Improved Mask-Based Neural Beamforming for Multichannel Speech Enhancement by Snapshot Matching Masking
ICASSP 2023 - 2023 IEEE International Conference on Acoustics, Speech and Signal Processing (ICASSP)(2023)
Abstract
In multichannel speech enhancement (SE), time-frequency (T-F) mask-based neural beamforming algorithms take advantage of deep neural networks to predict T-F masks that represent speech and noise dominance. The predicted masks are subsequently leveraged to estimate the speech and noise power spectral density (PSD) matrices for computing the beamformer filter weights based on signal statistics. However, in the literature most networks are trained to estimate some pre-defined masks, e.g., the ideal binary mask (IBM) and ideal ratio mask (IRM) that lack direct connection to the PSD estimation. In this paper, we propose a new masking strategy to predict the Snapshot Matching Mask (SMM) that aims to minimize the distance between the predicted and the true signal snapshots, thereby estimating the PSD matrices in a more systematic way. Performance of SMM compared with existing IBM- and IRM-based PSD estimation for mask-based neural beamforming is presented on several datasets to demonstrate its effectiveness for the SE task.
MoreTranslated text
Key words
Speech enhancement,neural beamforming,time-frequency mask,snapshot,power spectral density
AI Read Science
Must-Reading Tree
Example
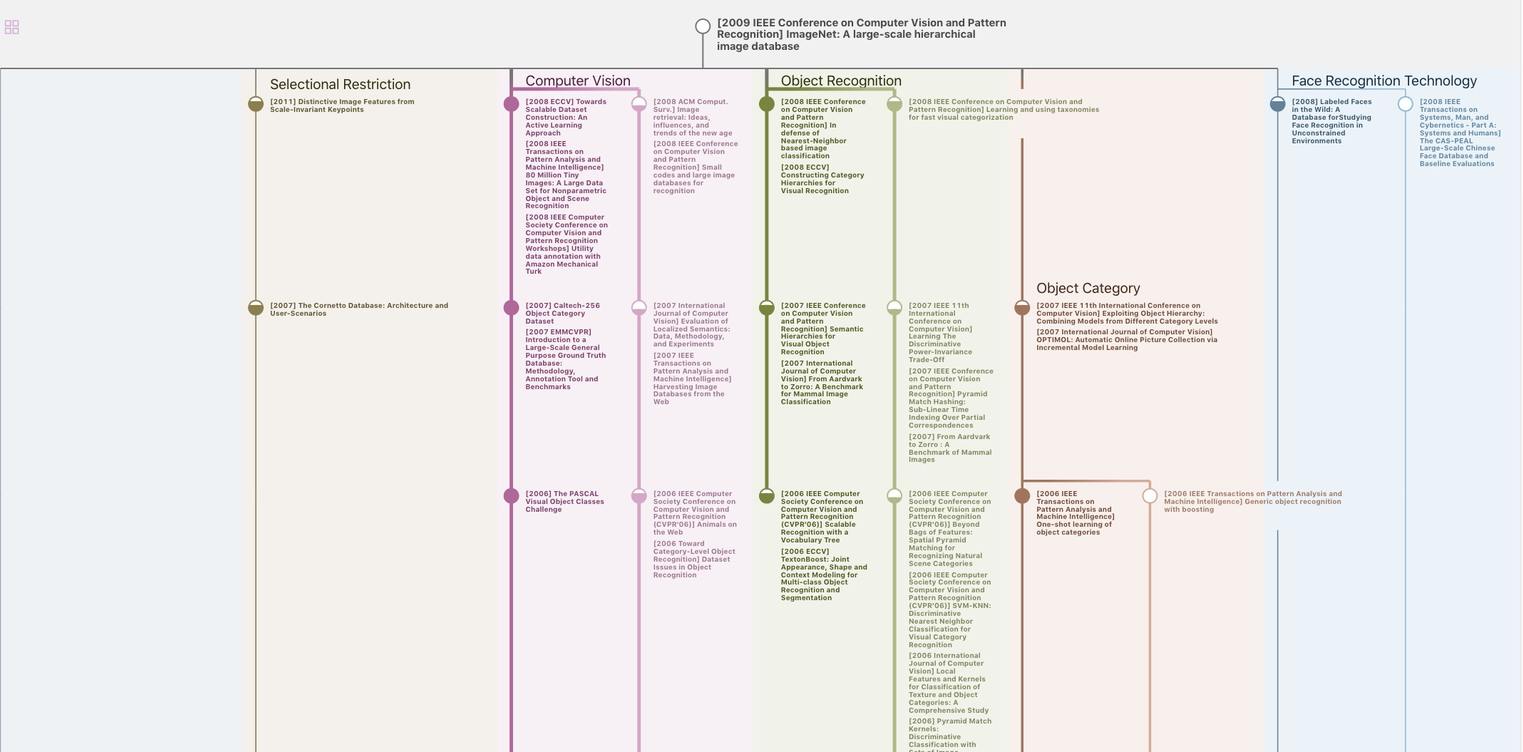
Generate MRT to find the research sequence of this paper
Chat Paper
Summary is being generated by the instructions you defined