ECGT2T: Towards Synthesizing Twelve-Lead Electrocardiograms from Two Asynchronous Leads
ICASSP 2023 - 2023 IEEE International Conference on Acoustics, Speech and Signal Processing (ICASSP)(2023)
摘要
Electrocardiograms (ECGs) are a non-invasive measurement used to observe the condition of the heart. Modern wearable devices can record ECGs, but typically provide fewer leads–signal outputs from electrodes–than the 12 in standard electrocardiograms. Consequently, data generated from these devices may be insufficient for accurately diagnosing more complex cardiac conditions. To bridge this gap, we propose ECGT2T, a deep generative model that synthesizes ten leads from an asynchronous Lead I and Lead II input to simulate a 12-lead ECG. Compared to the R-peaks of the original wave-forms, the generated signals had timing and amplitude errors under 15 milliseconds and 10%, respectively. Experiments on two widely used ECG datasets show classifiers trained on synthesized 12-lead electrocardiograms generated with ECGT2T outperforms models trained on one- or two-lead ECGs in detecting myocardial infarction and arrhythmia.
更多查看译文
关键词
Electrocardiograms,Healthcare,Wearable,Deep learning,Generative adversarial networks
AI 理解论文
溯源树
样例
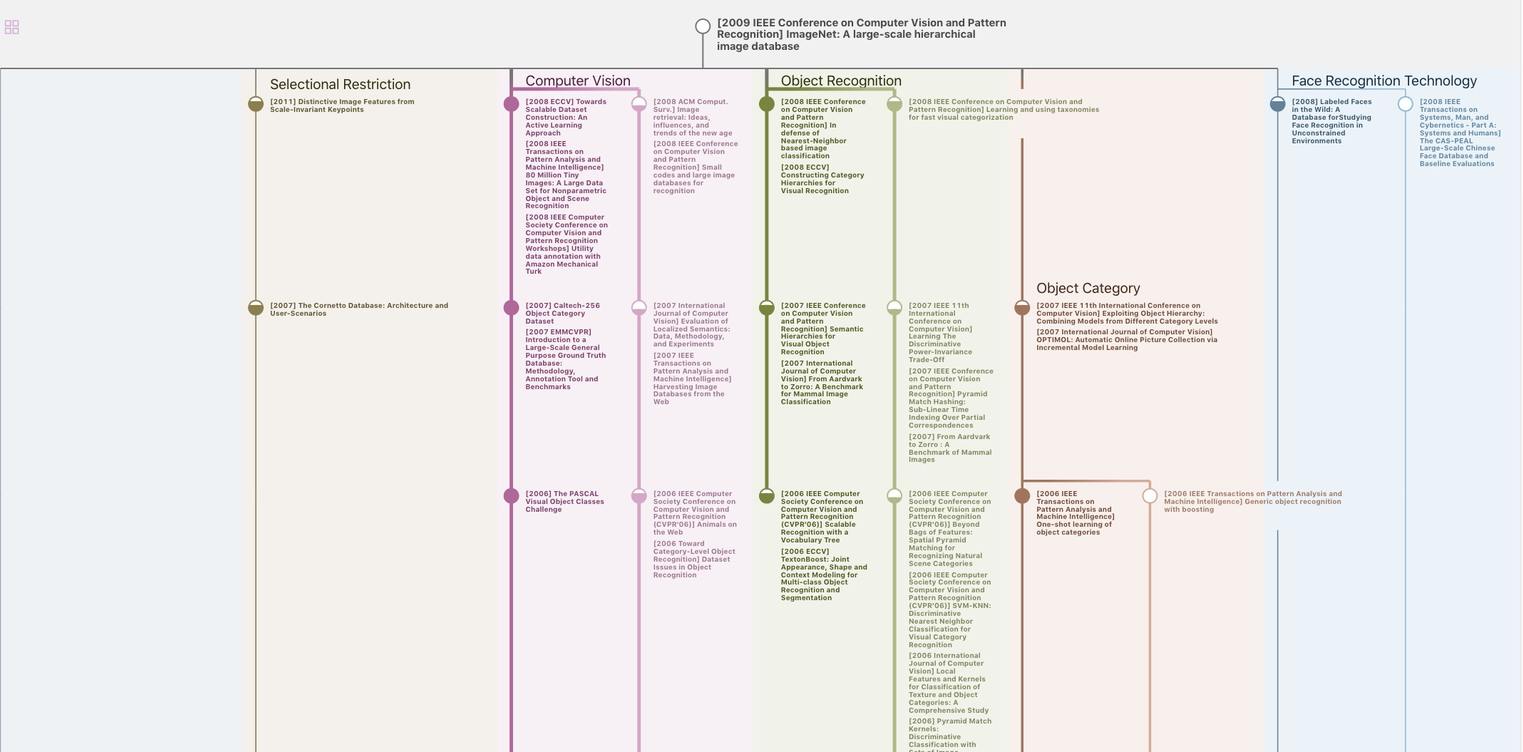
生成溯源树,研究论文发展脉络
Chat Paper
正在生成论文摘要