Meta-Learning for Image-Guided Millimeter-Wave Beam Selection in Unseen Environments
ICASSP 2023 - 2023 IEEE International Conference on Acoustics, Speech and Signal Processing (ICASSP)(2023)
摘要
The use of alternate modalities, like images, for fast beamforming in the millimeter wave (mmWave)-band is being proposed to ensure high bandwidth connectivity in vehicular scenarios typically seen in the context of autonomous cars. Considering the dynamic deployment conditions, a car may encounter new environments which were not explicitly included in an apriori training dataset. In this paper, we propose to use the Model-Agnostic Meta-Learning (MAML) framework on the image data of the mmWave vehicle-to-infrastructure beam selection FLASH dataset, to overcome the generalization issues of a pre-trained model in unseen non-line-of-sight (NLOS) connectivity environments. MAML has additional advantages over traditional deep-learning techniques: (i) it uses a fraction of the data which, in turn, simplifies data collection and storage, and (ii) it results in equal or higher accuracy in optimal beam selection compared to the case when the new environment dataset is fully available during initial training. We show that our MAML implementation improves test accuracy of beam selection by up to 86% with fine-tuning when encountering an unseen NLOS environment compared to conventional supervised learning.
更多查看译文
关键词
Meta-learning,Non-RF Data,mmWave,Beam Selection
AI 理解论文
溯源树
样例
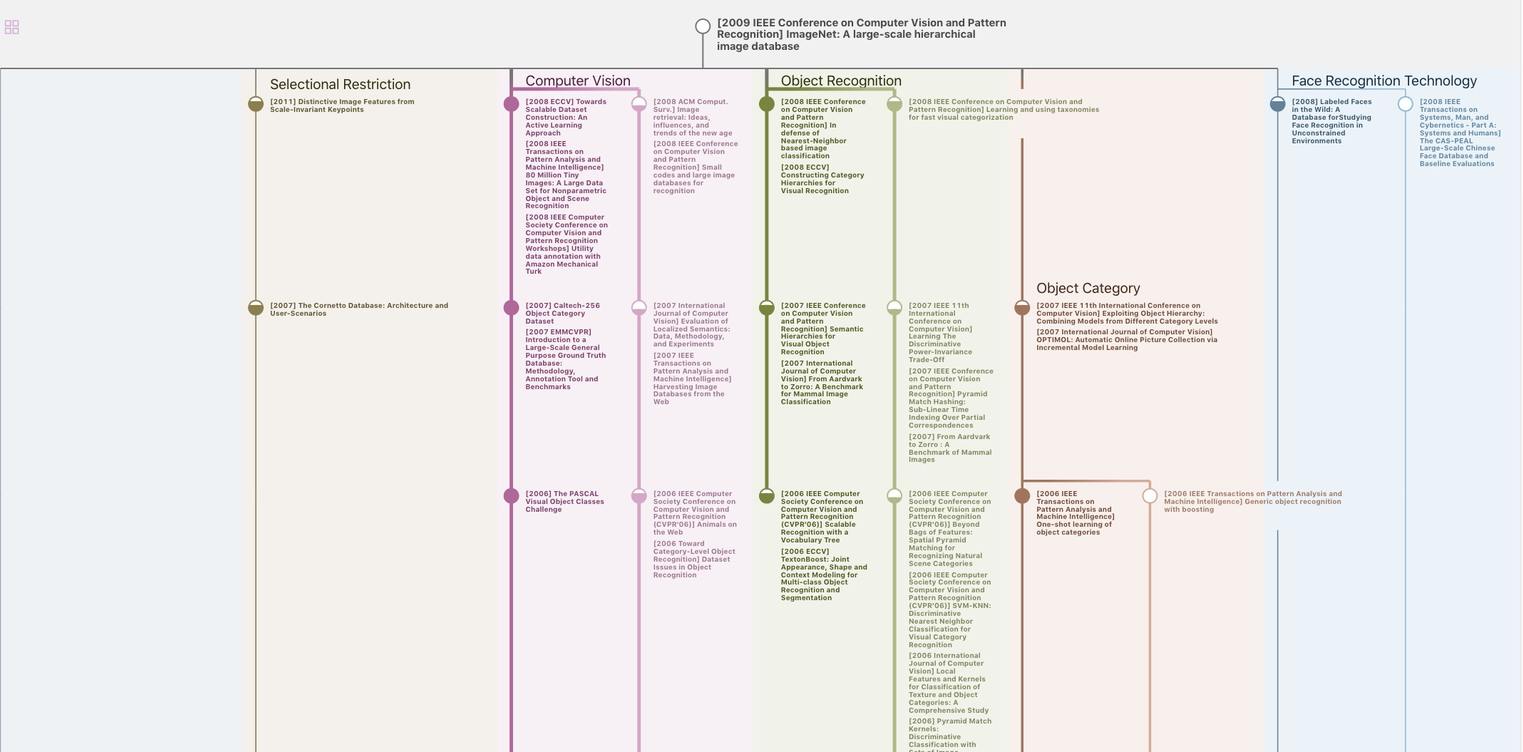
生成溯源树,研究论文发展脉络
Chat Paper
正在生成论文摘要