Function-level Vulnerability Detection Through Fusing Multi-Modal Knowledge
2023 38TH IEEE/ACM INTERNATIONAL CONFERENCE ON AUTOMATED SOFTWARE ENGINEERING, ASE(2023)
摘要
Software vulnerabilities damage the functionality of software systems. Recently, many deep learning-based approaches have been proposed to detect vulnerabilities at the function level by using one or a few different modalities (e.g., text representation, graph-based representation) of the function and have achieved promising performance. However, some of these existing studies have not completely leveraged these diverse modalities, particularly the underutilized image modality, and the others using images to represent functions for vulnerability detection have not made adequate use of the significant graph structure underlying the images. In this paper, we propose MVulD, a multi-modal-based function-level vulnerability detection approach, which utilizes multi-modal features of the function (i.e., text representation, graph representation, and image representation) to detect vulnerabilities. Specifically, MVulD utilizes a pre-trained model (i.e., UniXcoder) to learn the semantic information of the textual source code, employs the graph neural network to distill graph-based representation, and makes use of computer vision techniques to obtain the image representation while retaining the graph structure of the function. We conducted a large-scale experiment on 25,816 functions. The experimental results show that MVulD improves four state-of-the-art baselines by 30.8%-81.3%, 12.8%-27.4%, 48.8%-115%, and 22.9%-141% in terms of F1-score, Accuracy, Precision, and PR-AUC respectively.
更多查看译文
关键词
Vulnerability Detection,Computer Vision,Deep Learning,Multi-Modal Code Representations
AI 理解论文
溯源树
样例
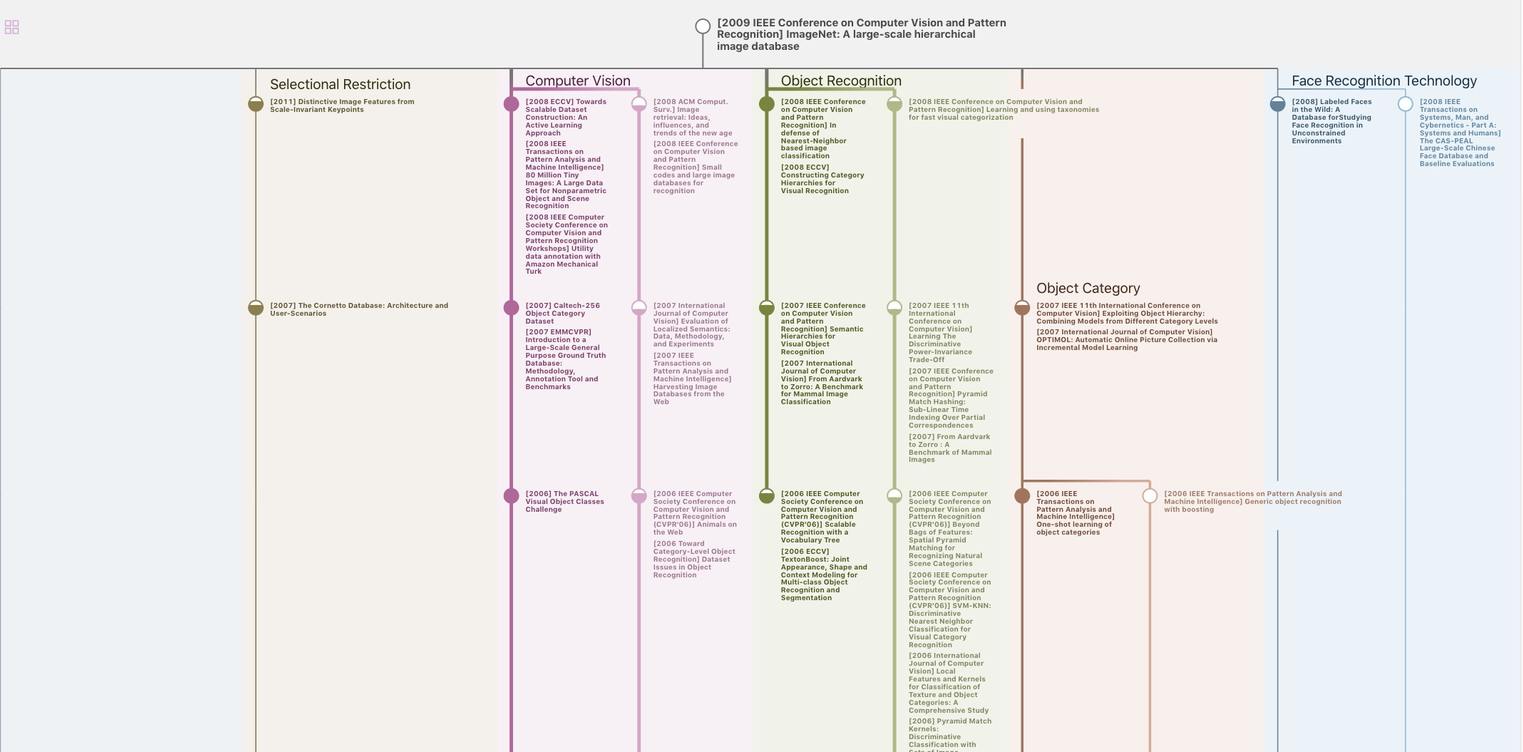
生成溯源树,研究论文发展脉络
Chat Paper
正在生成论文摘要