UNTAG: Learning Generic Features for Unsupervised Type-Agnostic Deepfake Detection
ICASSP 2023 - 2023 IEEE International Conference on Acoustics, Speech and Signal Processing (ICASSP)(2023)
摘要
This paper introduces a novel framework for unsupervised type-agnostic deepfake detection called UNTAG. Existing methods are generally trained in a supervised manner at the classification level, focusing on detecting at most two types of forgeries; thus, limiting their generalization capability across different deepfake types. To handle that, we reformulate the deepfake detection problem as a one-class classification supported by a self-supervision mechanism. Our intuition is that by estimating the distribution of real data in a discriminative feature space, deepfakes can be detected as outliers regardless of their type. UNTAG involves two sequential steps. First, deep representations are learned based on a self-supervised pretext task focusing on manipulated regions. Second, a oneclass classifier fitted on authentic image embeddings is used to detect deepfakes. The results reported on several datasets show the effectiveness of UNTAG and the relevance of the proposed new paradigm. The code is publicly available.
更多查看译文
关键词
Type-agnostic Deepfake Detection,Unsupervised classification
AI 理解论文
溯源树
样例
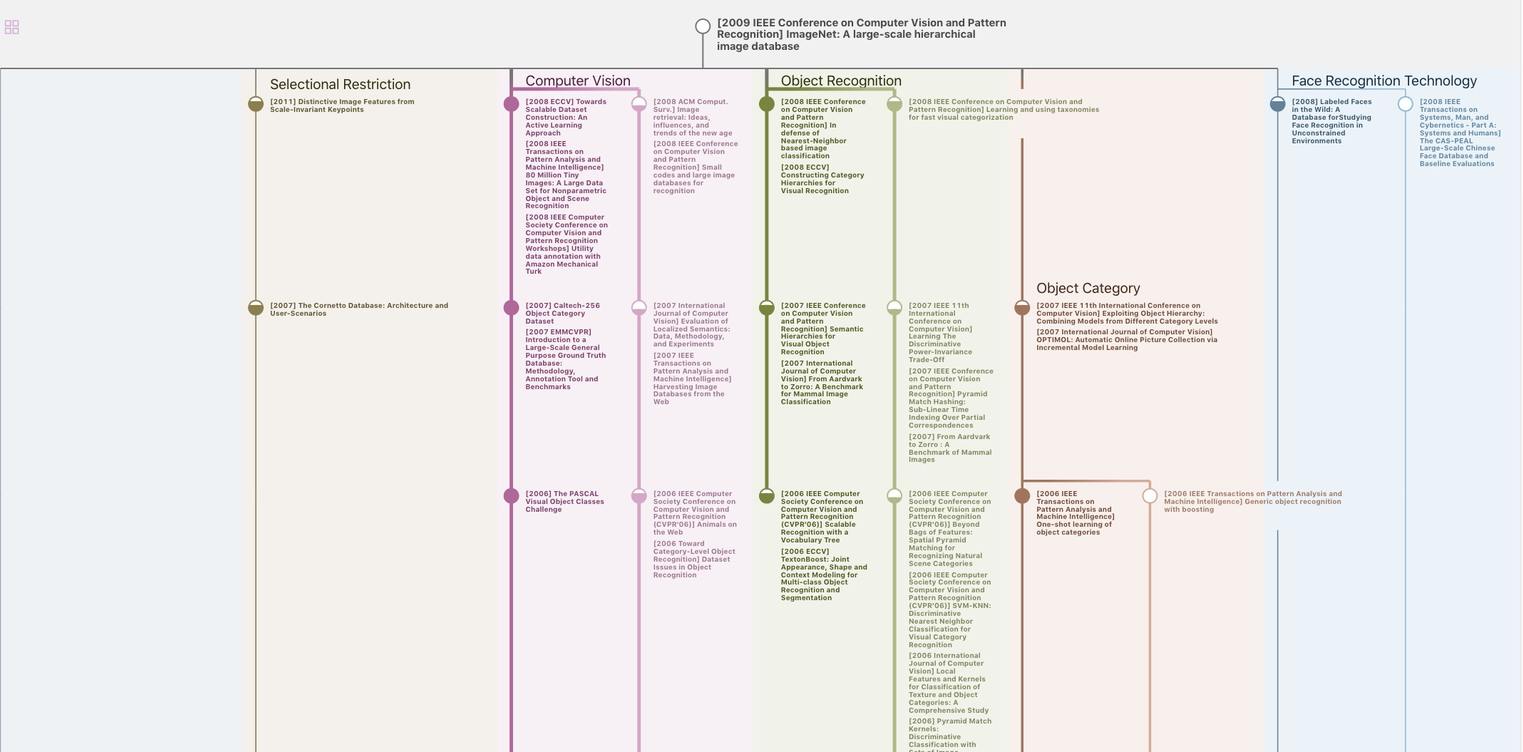
生成溯源树,研究论文发展脉络
Chat Paper
正在生成论文摘要