Adversarial Network Pruning by Filter Robustness Estimation
ICASSP 2023 - 2023 IEEE International Conference on Acoustics, Speech and Signal Processing (ICASSP)(2023)
摘要
Network pruning has been extensively studied in model compression to reduce neural networks’ memory, latency, and computation cost. However, the pruned networks still suffer from the threat posed by adversarial examples, limiting the broader application of the pruned networks in safety-critical applications. Previous studies maintain the robustness of the pruned networks by combining adversarial training and network pruning but ignore preserving the robustness at a high sparsity ratio in structured pruning. To address such a problem, we propose an effective filter importance criterion, Filter Robustness Estimation (FRE), to evaluate the importance of filters by estimating their contribution to the adversarial training loss. Empirical results show that our FRE-based Robustness-aware Filter Pruning (FRFP) outperforms the state-of-the-art methods by 12.19%∼37.01% of empirical robust accuracy on the CIFAR10 dataset with the VGG16 network at an extreme pruning ratio of 90%.
更多查看译文
关键词
Neural network pruning,structured pruning,adversarial training,adversarial example
AI 理解论文
溯源树
样例
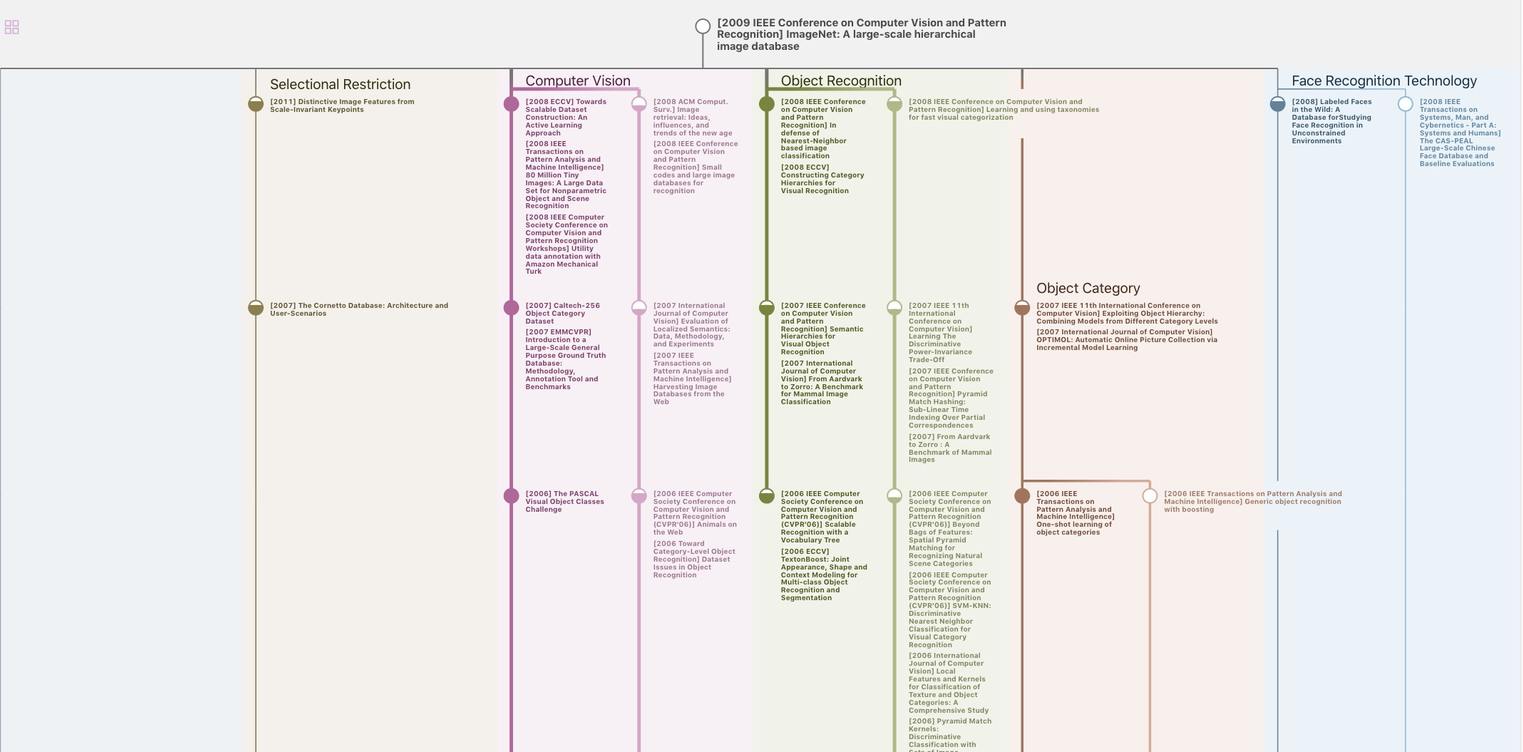
生成溯源树,研究论文发展脉络
Chat Paper
正在生成论文摘要