Dual-graph co-representation learning for knowledge-Graph Enhanced Recommendation
ICASSP 2023 - 2023 IEEE International Conference on Acoustics, Speech and Signal Processing (ICASSP)(2023)
摘要
Knowledge graphs can help improve the performance of recommender systems by mitigating sparsity and cold-start problems. However, existing approaches usually suffer from problems of domain distribution matching and cycle consistency for co-representation learning, as the representations of items from the recommendation domain and entities from the knowledge graph domain are heterogeneous and cannot consistently or effectively transfer information between domains. Moreover, previous works simply propagate in one of user-item graph and knowledge graph, and ignore the topology information of the other graph. We design a dual-graph framework, named DGCR, with two graph neural networks propagating in user-item graph and knowledge graph respectively to extract topology information from both graphs. We also propose Cycle Transfer Unit, shared in every layer as the key to DGCR to model the universal rule of domain transfer. Cycle Transfer Unit can further alleviate the problems of bias between domains and information loss during transfer.
更多查看译文
关键词
Recommender Systems,Knowledge-Graph-Enhanced Recommendation,GNN
AI 理解论文
溯源树
样例
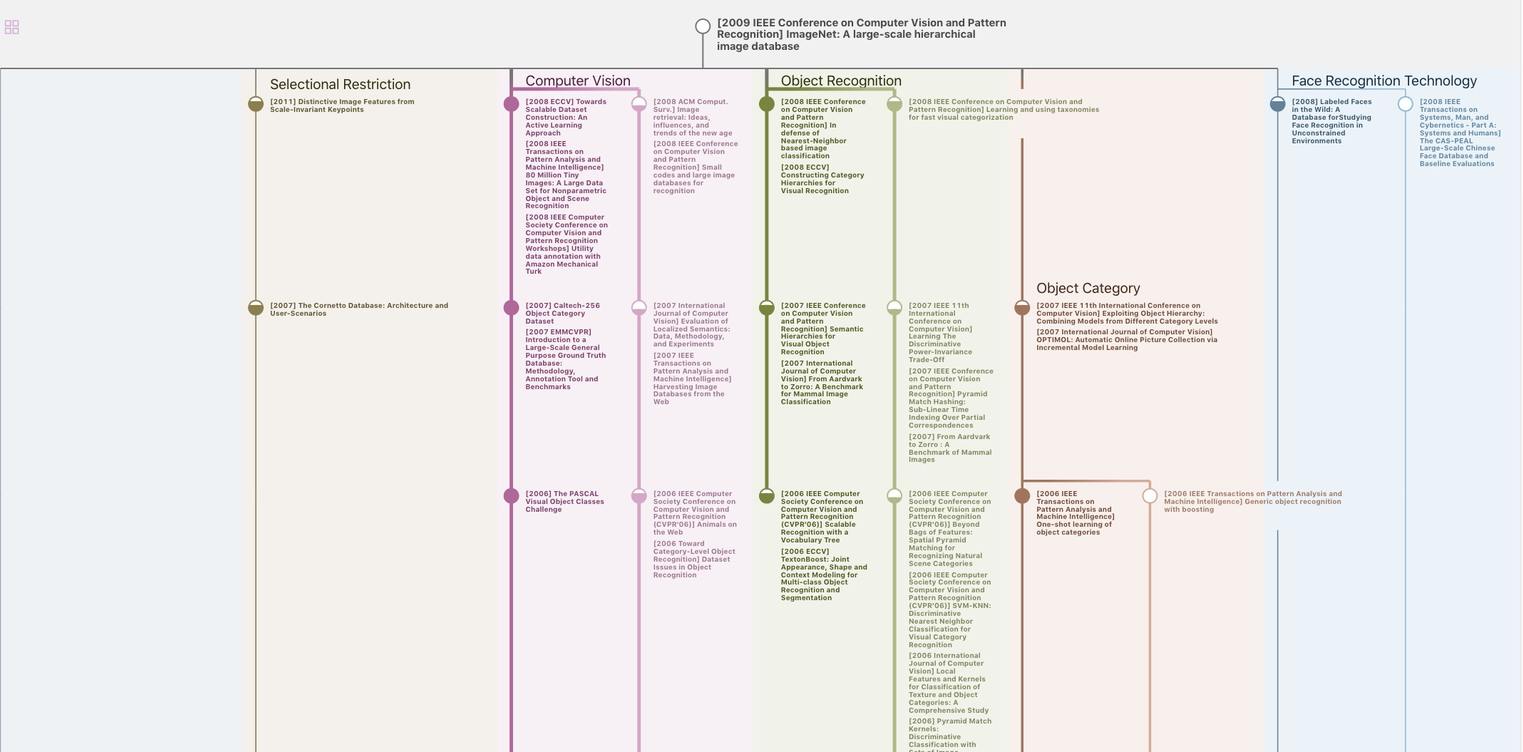
生成溯源树,研究论文发展脉络
Chat Paper
正在生成论文摘要