Relational Representation Learning for Zero-Shot Relation Extraction with Instance Prompting and Prototype Rectification
ICASSP 2023 - 2023 IEEE International Conference on Acoustics, Speech and Signal Processing (ICASSP)(2023)
摘要
Zero-shot relation extraction aims to extract novel relations that are not observed beforehand. However, existing representation methods are not pre-trained for relational representations and embeddings contain much linguistic information, the distances between them are not consistent with relational semantic similarity. In this paper, we propose a novel method based on Instance Prompting and Prototype Rectification (IPPR) to conduct relational representation learning for zeroshot relation extraction. Instance prompting is designed to reduce the gap between pre-training and fine-tuning, and guide the pre-trained model to generate relation-oriented instance representations. Prototype rectification aims to push the prototype embeddings away from each other and makes the instance embeddings closer to its corresponding prototype embeddings for dynamically rectifying the prototype embeddings. Experimental results on two public datasets demonstrate that our proposed method achieves new state-of-the-arts performance
1
.
更多查看译文
关键词
Zero-shot relation extraction,novel relations,relational representation learning
AI 理解论文
溯源树
样例
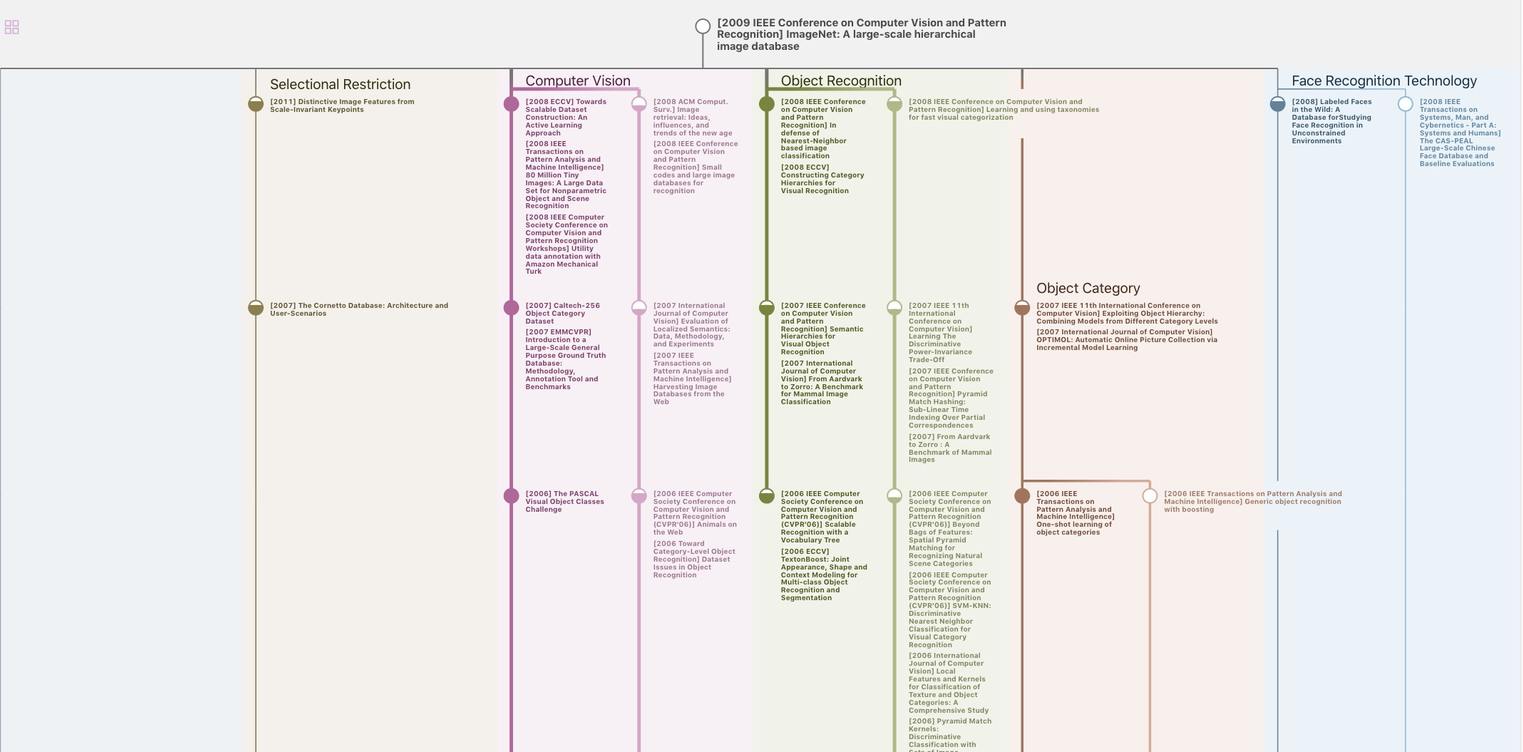
生成溯源树,研究论文发展脉络
Chat Paper
正在生成论文摘要