GOP-Based Latent Refinement for Learned Video Coding
ICASSP 2023 - 2023 IEEE International Conference on Acoustics, Speech and Signal Processing (ICASSP)(2023)
摘要
This paper presents a method allowing learned video encoders to apply arbitrary latent refinement strategies to serve as RateDistortion Optimization (RDO) at the time of encoding. To do so, a latent domain search is applied on an initial latent representation of the video signal. This search is implemented as a set of iterations, each of which performs a gradient descent with back-propagation of error defined by a Lagrangian RD cost. This cost function is intentionally chosen to be the same as the cost function that was used during the end-to-end model training, except that instead of updating model weights, each iteration fine-tunes the latent representation itself. Moreover, a temporal look-ahead is integrated in the cost function of I and P frames to take into account the cascade effect of their latent fine-tuning on subsequent frames in the Group of Pictures (GOP). The experiments show that the proposed latent space RDO method can improve by 11.6% and 9.4% in terms of BD-BR coding efficiency in Random-Access (RA) and All-Intra (AI) configurations, when applied on top a high-performance opensource end-to-end codec.
更多查看译文
关键词
Learned Video Coding,Rate-Distortion Optimization,Back-propagation with gradient decent
AI 理解论文
溯源树
样例
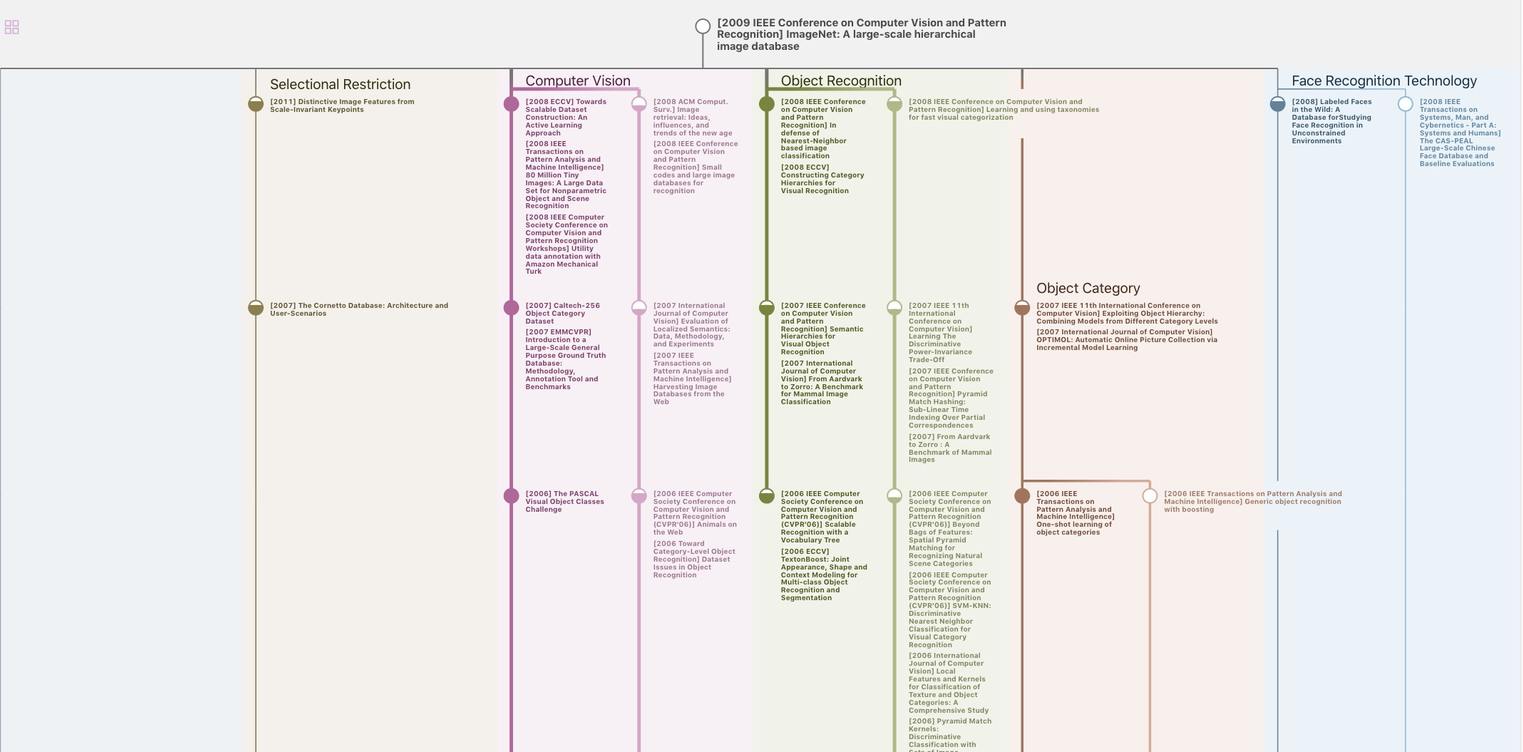
生成溯源树,研究论文发展脉络
Chat Paper
正在生成论文摘要