Independent Vector Analysis with Multivariate Gaussian Model: a Scalable Method by Multilinear Regression
ICASSP 2023 - 2023 IEEE International Conference on Acoustics, Speech and Signal Processing (ICASSP)(2023)
摘要
Joint blind source separation (JBSS) is a powerful tool for analyzing multiple linked datasets, distinguished by the key ability to exploit cross-dataset dependencies. Despite this ability generally improving overall estimation performance, joint decompositions also incur considerable computational costs, which can lead to intractable problems with hundreds or thousands of datasets. In this paper, we introduce an efficient method for large-scale JBSS by multilinear regression. We consider a model where out of all datasets, only a selected subset are first decomposed to provide regressors that sufficiently estimate sources across all datasets. These regressors define a per-source cost function that naturally extends independent vector analysis (IVA) with a multivariate Gaussian source prior (IVA-G), a powerful formulation for exploiting cross-dataset dependencies. Using simulated and real fMRI data, we demonstrate significant advantages of this method compared with other JBSS methods.
更多查看译文
关键词
Joint Blind Source Separation,Independent Vector Analysis,Group Independent Component Analysis
AI 理解论文
溯源树
样例
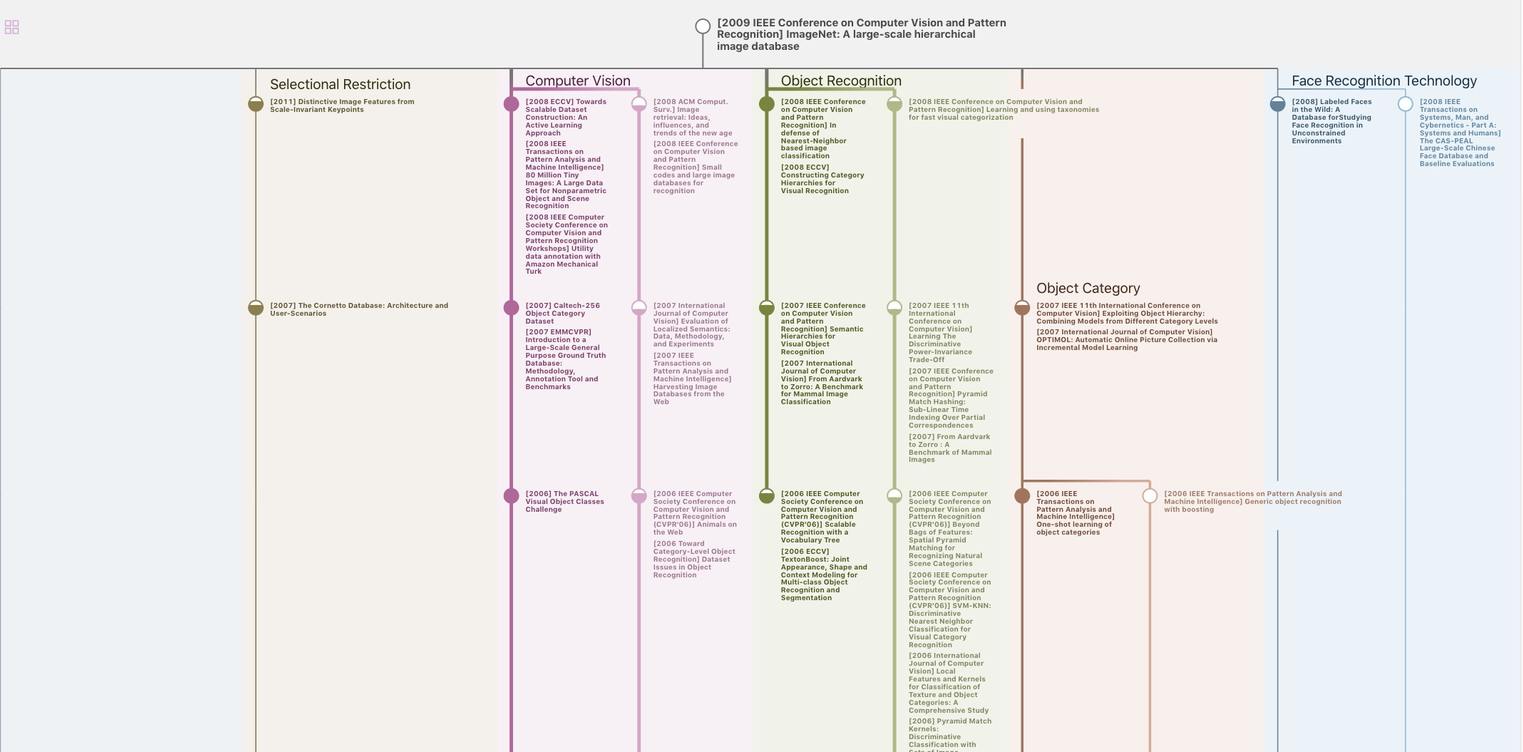
生成溯源树,研究论文发展脉络
Chat Paper
正在生成论文摘要