Stay In The Middle: A Semi-Supervised Model for CT Metal Artifact Reduction
ICASSP 2023 - 2023 IEEE International Conference on Acoustics, Speech and Signal Processing (ICASSP)(2023)
摘要
Metal artifacts degrade CT image’s quality. Recently, some deep learning-based metal artifact reduction (MAR) methods have been developed. Supervised MAR methods don’t perform well in clinical due to the domain gap between simulated and clinical data. Although this problem can be avoided in an unsupervised way, severe artifacts cannot be well suppressed. Semi-supervised MAR methods can alleviate the domain gap problem. However, the existing ones are usually accompanied by boosted model scale, which is challenging for optimization. In this paper, we propose a novel semi-supervised framework for MAR, termed SemiMAR. First, we only use one generator to learn the clean part, instead of multiple encoders and decoders disentangling artifacts. Thus, the model naturally becomes much smaller. To recover more tissue details, the advanced dual-domain MAR network knowledge is distilled into our model in both the image domain and latent feature space. Extensive experiments demonstrate the efficiency and robustness of our model.
更多查看译文
关键词
Computed Tomography,Metal Artifact Reduction,Semi-Supervised Learning,Contrastive Learning
AI 理解论文
溯源树
样例
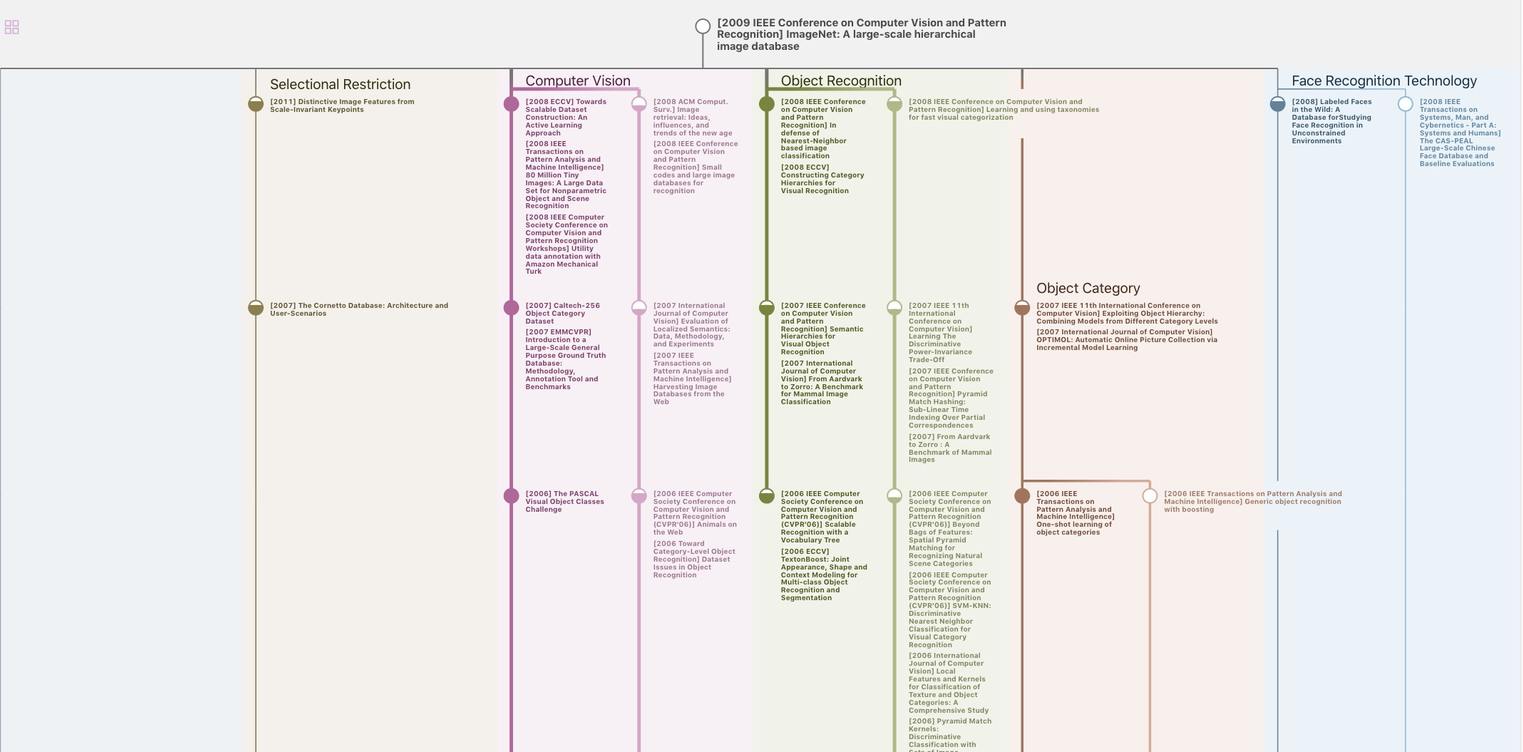
生成溯源树,研究论文发展脉络
Chat Paper
正在生成论文摘要