Newton-Based Trainable Learning Rate
ICASSP 2023 - 2023 IEEE International Conference on Acoustics, Speech and Signal Processing (ICASSP)(2023)
摘要
Selecting an appropriate learning rate for efficiently training deep neural networks is a difficult process that can be affected by numerous parameters, such as the dataset, the model architecture or even the batch size. In this work, we propose an algorithm for automatically adjusting the learning rate during the training process, assuming a gradient descent formulation. The rationale behind our approach is to train the learning rate along with the model weights. Specifically, we formulate first and second-order gradients w.r.t. the learning rate as functions of consecutive weight gradients, leading to a cost-effective implementation. Our extensive experimental evaluation validates the effectiveness of the proposed method for a plethora of different settings. The proposed method has proven to be robust to both the initial learning rate and the batch size, making it ideal for an off-the-shelf optimizing scheme.
更多查看译文
关键词
gradient descent,adaptive learning rate
AI 理解论文
溯源树
样例
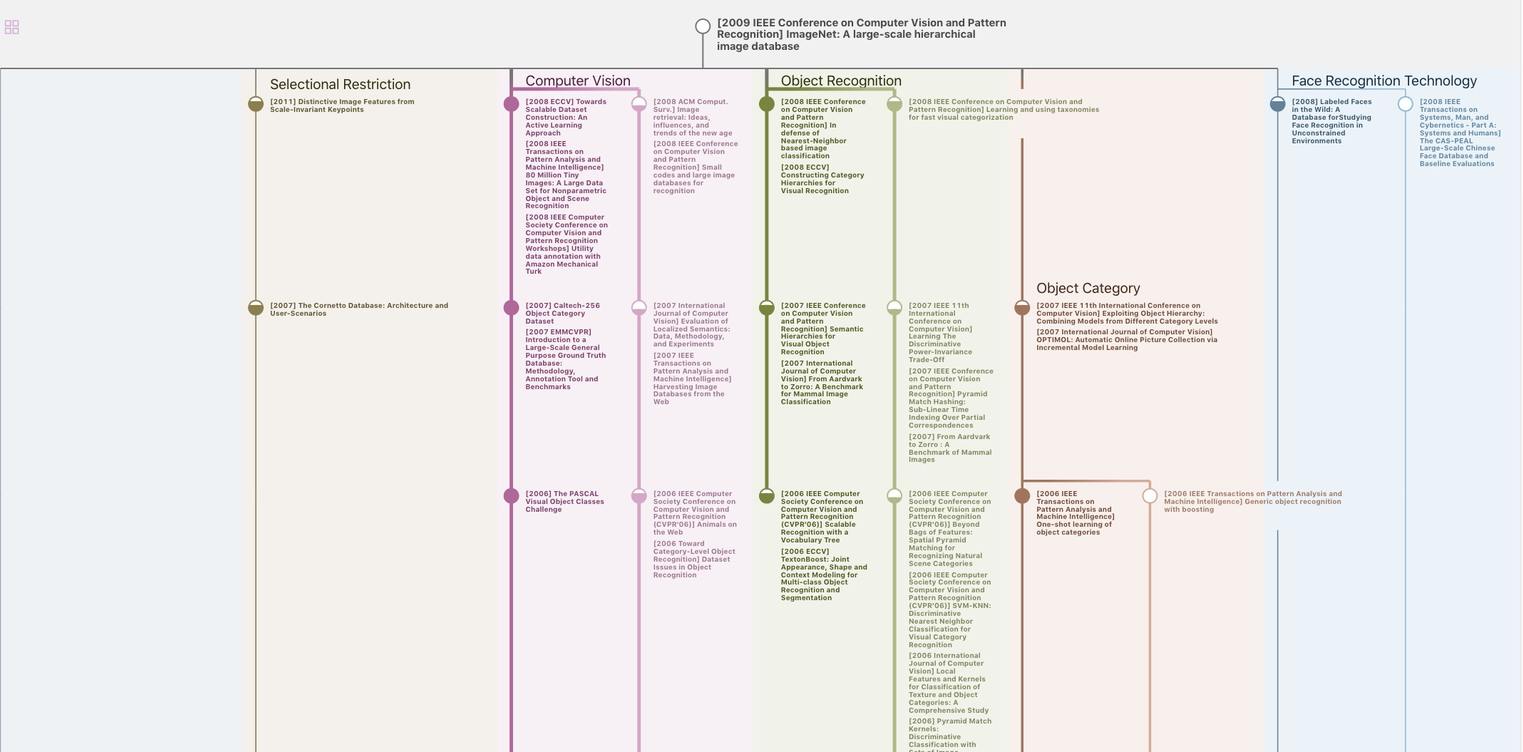
生成溯源树,研究论文发展脉络
Chat Paper
正在生成论文摘要