Estimating Normalized Graph Laplacians in Financial Markets
ICASSP 2023 - 2023 IEEE International Conference on Acoustics, Speech and Signal Processing (ICASSP)(2023)
摘要
Gaussian Markov random fields, a class of graphical models, play an increasingly important role in real-world problems, where they are often applied to uncover conditional correlations between pairs of entities in a network. Motivated by recent applications of graphs in financial markets, we investigate the problem of learning undirected, weighted, normalized, graphical models. More precisely, we design an optimization algorithm to learn precision matrices that are modeled as normalized graph Laplacians. The proposed algorithm takes advantages of frameworks such as the alternating direction method of multipliers and projected gradient descent, which allows us to decompose the original problem into subproblems that can be solved efficiently. We demonstrate the empirical performance of the proposed algorithm, in comparison to state-of-the-art benchmark models, in a number of datasets involving financial time-series.
更多查看译文
关键词
graphical models,normalized Laplacian,financial markets,time series,estimation theory
AI 理解论文
溯源树
样例
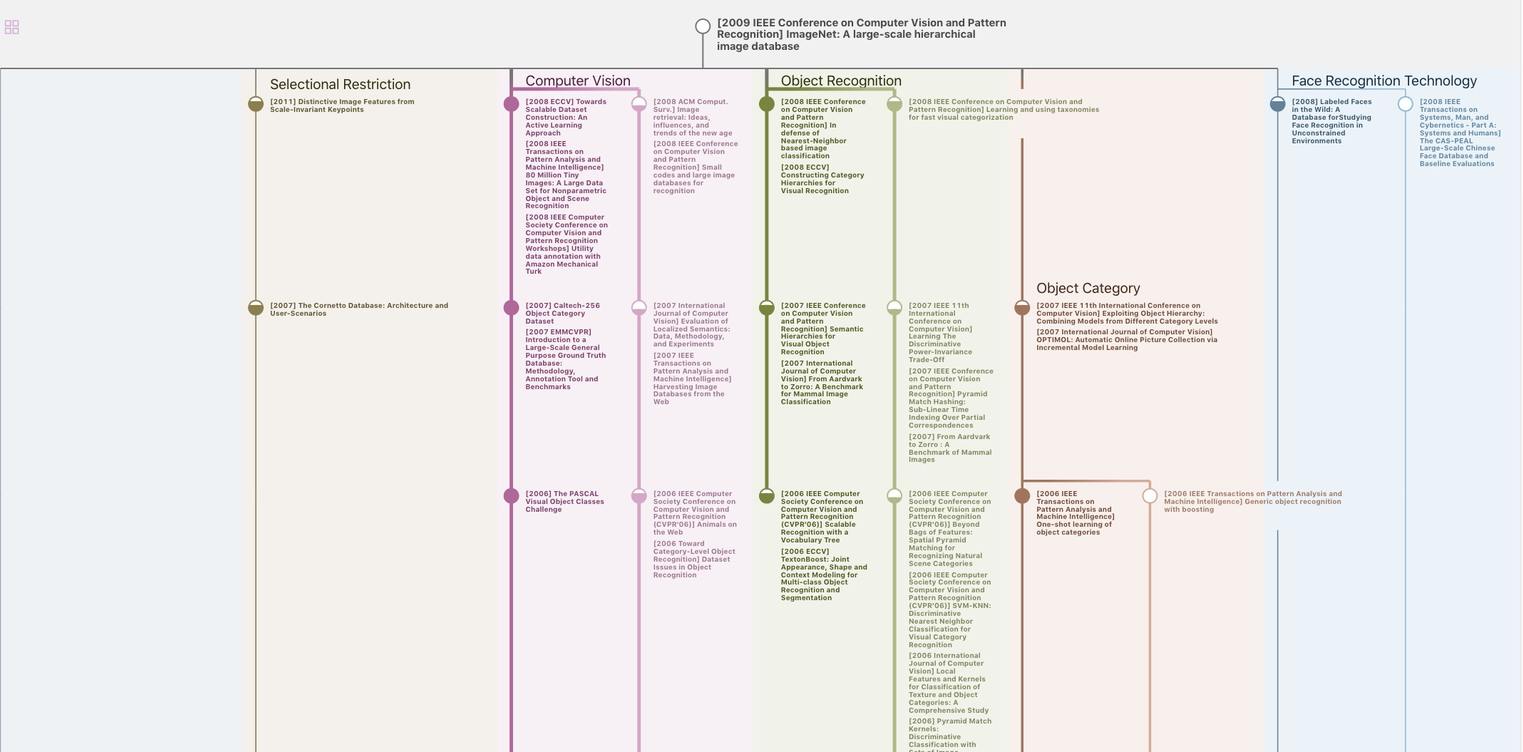
生成溯源树,研究论文发展脉络
Chat Paper
正在生成论文摘要