Adversarial Robustness of Mel Based Speaker Recognition Systems
2023 ASIA PACIFIC SIGNAL AND INFORMATION PROCESSING ASSOCIATION ANNUAL SUMMIT AND CONFERENCE, APSIPA ASC(2023)
摘要
Convolutional neural networks (CNN) applied to Mel spectrograms now have a dominant presence in the landscape of speaker recognition systems. Correspondingly, it is also important to evaluate their robustness to adversarial attacks that remains not thoroughly explored for end-to-end trained CNNs for speaker recognition. Our work addresses this gap and investigates variations of the iterative Fast Gradient Sign Method (FGSM) to perform adversarial attacks. We observe that a vanilla iterative FGSM can flip the identity of each speaker sample to that of every other speaker in the LibriSpeech dataset. Furthermore, we propose adversarial attacks specific to Mel spectrogram features by (a) limiting the number of pixels attacked, (b) restricting changes to specific frequency bands, (c) restricting changes to particular time duration, and (d) using a substitute model to craft the adversarial sample. Using thorough qualitative and quantitative results, we demonstrate the fragility and non-intuitive nature of the current CNN-based speaker recognition systems, where the predicted speaker identities can be flipped without any perceptible changes in the audio. The samples are available at "https://advdemo.github.io/speech/"
更多查看译文
AI 理解论文
溯源树
样例
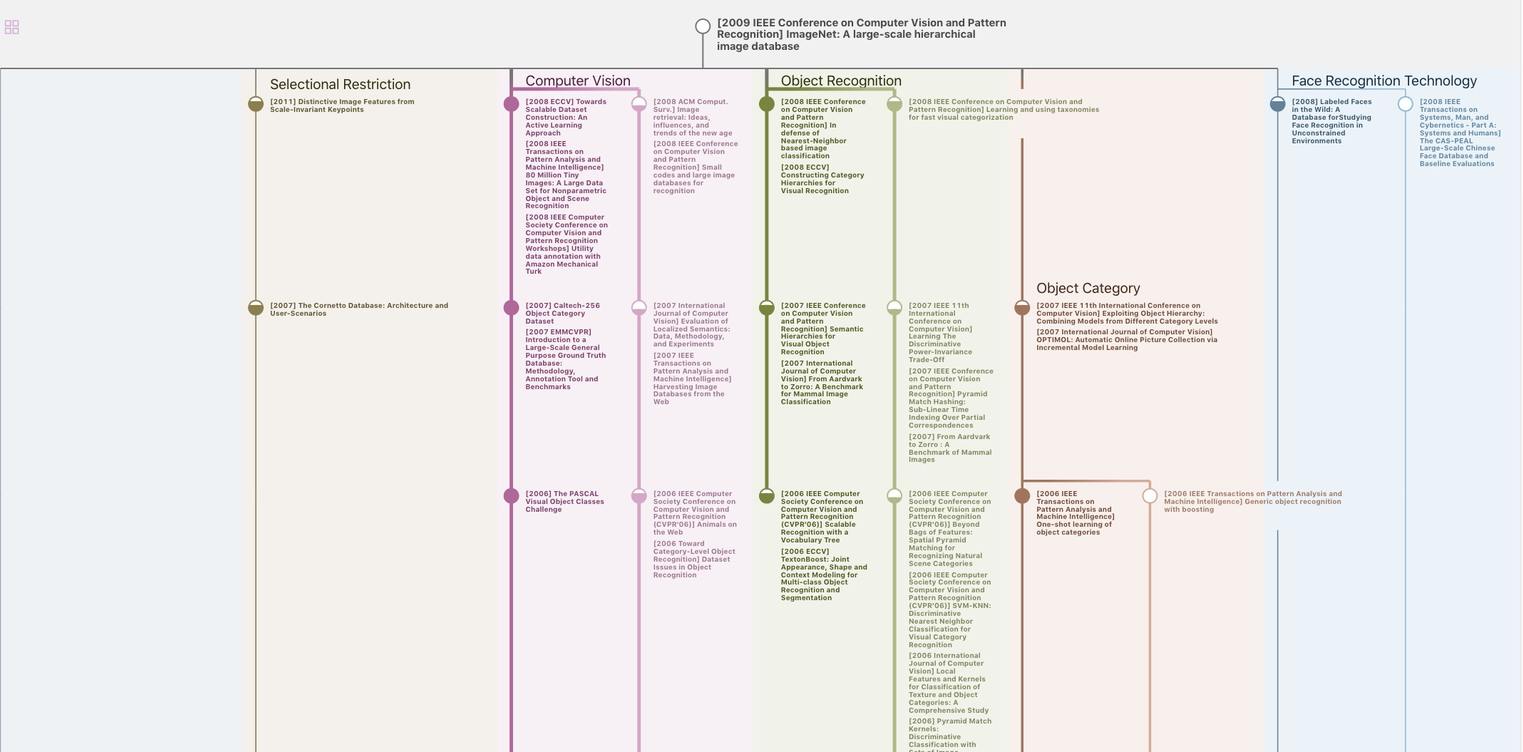
生成溯源树,研究论文发展脉络
Chat Paper
正在生成论文摘要