Attention-Based Randomized Ensemble Multi-Agent Q-Learning
2023 23rd International Conference on Control, Automation and Systems (ICCAS)(2023)
摘要
Cooperative multi-agent scenarios are prevalent in real-world applications. Optimal coordination of agents requires appropriate task allocation, considering each task's complexity and each agent's capability. This becomes challenging under decentralization and partial observability, as agents must self-allocate tasks using limited state information. We introduce a novel multi-agent environment in which effective sub-task assignment is crucial for high-scoring performance. In addition, we propose a new multi-agent reinforcement learning framework named as attention-based randomized ensemble multi-agent Q-learning, or AREQ for short. This approach integrates a unique network structure using a multi-head attention mechanism, efficiently extracting task-related information from observations. AREQ also incorporates a randomized ensemble method, enhancing sample efficiency. We explore the impact of this attention-based structure and the random ensemble method through an ablation study and show AREQ's superiority compared to existing MARL methods within our proposed environment.
更多查看译文
关键词
Multi-agent reinforcement learning,Attention,Ensemble network
AI 理解论文
溯源树
样例
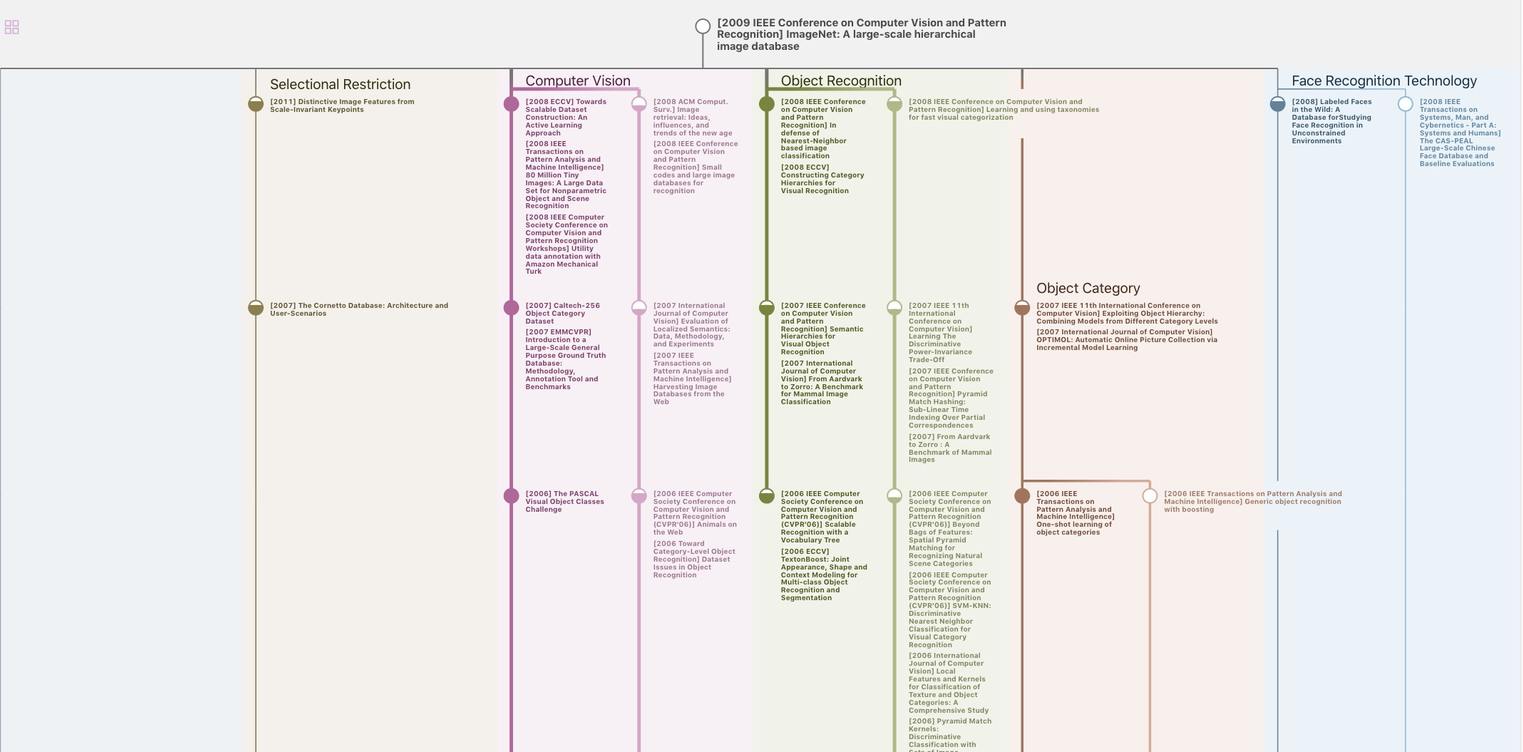
生成溯源树,研究论文发展脉络
Chat Paper
正在生成论文摘要