GAFormer: Wearable IMU-Based Human Activity Recognition with Gramian Angular Field and Transformer
2023 ASIA PACIFIC SIGNAL AND INFORMATION PROCESSING ASSOCIATION ANNUAL SUMMIT AND CONFERENCE, APSIPA ASC(2023)
摘要
Recognizing human activities (HAR) from wearable motion sensors has widely practical applications due to its low cost, convenient use, and scalability. Plenty of works utilize 1D CNN or RNN to capture temporal information from time series, while others take advantage of 2D CNN architectures that can effectively handle spatial correlation, and distinctive recognition features, which are really useful for recognition tasks. This paper proposes GAFormer, a method, which exploits the latter, for human activity recognition from wearable motion sensors. GAFormer transforms the raw IMU data into a Gramian Angular Difference Field (GADF) image, which encodes the pair-wise angles between different sensor readings to capture the temporal dynamics and relationships among the sensor measurements. Next, a transformer model is employed to extract visual features from GADF images effectively. In addition, we adapted a state-of-the-art transformer CoAtNet as the backbone of GAFormer. GAFormer is evaluated over two published datasets C-MHAD and GesHome. With the accuracies of 98% on C-MHAD and 95.5% on GesHome's subset, GAFormer demonstrates that combining the GADF technique and a transformer model could be feasible and promising for motion sensor-based action recognition.
更多查看译文
关键词
Human activity recognition,inertial sensors,features extraction,Convolutional Neural Networks,Transformers,Gramian Angular Field
AI 理解论文
溯源树
样例
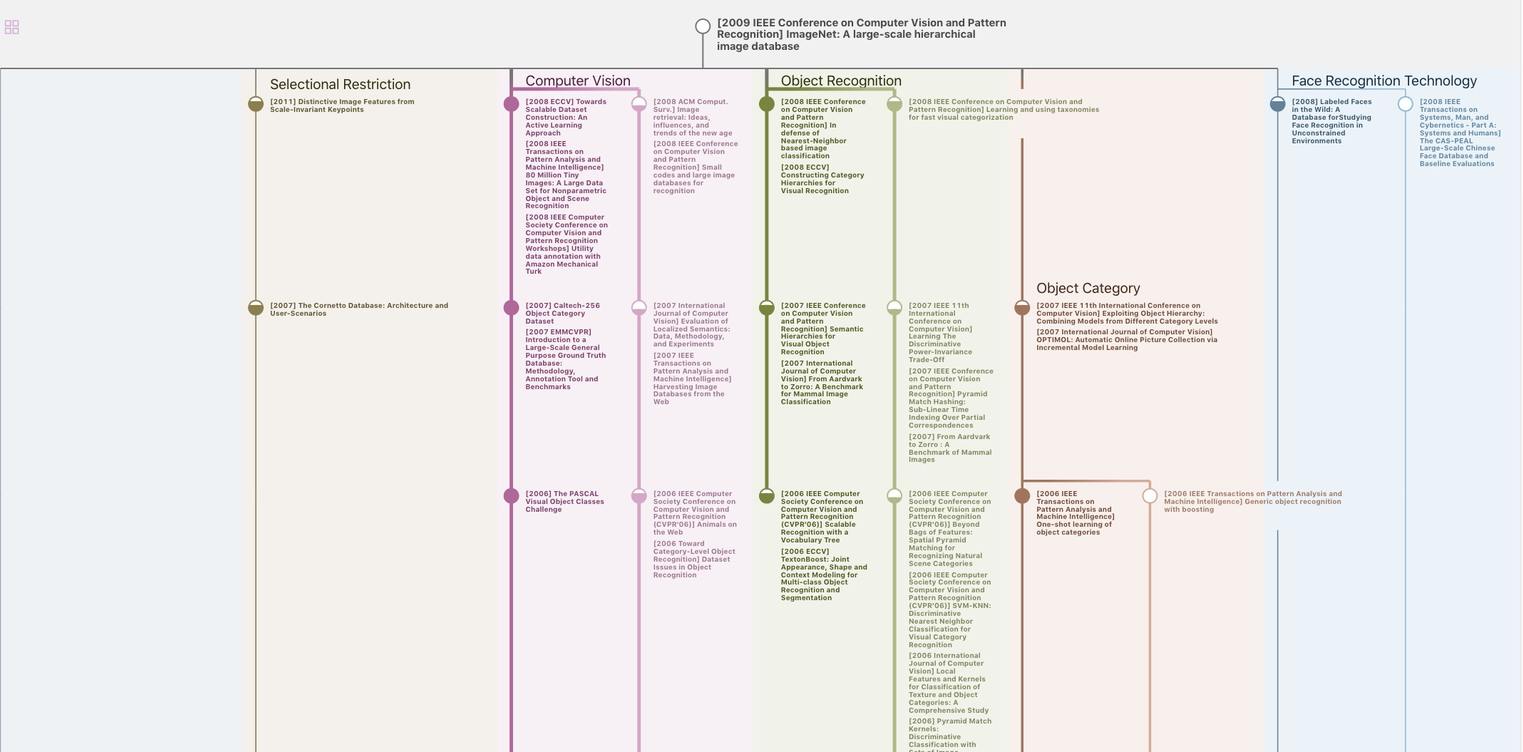
生成溯源树,研究论文发展脉络
Chat Paper
正在生成论文摘要