Self-Supervised Audio-Visual Speaker Representation with Co-Meta Learning
ICASSP 2023 - 2023 IEEE International Conference on Acoustics, Speech and Signal Processing (ICASSP)(2023)
摘要
In self-supervised speaker verification, the quality of pseudo labels determines the upper bound of its performance and it is not uncommon to end up with massive amount of unreliable pseudo labels. We observe that the complementary information in different modalities ensures a robust supervisory signal for audio and visual representation learning. This motivates us to propose an audio-visual self-supervised learning framework named Co-Meta Learning. Inspired by the Coteaching+, we design a strategy that allows the information of two modalities to be coordinated through the Update by Disagreement. Moreover, we use the idea of modelagnostic meta learning (MAML) to update the network parameters, which makes the hard samples of two modalities to be better resolved by the other modality through gradient regularization. Compared to the baseline, our proposed method achieves a 29.8%, 11.7% and 12.9% relative improvement on Vox-O, Vox-E and Vox-H trials of Voxceleb1 evaluation dataset respectively.
更多查看译文
关键词
self-supervised learning,speaker verification,audio-visual data,co-teaching+,meta-learning
AI 理解论文
溯源树
样例
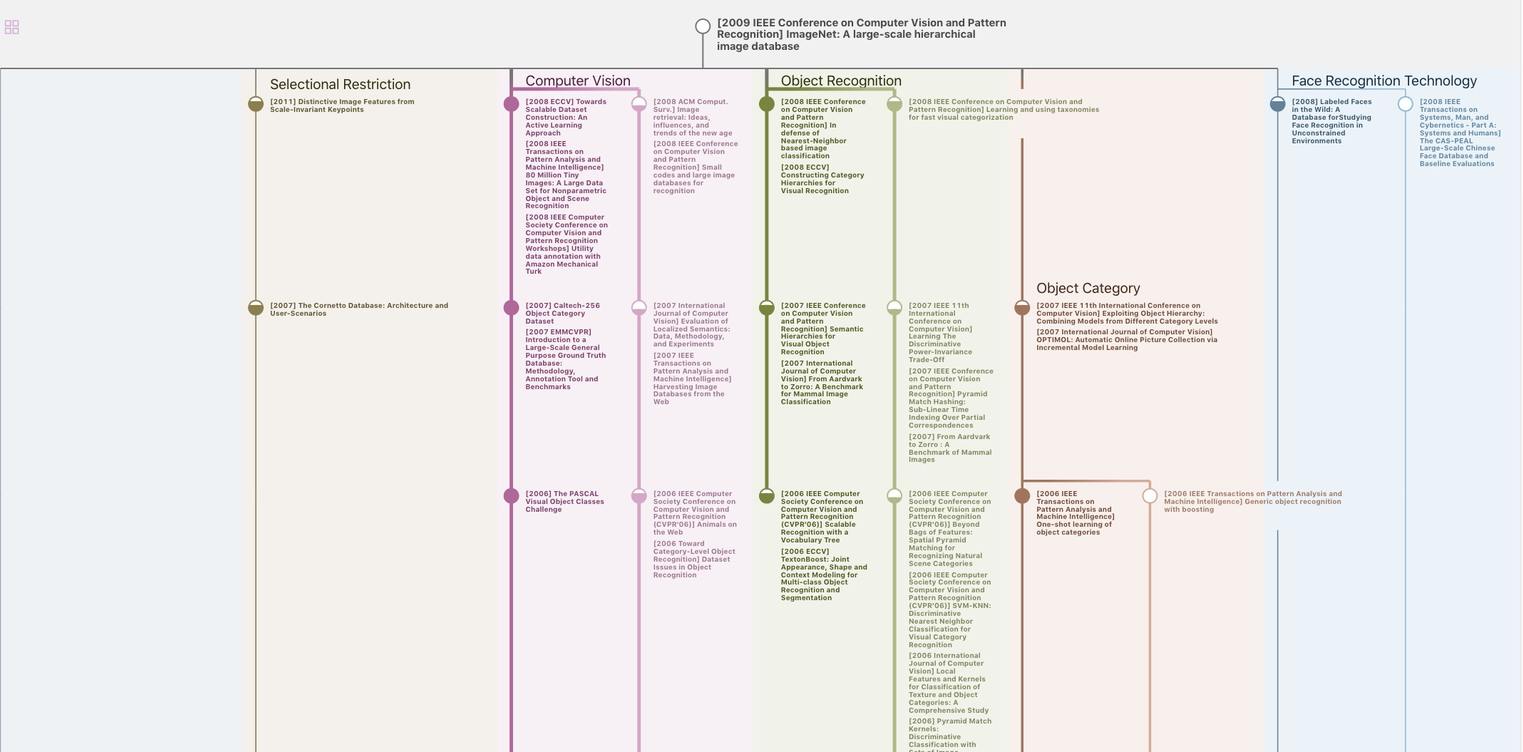
生成溯源树,研究论文发展脉络
Chat Paper
正在生成论文摘要