalography (EEG) data using machine learning (ML) techniques is a developing area of research. However, the presence of noise and artifacts in EEG signals poses a significant challenge to achieving accurate results. In recent years, Wavelet Transform (WT) has emerged as an effective method for denoising and analyzing non-stationary biosignal data, such as EEG. This study focuses on using Wavelet Packet Decomposition (WPD), an extension of Wavelet Transform (WT), for denoising EEG signals and evaluates its effect on classification accuracy of mental states during music listening versus no-music stimulus. In WPD-based denoising, the optimal choice of threshold level is crucial for balancing signal retention and noise reduction. EEG classification performance also depends on the choice of feature sets used to train classification models. Statistical, covariance, entropy, and wavelet-based features were extracted from EEG signals denoised at various threshold levels using WPD. We empirically evaluate the effects of different threshold levels on the classification performance of EEG feature sets using k-Nearest Neighbours (kNN) classifier. Our findings show that the choice of denoising parameters and feature sets are interlinked, and by optimally choosing a feature set and corresponding EEG signal denoising threshold, maximal classification performance can be achieved. Using covariance features, aggressive signal denoising, and the kNN Classifier, maximal classification accuracy is achieved.
更多查看译文
关键词
Electroencephalography,wavelet packet decomposition,denoising,threshold selection,classification
AI 理解论文
溯源树
样例
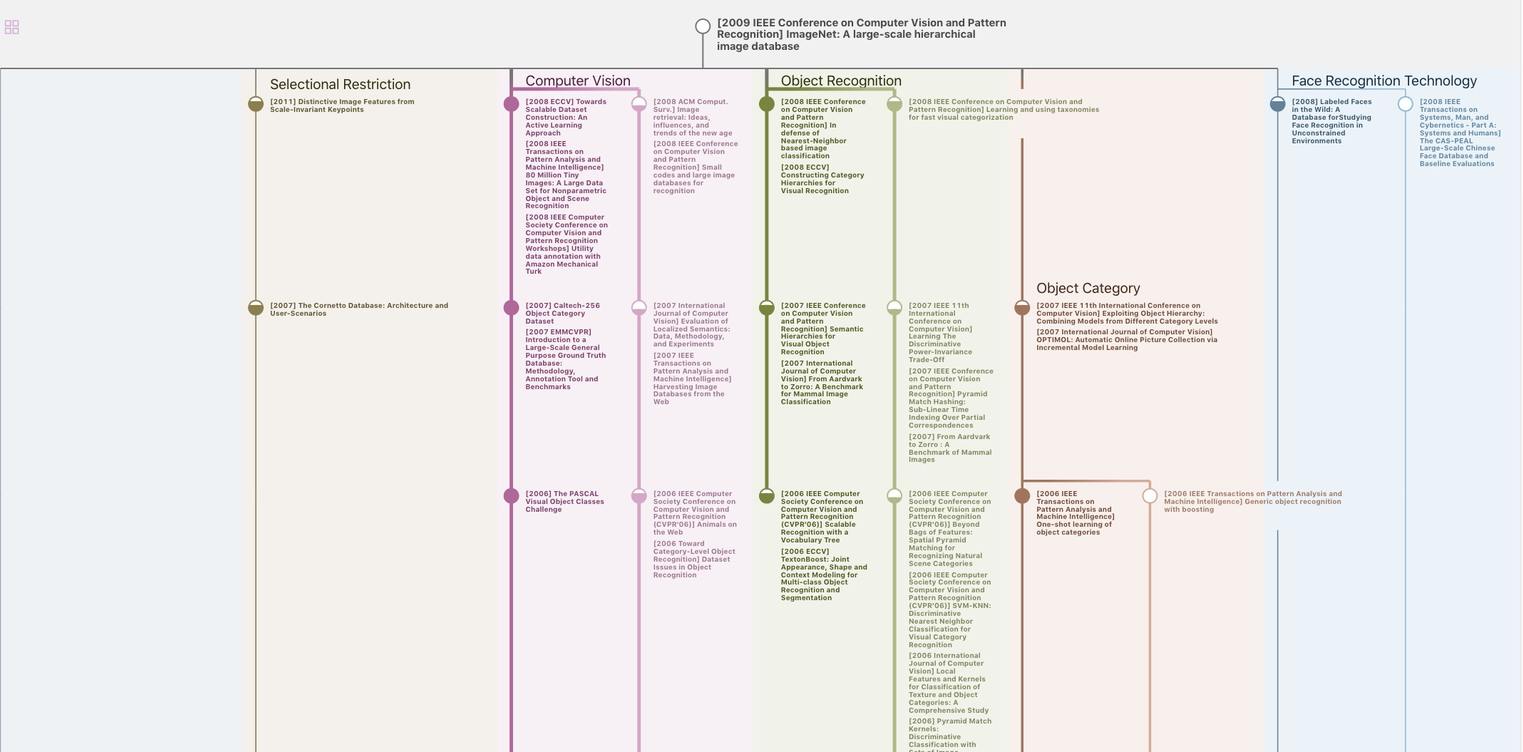
生成溯源树,研究论文发展脉络
Chat Paper
正在生成论文摘要