A Lightweight Fourier Convolutional Attention Encoder for Multi-Channel Speech Enhancement
ICASSP 2023 - 2023 IEEE International Conference on Acoustics, Speech and Signal Processing (ICASSP)(2023)
摘要
Beamforming weights prediction via deep neural networks has been one of the main methods in multi-channel speech enhancement tasks. The spectral-spatial cues are crucial in beamforming weights estimation, however, many existing works fail to optimally predict the beamforming weights with an absence of adequate spectral-spatial information learning. To tackle this challenge, we propose a Fourier convolutional attention encoder (FCAE) to provide a global receptive field over the frequency axis and boost the learning of spectral contexts and cross-channel features. Besides, a new convolutional recurrent encoder-decoder (CRED) structure is proposed in this work, within which FCAEs, attention blocks with skip connections and a deep feedback sequential memory network (DFSMN) serving as recurrent module are involved. The proposed CRED structure is exploited to capture the spectral-spatial joint information to obtain accurate estimation of beamforming weights. Experimental results demonstrate the superiority of the proposed approach with only 0.74M parameters and a PESQ improvement from 2.225 to 2.359 on the ConferencingSpeech2021 challenge development test set.
更多查看译文
关键词
Multichannel speech enhancement,neural beamformer,fast fourier convolution,deep learning
AI 理解论文
溯源树
样例
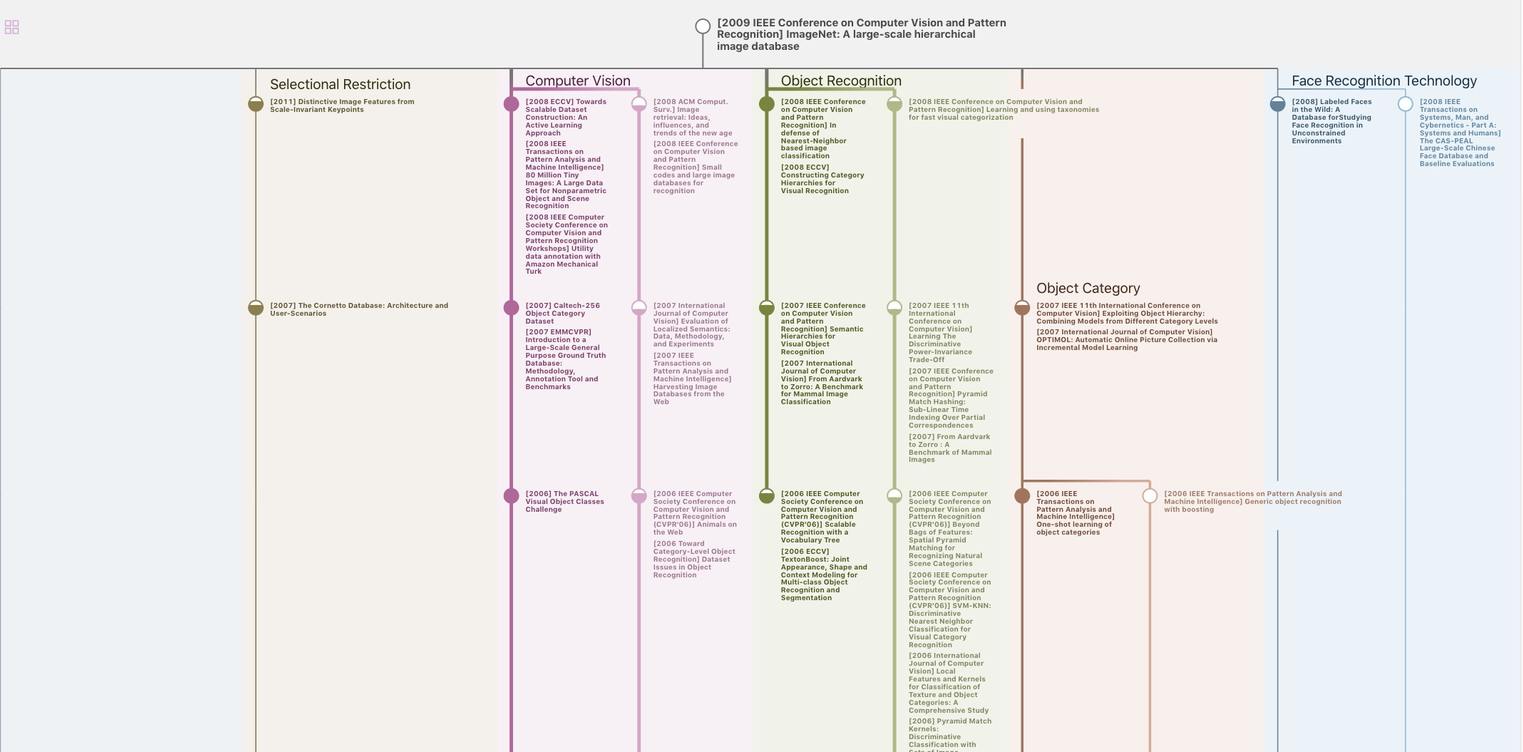
生成溯源树,研究论文发展脉络
Chat Paper
正在生成论文摘要