Exploring Residual Cepstral Features for Spoken Language Identification
2023 ASIA PACIFIC SIGNAL AND INFORMATION PROCESSING ASSOCIATION ANNUAL SUMMIT AND CONFERENCE, APSIPA ASC(2023)
摘要
In this paper, we introduce a Spoken Language Identification (SLI) system, which uses Linear Prediction (LP) residual, decomposed with linear filterbank and followed by log and Discrete Cosine Transform (DCT). This feature set is called Linear Frequency Residual Cepstral Coefficients (LFRCC). Mel Frequency Cepstral Coefficients (MFCC) and Linear Frequency Cepstral Coefficients (LFCC) were also extracted and kept as baseline features. The Attentive Time Delay Neural Network (Attentive - TDNN) was employed to evaluate the efficiency, relevancy, and sufficiency of said LFRCC features. For experiments, a computationally challenging VoxLingua107 dataset was used. From experiments, we achieved 9.22%, 7.8%, and 6.86% EER for MFCC, LFCC, and LFRCC, respectively. Significant improvements in accuracies and equal error rate (EER) were observed when comparing the performance of MFCC and LFCC with data fusion strategies. The feature-level fusion of MFCC and LFRCC resulted in a notable enhancement of 3.16%, 2.12%, and 1.16%, accuracies compared to other combinations. Moreover, each combination of feature sets in score-level fusion has outperformed LFRCC and baselines. This paper provides a significant study on how prosodic information provided by LP residual contributes to language-specific information. We present the pitch strength analysis to understand better the contribution of LP residual in capturing language-specific information.
更多查看译文
AI 理解论文
溯源树
样例
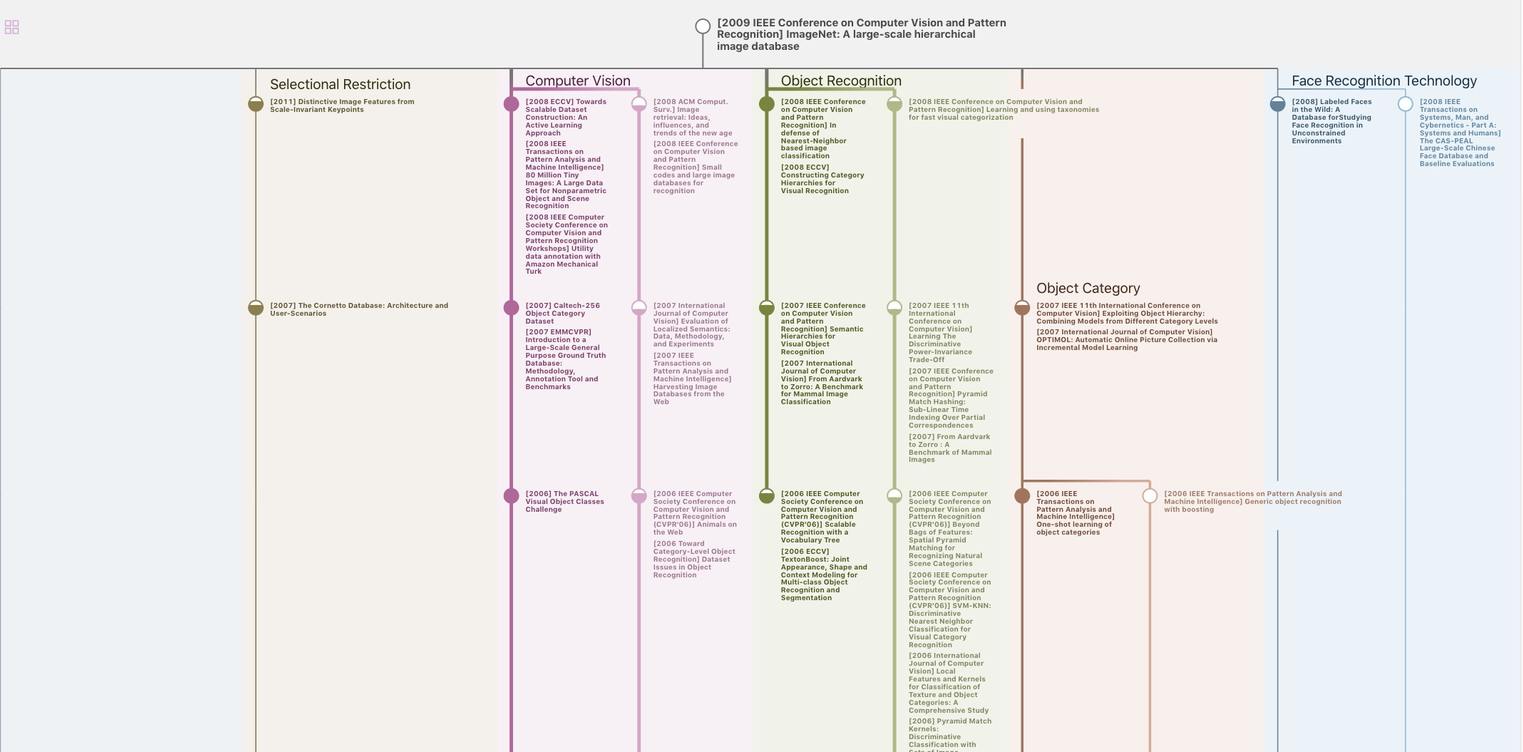
生成溯源树,研究论文发展脉络
Chat Paper
正在生成论文摘要